Finding Representative Sampling Subsets in Sensor Graphs Using Time-series Similarities
arxiv(2023)
摘要
With the increasing use of Internet-of-Things-enabled sensors, it is important to have effective methods to query the sensors. For example, in a dense network of battery-driven temperature sensors, it is often possible to query (sample) only a subset of the sensors at any given time, since the values of the non-sampled sensors can be estimated from the sampled values. If we can divide the set of sensors into disjoint so-called representative sampling subsets, in which each represents all the other sensors sufficiently well, then we can alternate between the sampling subsets and, thus, increase the battery life significantly of the sensor network. In this article, we formulate the problem of finding representative sampling subsets as a graph problem on a so-called sensor graph with the sensors as nodes. Our proposed solution, SubGraphSample, consists of two phases. In Phase-I, we create edges in the similarity graph based on the similarities between the time-series of sensor values, analyzing six different techniques based on proven time-series similarity metrics. In Phase-II, we propose six different sampling techniques to find the maximum number of representative sampling subsets. Finally, we propose AutoSubGraphSample, which auto-selects the best technique for Phase-I and Phase-II for a given dataset. Our extensive experimental evaluation shows that AutoSubGraphSample can yield significant battery-life improvements within realistic error bounds.
更多查看译文
关键词
Sampling sets,similarity graph,reconstruction error,stratification approach,time-series similarity,internet of things
AI 理解论文
溯源树
样例
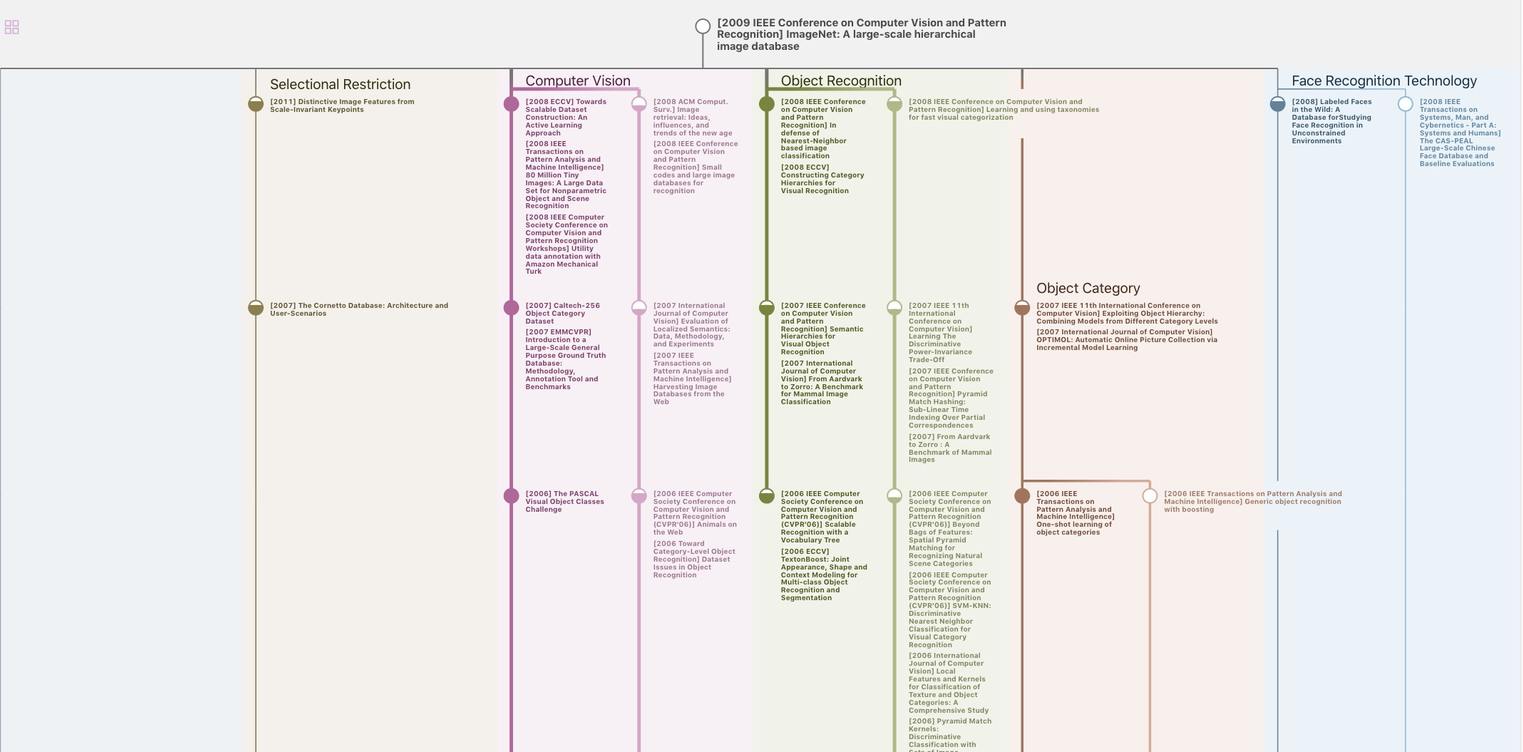
生成溯源树,研究论文发展脉络
Chat Paper
正在生成论文摘要