Homogeneous Transfer Active Learning for Time Series Classification.
ICMLA(2021)
摘要
The scarcity of labeled time-series data is a major challenge in use of deep learning methods for Time Series Classification tasks. This is especially important for the growing field of sensors and Internet of things, where data of high dimensions and complex distributions coming from the numerous field devices has to be analyzed to provide meaningful applications. To address the problem of scarce training data, we propose a heuristic combination of deep transfer learning and deep active learning methods to provide near optimal training abilities to the classification model. To mitigate the need of labeling large training set, two essential criteria - informativeness and representativeness have been proposed for selecting time series training samples. After training the model on source dataset, we propose a framework for the model skill transfer to forecast certain weather variables on a target dataset in an homogeneous transfer settings. Extensive experiments on three weather datasets show that the proposed hybrid Transfer Active Learning method achieves a higher classification accuracy than existing methods, while using only 20% of the training samples.
更多查看译文
关键词
Transfer Learning,Active Learning,Time Series Classification
AI 理解论文
溯源树
样例
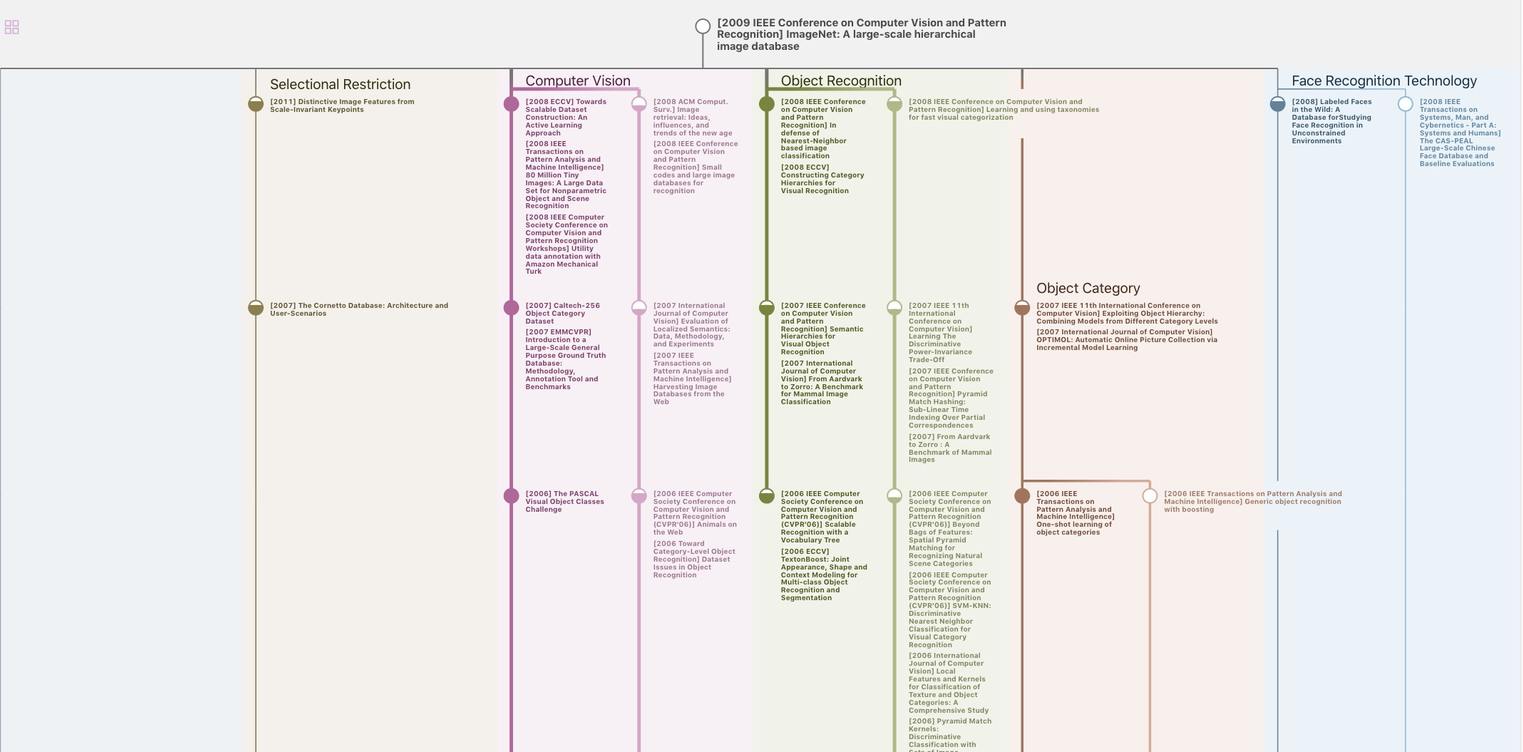
生成溯源树,研究论文发展脉络
Chat Paper
正在生成论文摘要