Federated Principal Component Analysis for Genome-Wide Association Studies
2021 21ST IEEE INTERNATIONAL CONFERENCE ON DATA MINING (ICDM 2021)(2021)
摘要
Federated learning (FL) has emerged as a privacy-aware alternative to centralized data analysis, especially for biomedical analyses such as genome-wide association studies (GWAS). The data remains with the owner, which enables studies previously impossible due to privacy protection regulations. Principal component analysis (PCA) is a frequent preprocessing step in GWAS, where the eigenvectors of the sample-by-sample covariance matrix are used as covariates in the statistical tests. Therefore, a federated version of PCA suitable for vertical data partitioning is required for federated GWAS. Existing federated PCA algorithms exchange the complete sample eigenvectors, a potential privacy breach. In this paper, we present a federated PCA algorithm for vertically partitioned data which does not exchange the sample eigenvectors and is hence suitable for federated GWAS. We show that it outperforms existing federated solutions in terms of convergence behavior and scalability. Additionally, we provide a user-friendly privacy-aware web tool to promote acceptance of federated PCA among GWAS researchers.
更多查看译文
关键词
Federated Learning,Principal Component Analysis,PCA,Unsupervised Machine Learning,GWAS,Genome wide Association Studies,Population Stratification,Vertical Data Partitioning
AI 理解论文
溯源树
样例
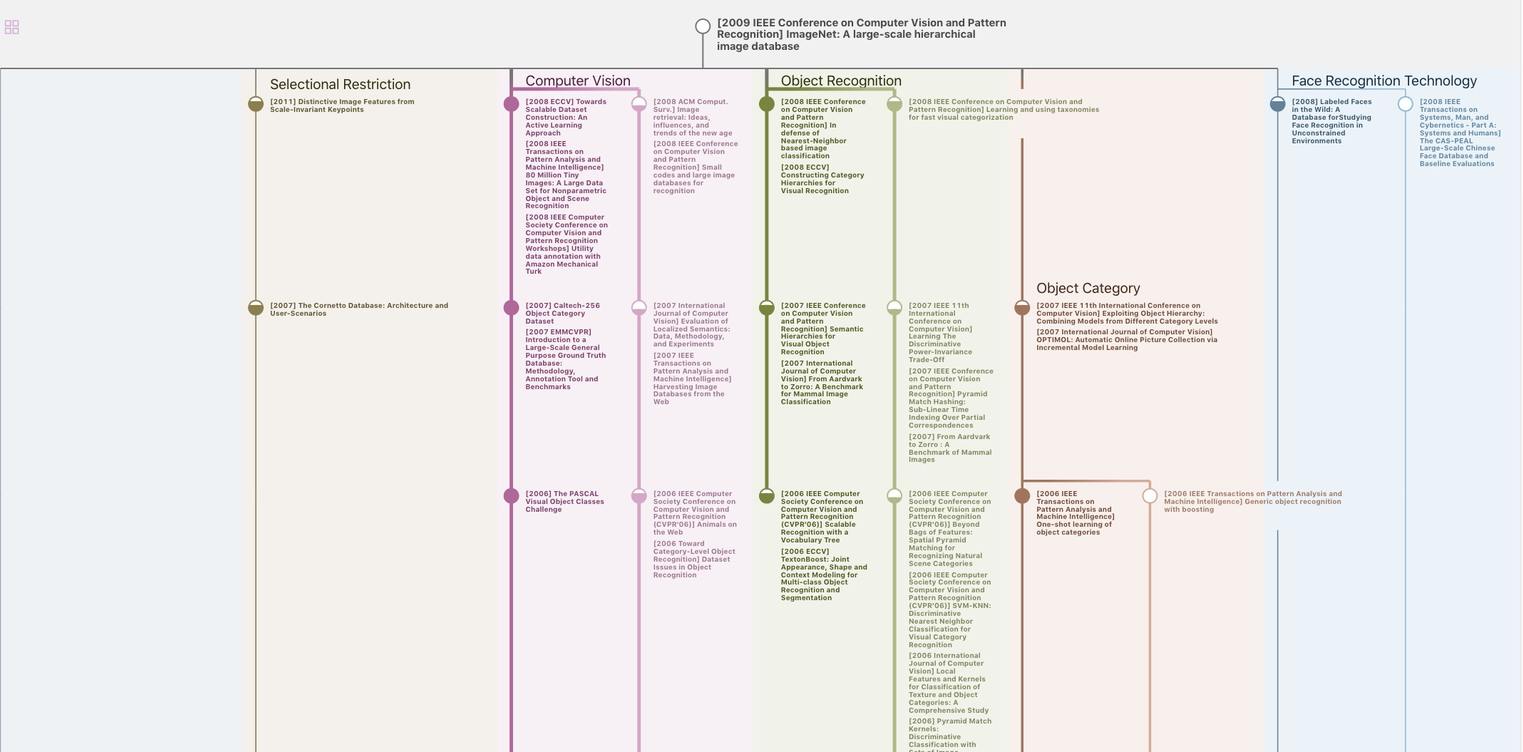
生成溯源树,研究论文发展脉络
Chat Paper
正在生成论文摘要