Cam-Guided U-Net With Adversarial Regularization For Defect Segmentation.
ICIP(2021)
摘要
Defect segmentation is critical in real-wold industrial product quality assessment. There are usually a huge number of normal (defect-free) images but a very limited number of annotated anomalous images. This poses huge challenges to exploiting Fully-Convolutional Networks (FCN), e.g., UNet, as they require sufficient anomalous images with defect annotations during training. To further leverage the information from normal data, a novel CAM-guided U-Net with adversarial regularization (CAM-UNet-AR) is proposed. We first modify the existing CAM-UNet to incorporate the CAMs for both normal and anomalous classes and fine-tune the segmentation network using a combined loss which jointly considers pixel-wise classification, foreground segmentation and boundary segmentation. Secondly, an auxiliary adversarial regularization module (ARM) is proposed to facilitate the segmentation network to encode the "normal components" from training images into consistent representations. Extensive experiments on MVTec AD dataset show the superiority of our proposed network over multiple state-of-the-art U-Net variants.
更多查看译文
关键词
Defect Segmentation,U-Net,Adversarial Regularization
AI 理解论文
溯源树
样例
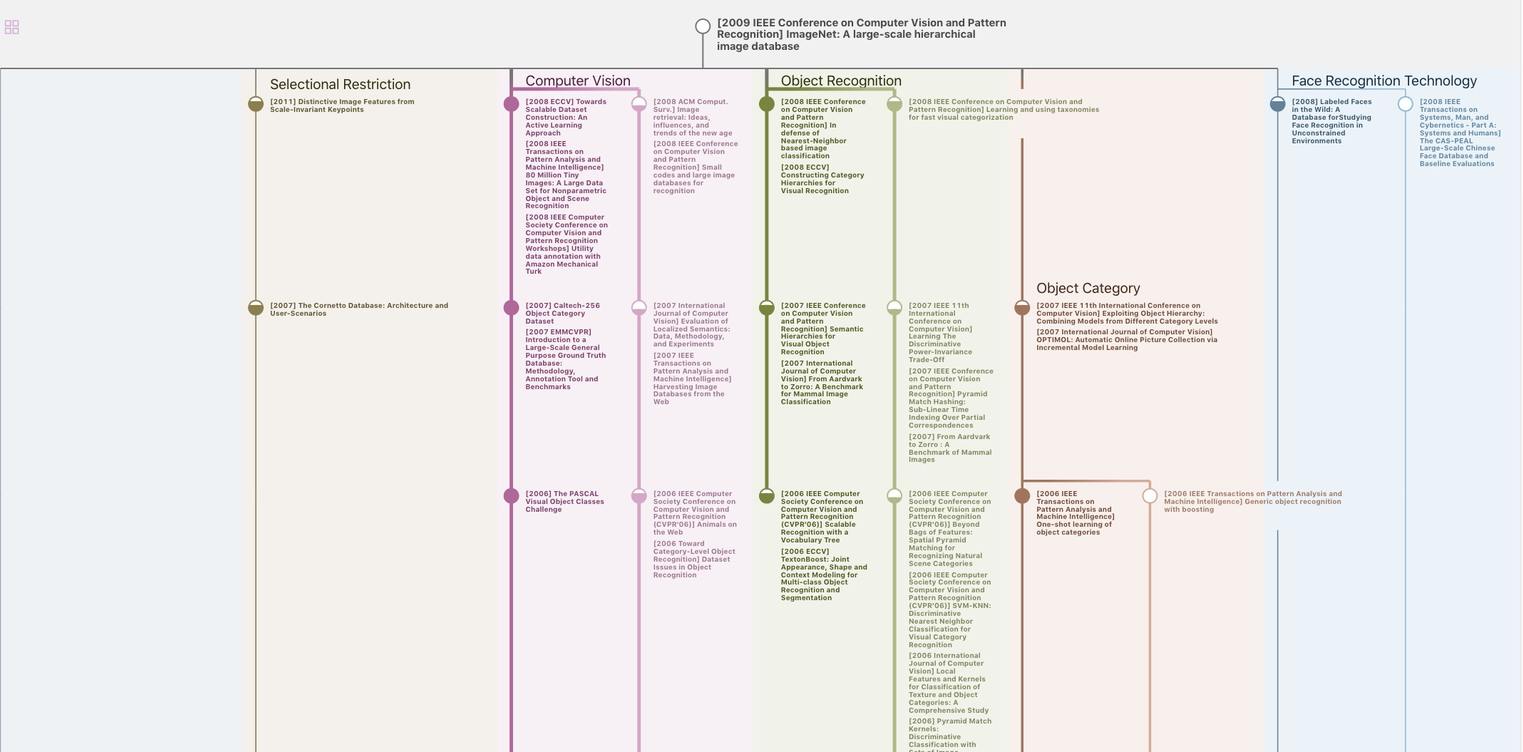
生成溯源树,研究论文发展脉络
Chat Paper
正在生成论文摘要