Improving Neural Blind Deconvolution.
ICIP(2021)
摘要
The field of blind image deblurring was for a long time dominated by Maximum-A-Posteriori methods seeking the optimal pair of sharp image-blur of a suitable functional. Recently, learning-based methods, especially those based on deep convolutional neural networks, are proving effective and are receiving increasing attention by the research community. In 2020, Ren et al. proposed a deblurring method called SelfDeblur which combines the model-driven approach of traditional MAP methods and the generative power of neural nets. The method is capable of producing very high-quality results, yet it inherits some problems of MAP methods, especially possible convergence to a wrong local optimum. In this paper we propose several easy-to-implement modifications of SelfDeblur, namely suitable initialization, multiscale processing, and regularization, that improve the average performance of the original method and decrease the probability of failure.
更多查看译文
关键词
blind deblurring,SelfDeblur,deep image prior,multiscale
AI 理解论文
溯源树
样例
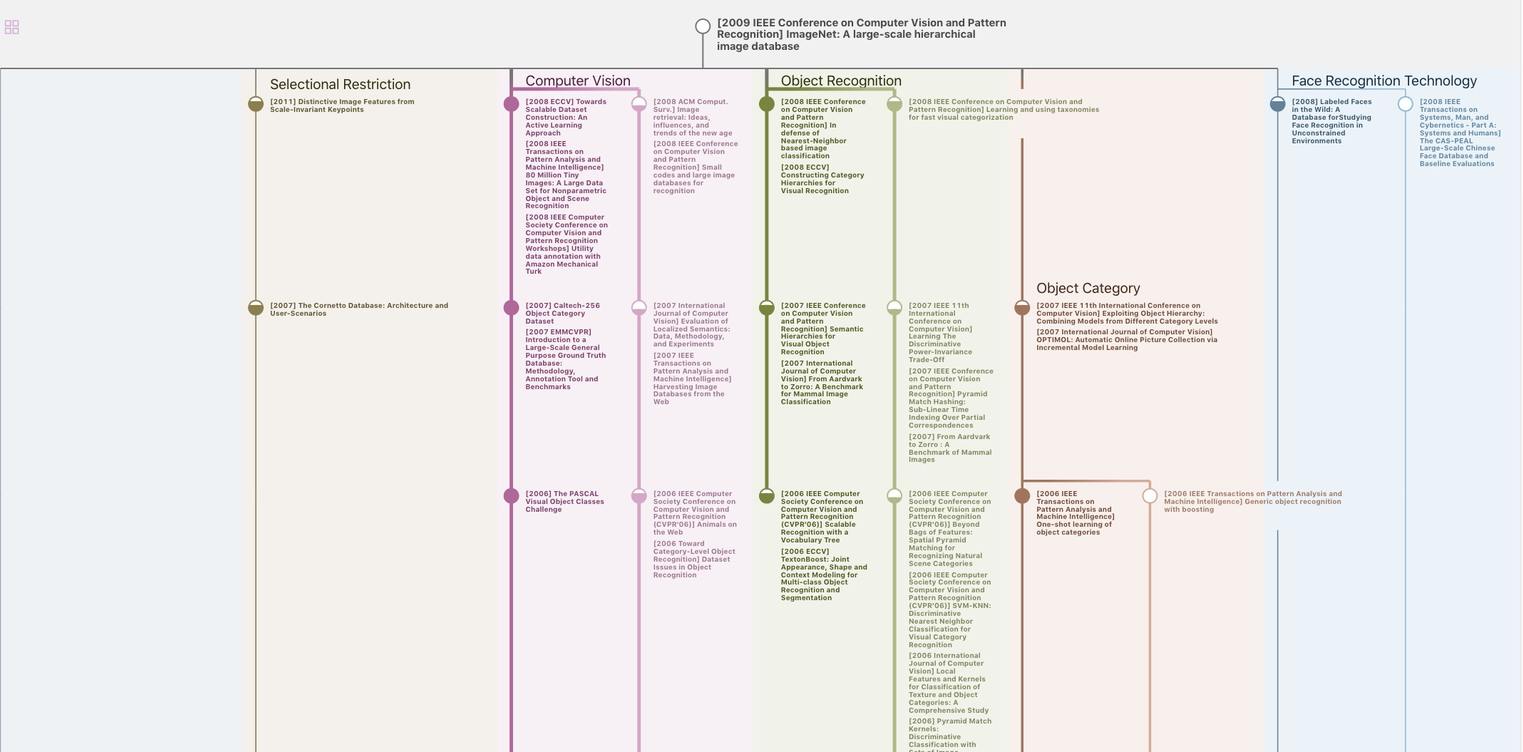
生成溯源树,研究论文发展脉络
Chat Paper
正在生成论文摘要