Multivariate LSTM for Stock Market Volatility Prediction
MACHINE LEARNING, OPTIMIZATION, AND DATA SCIENCE (LOD 2021), PT II(2022)
摘要
Volatility is a measure of fluctuation in financial asset returns, practical measurement of risk, and a key variable for calculating options prices. Accurate prediction of volatility is crucial to maintaining profitable investments and trading strategies. Statistical models such as GARCH are used today to predict volatility and time series, though new methods are actively being researched to improve the prediction accuracy to cope with the rapidly increasing trading volumes and stock market influencing factors. The aim of this paper is to investigate a new method to improve market volatility forecasting accuracy by innovatively introducing a new setup of the Recurrent Neural Network (RNN) algorithm. In particular, the proposed model is a stacked Long Short-Term Memory (LSTM) with multivariate input composed of multiple asset daily prices of different lag time-steps. The proposed model is used to predict volatility under different market conditions and is compared to the predictions obtained with GARCH as well as to the actual volatility of the same forecasting period. The results show that the prediction of the future realized volatility using a single feature LSTM has comparable accuracy to GARCH. They also indicate that a stacked LSTM can significantly improve the volatility prediction accuracy when configured with multivariate input of more than one asset and a lagging period of more than a day. A stacked multivariate LSTM setup enables the prediction model to capture complex patterns in the time series data of assets prices and provides a superior alternative to statistical models in volatility modelling and prediction. The proposed multivariate LSTM architecture clearly shows faster and more accurate modelling of daily volatility and therefore can be used for intra-day modelling specifically for high frequency trading environments.
更多查看译文
关键词
Deep Learning, Recurrent Neural Network, Long Short-Term Memory, Financial markets, Volatility, Day trading
AI 理解论文
溯源树
样例
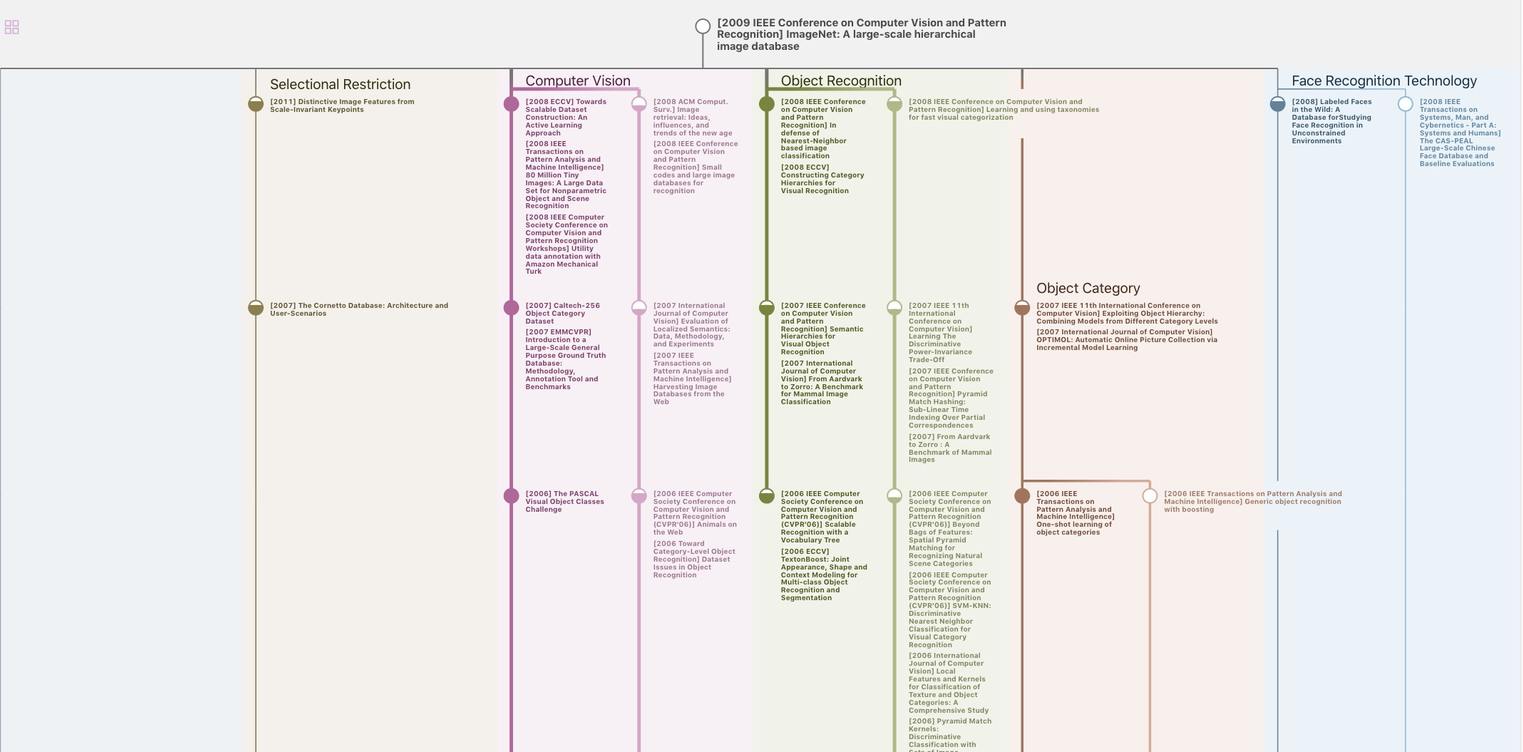
生成溯源树,研究论文发展脉络
Chat Paper
正在生成论文摘要