Efficient Online Label Consistent Hashing for Large-Scale Cross-Modal Retrieval.
ICME(2021)
摘要
Existing cross-modal hashing still faces three challenges: (1) Most batch-based methods are unsuitable for processing large-scale and streaming data. (2) Current online methods often suffer from insufficient semantic association, while lacking flexibility to learn the hash functions for varying streaming data. (3) Existing supervised methods always require much computation time or accumulate large quantization loss to learn hash codes. To address above challenges, we present an efficient Online Label Consistent Hashing (OLCH) for cross-modal retrieval, which aims to incrementally learn hash codes for the current arriving data, while updating the hash functions at a streaming manner. To be specific, an on-line semantic representation learning framework is designed to adaptively preserve the semantic similarity across different modalities, and a mini-batch online gradient descent approach associated with forward-backward splitting is developed to optimize the hash functions. Accordingly, the hash codes are adaptively learned online with the high discriminative capability, while avoiding high computation complexity to process the streaming data. Experimental results show its outstanding performance in comparison with the-state-of-arts.
更多查看译文
关键词
Online label consistent hashing,online semantic representation,mini-batch online gradient descent,forward-backward splitting
AI 理解论文
溯源树
样例
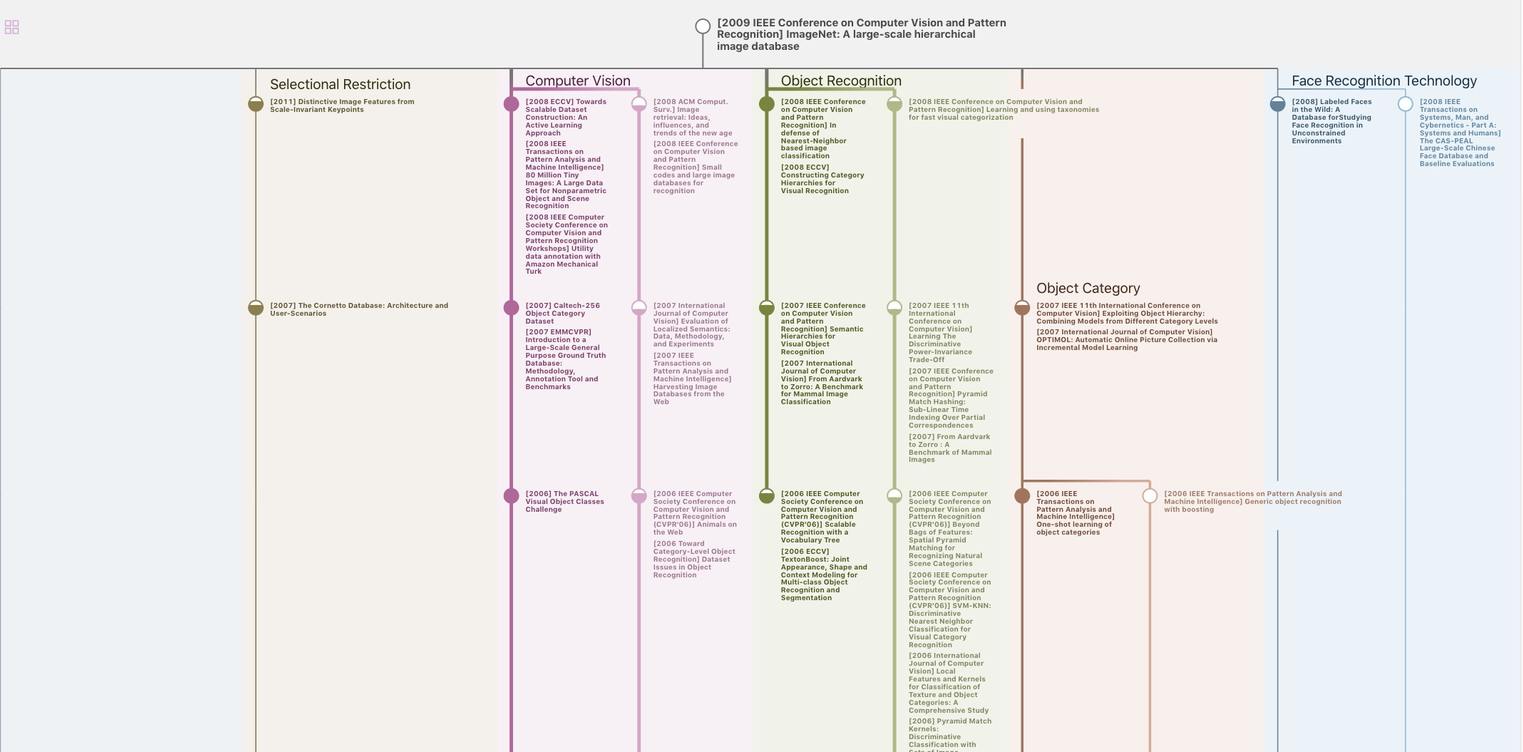
生成溯源树,研究论文发展脉络
Chat Paper
正在生成论文摘要