Similarity learning to enable building searches in post-event image data
COMPUTER-AIDED CIVIL AND INFRASTRUCTURE ENGINEERING(2022)
摘要
Reconnaissance teams collect perishable data after each disaster to learn about building performance. However, often these large image sets are not adequately curated, nor do they have sufficient metadata (e.g., GPS), hindering any chance to identify images from the same building when collected by different reconnaissance teams. In this study, Siamese convolutional neural networks (S-CNN) are implemented and repurposed to establish a building search capability suitable for post-disaster imagery. This method can automatically rank and retrieve corresponding building images in response to a single query using an image. In the demonstration, we utilize real-world images collected from 174 reinforced-concrete buildings affected by the 2016 Southern Taiwan and the 2017 Pohang (South Korea) earthquake events. A quantitative performance evaluation is conducted by examining two metrics introduced for this application: Similarity Score (SS) and Similarity Rank (SR).
更多查看译文
AI 理解论文
溯源树
样例
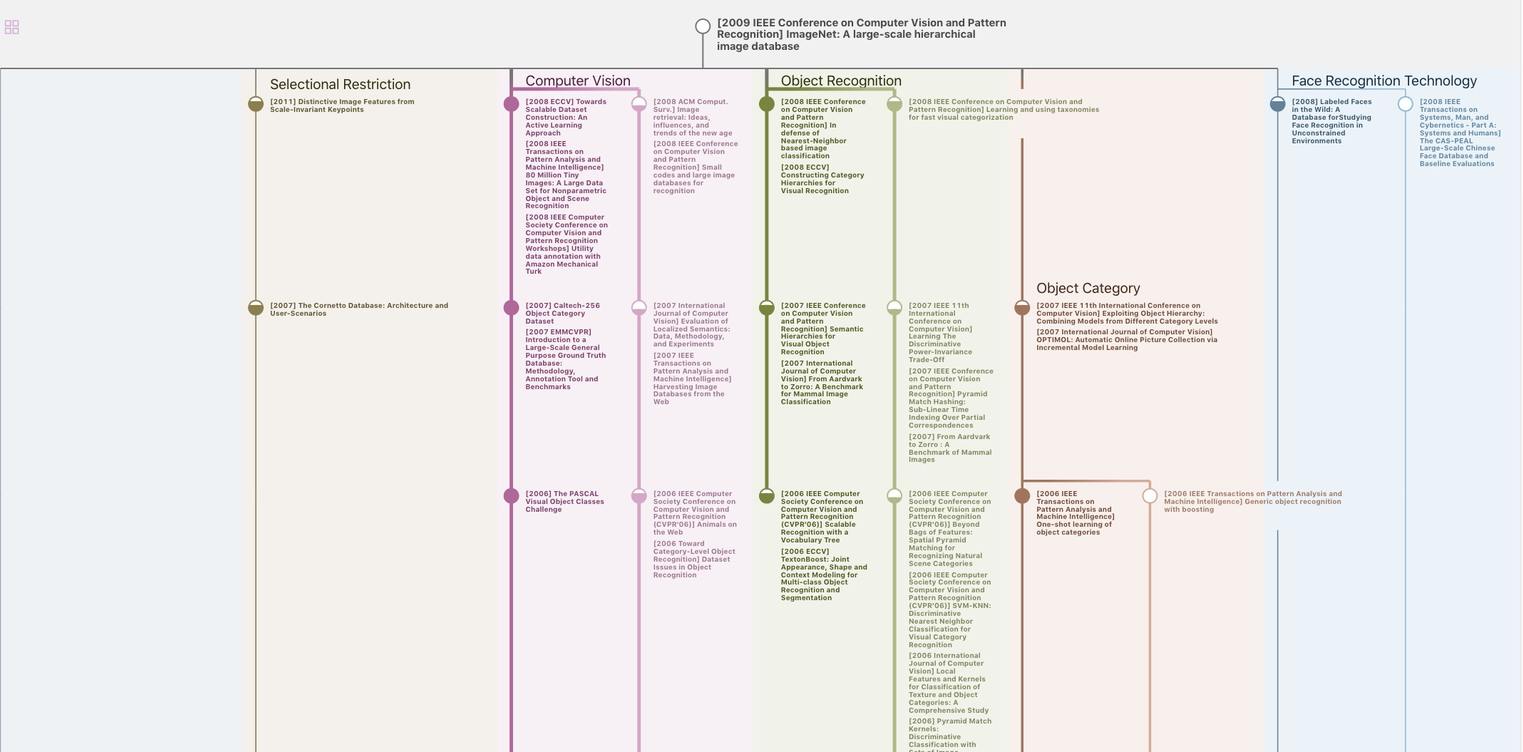
生成溯源树,研究论文发展脉络
Chat Paper
正在生成论文摘要