A collaborative decision support system for multi-criteria automatic clustering
DECISION SUPPORT SYSTEMS(2022)
摘要
Automatic clustering is a challenging problem, especially when the decision-maker has little or no information about the nature of the dataset and the criteria of interest. There is a lack of generalizability in the current validity indexes (VI) for automatic clustering algorithms, as each considers a limited number of objectives and mostly ignores the other aspects of clustering validation. The proposed framework benefits from collaboration among selected evolutionary algorithms. A mixed-integer non-linear programming model is developed, and a framework is proposed for a six-step decision support system to solve it. The decision-maker (DM) selects the quantitative (primary) VIs and the evolutionary algorithms. Given DM's knowledge on the dataset and VIs, DM can incorporate qualitative (secondary) VIs. DM determines the quality threshold for each VI and runs the evolutionary algorithms separately. The DSS then saves the best obtained value of VIs in order to prepare the input necessary to construct the aggregated function. Based on the selected primary VIs, a new normalized aggregated function is developed and solved repeatedly using the randomly selected or predefined weights of importance. Eventually, DM employs a proper DEA model to define the final clustering output among all possible solutions. Given multiple efficient solutions, the best-worst method and a multi-criteria decision-making approach are applied to find the final output. The applicability of the proposed approach is illustrated on a synthetic and two secondary datasets, and the result at each step is discussed in detail.
更多查看译文
关键词
Automatic clustering, Evolutionary algorithms, Multi-objective, DEA, BWM
AI 理解论文
溯源树
样例
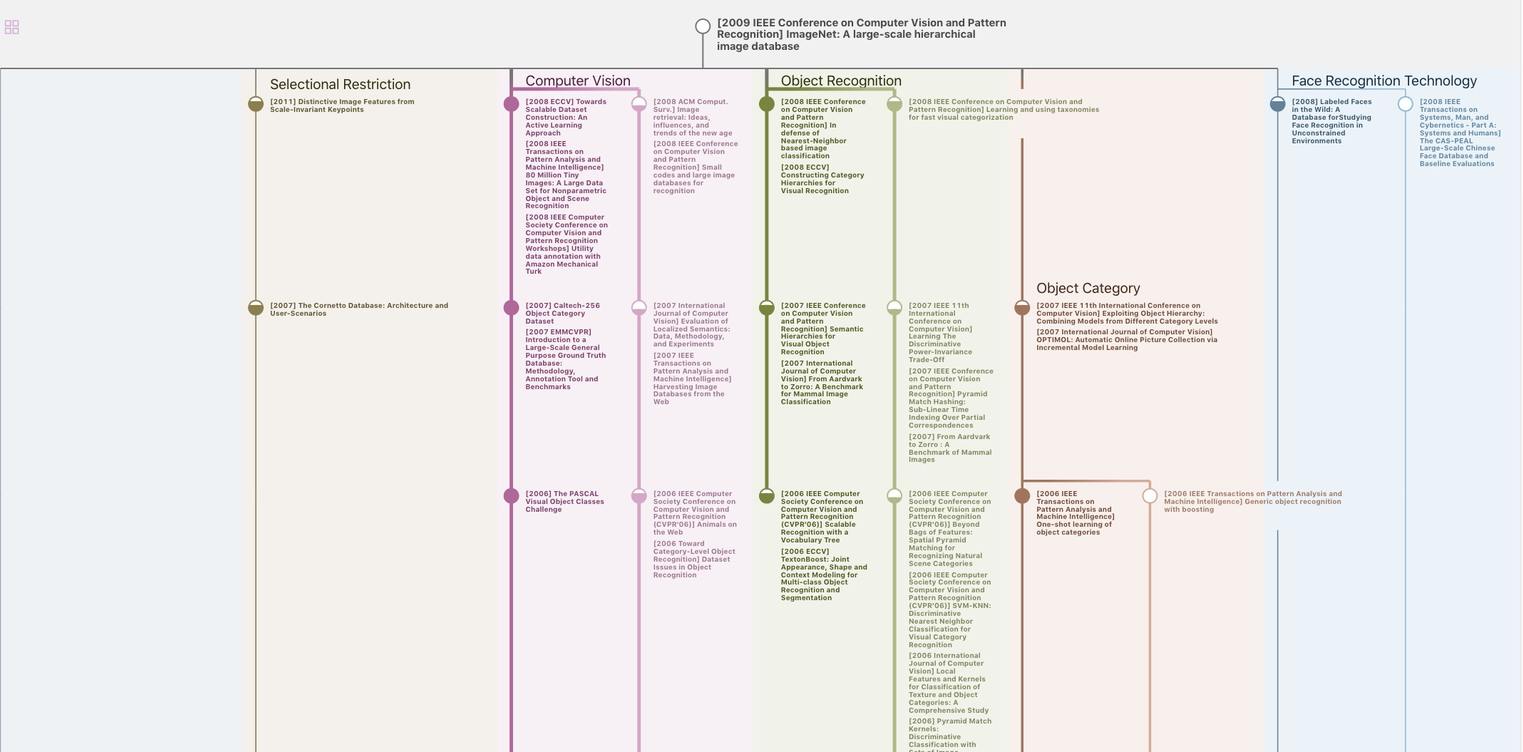
生成溯源树,研究论文发展脉络
Chat Paper
正在生成论文摘要