Towards AutoQML: A Cloud-Based Automated Circuit Architecture Search Framework
2022 IEEE 19th International Conference on Software Architecture Companion (ICSA-C)(2022)
摘要
The learning process of classical machine learning algorithms is tuned by hyperparameters that need to be customized to best learn and generalize from an input dataset. In recent years, Quantum Machine Learning (QML) has been gaining traction as a possible application of quantum computing which may provide quantum advantage in the future. However, quantum versions of classical machine learning algorithms introduce a plethora of additional parameters and circuit variations that have their own intricacies in being tuned.In this work, we take the first steps towards Automated Quantum Machine Learning (AutoQML). We propose a concrete description of the problem, and then develop a classical-quantum hybrid cloud architecture that allows for parallelized hyperparameter exploration and model training.As an application use-case, we train a quantum Generative Adversarial neural Network (qGAN) to generate energy prices that follow a known historic data distribution. Such a QML model can be used for various applications in the energy economics sector.
更多查看译文
关键词
quantum machine learning,parametrized quantum circuit,quantum neural network,software architecture,cloud computing
AI 理解论文
溯源树
样例
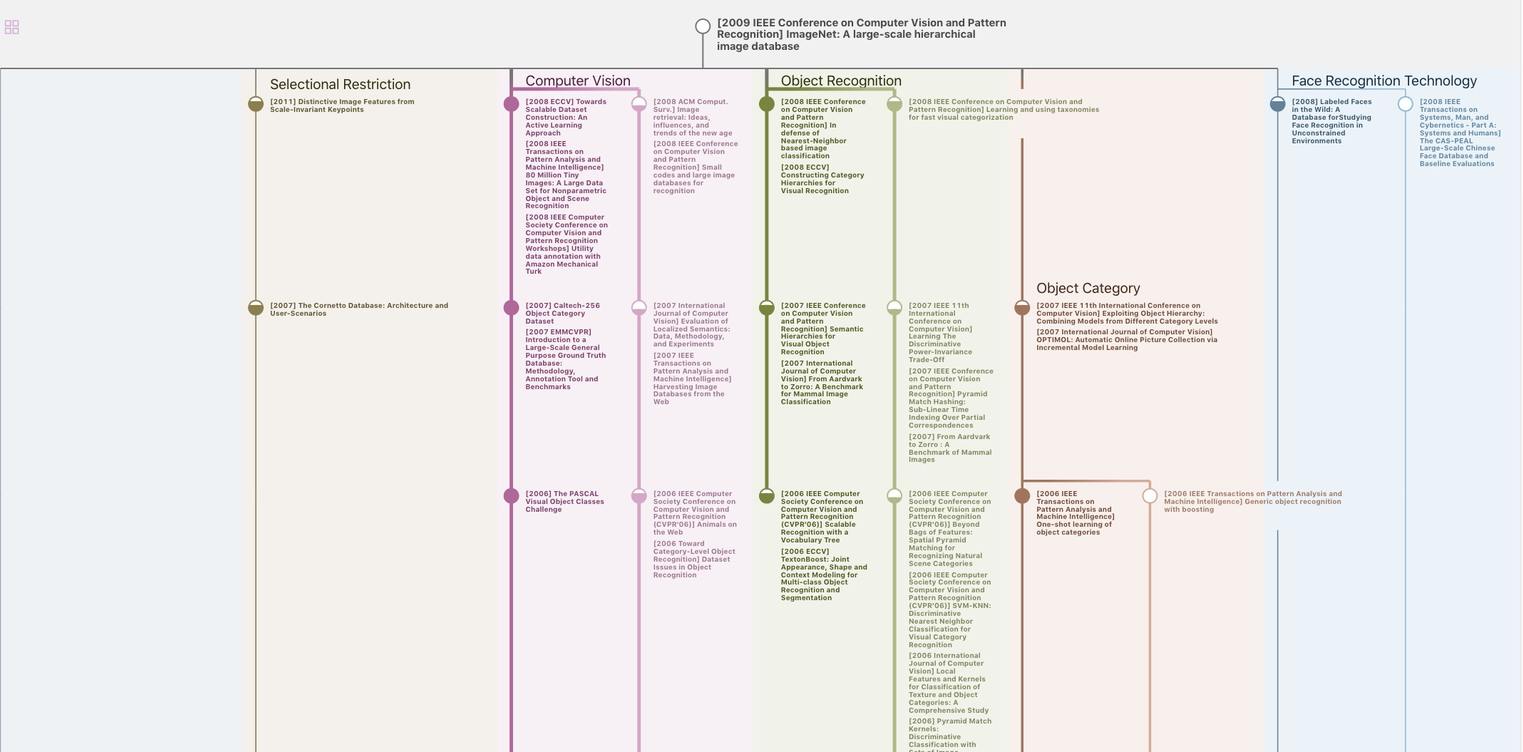
生成溯源树,研究论文发展脉络
Chat Paper
正在生成论文摘要