Causal Structural Learning on MPHIA Individual Dataset.
Journal of the American Statistical Association(2022)
摘要
The Population-based HIV Impact Assessment (PHIA) is an ongoing project that conducts nationally representative HIV-focused surveys for measuring national and regional progress toward UNAIDS' 90-90-90 targets, the primary strategy to end the HIV epidemic. We believe the PHIA survey offers a unique opportunity to better understand the key factors that drive the HIV epidemics in the most affected countries in sub-Saharan Africa. In this article, we propose a novel causal structural learning algorithm to discover important covariates and potential causal pathways for 90-90-90 targets. Existing constrained-based causal structural learning algorithms are quite aggressive in edge removal. The proposed algorithm preserves more information about important features and potential causal pathways. It is applied to the Malawi PHIA (MPHIA) data set and leads to interesting results. For example, it discovers age and condom usage to be important for female HIV awareness; the number of sexual partners to be important for male HIV awareness; and knowing the travel time to HIV care facilities leads to a higher chance of being treated for both females and males. We further compare and validate the proposed algorithm using BIC and using Monte Carlo simulations, and show that the proposed algorithm achieves improvement in true positive rates in important feature discovery over existing algorithms.
更多查看译文
关键词
90-90-90 targets,Causal structural learning,HIV,PHIA
AI 理解论文
溯源树
样例
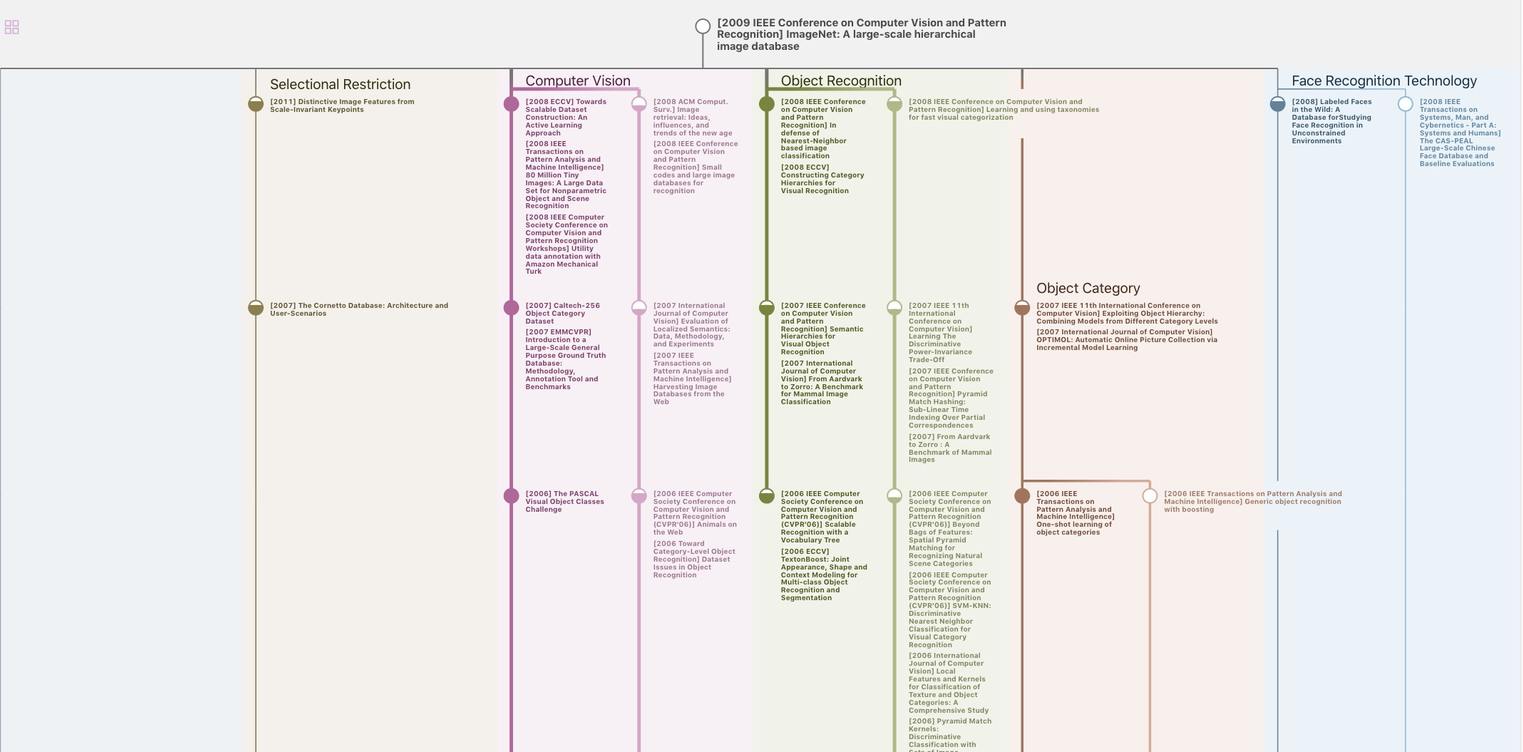
生成溯源树,研究论文发展脉络
Chat Paper
正在生成论文摘要