Reward Reports for Reinforcement Learning
PROCEEDINGS OF THE 2023 AAAI/ACM CONFERENCE ON AI, ETHICS, AND SOCIETY, AIES 2023(2023)
摘要
Building systems that are good for society in the face of complex societal effects requires a dynamic approach. Recent approaches to machine learning (ML) documentation have demonstrated the promise of discursive frameworks for deliberation about these complexities. However, these developments have been grounded in a static ML paradigm, leaving the role of feedback and post-deployment performance unexamined. Meanwhile, recent work in reinforcement learning has shown that the effects of feedback and optimization objectives on system behavior can be wide-ranging and unpredictable. In this paper we sketch a framework for documenting deployed and iteratively updated learning systems, which we call Reward Reports. Taking inspiration from technical concepts in reinforcement learning, we outline Reward Reports as living documents that track updates to design choices and assumptions behind what a particular automated system is optimizing for. They are intended to track dynamic phenomena arising from system deployment, rather than merely static properties of models or data. After presenting the elements of a Reward Report, we discuss a concrete example: Meta's BlenderBot 3 chatbot. Several others for game-playing (DeepMind's MuZero), content recommendation (MovieLens), and traffic control (Project Flow) are included in the appendix.
更多查看译文
关键词
Reward function,reporting,documentation,disaggregated evaluation,ethical considerations
AI 理解论文
溯源树
样例
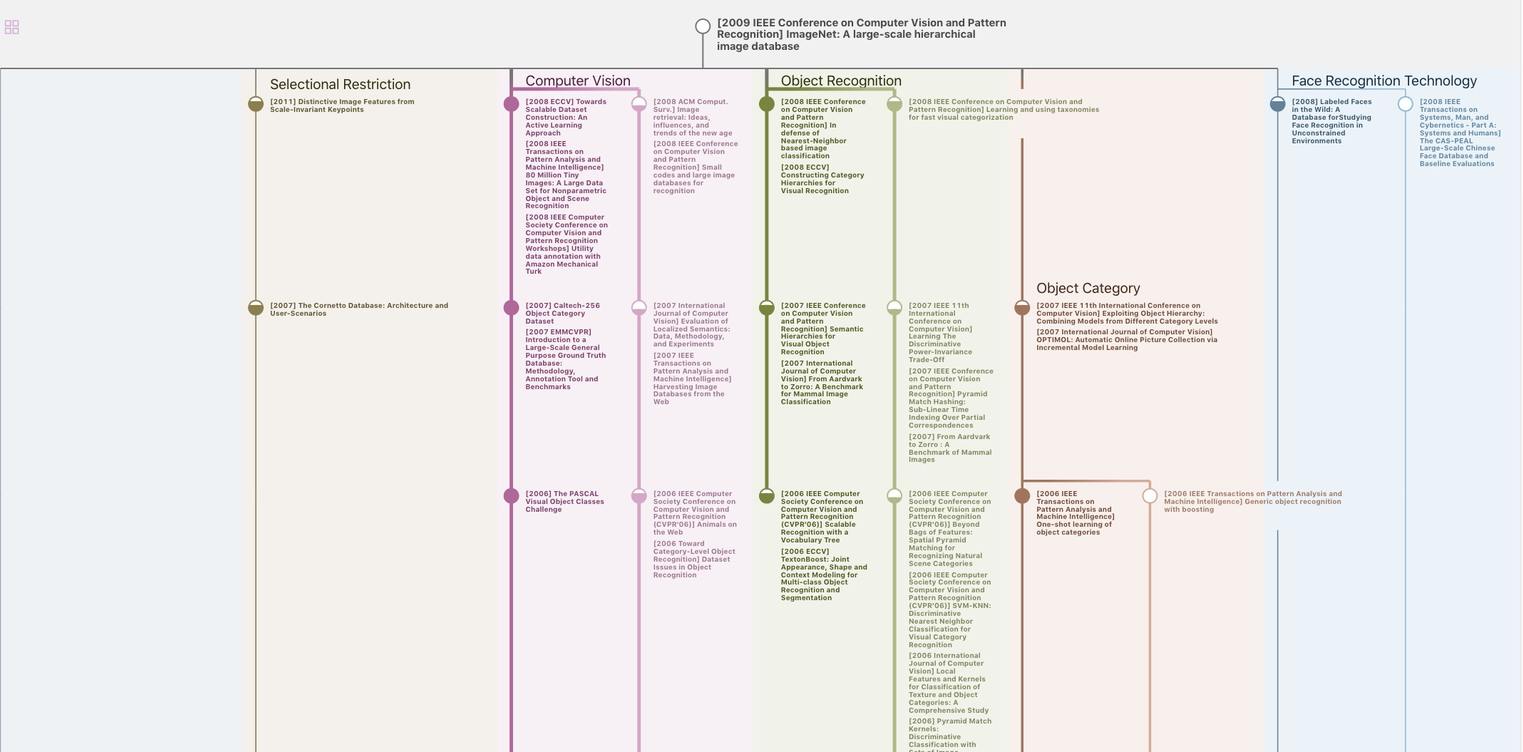
生成溯源树,研究论文发展脉络
Chat Paper
正在生成论文摘要