Network inference with Granger causality ensembles on single-cell transcriptomics.
Cell reports(2022)
摘要
Cellular gene expression changes throughout a dynamic biological process, such as differentiation. Pseudotimes estimate cells' progress along a dynamic process based on their individual gene expression states. Ordering the expression data by pseudotime provides information about the underlying regulator-gene interactions. Because the pseudotime distribution is not uniform, many standard mathematical methods are inapplicable for analyzing the ordered gene expression states. Here we present single-cell inference of networks using Granger ensembles (SINGE), an algorithm for gene regulatory network inference from ordered single-cell gene expression data. SINGE uses kernel-based Granger causality regression to smooth irregular pseudotimes and missing expression values. It aggregates predictions from an ensemble of regression analyses to compile a ranked list of candidate interactions between transcriptional regulators and target genes. In two mouse embryonic stem cell differentiation datasets, SINGE outperforms other contemporary algorithms. However, a more detailed examination reveals caveats about poor performance for individual regulators and uninformative pseudotimes.
更多查看译文
AI 理解论文
溯源树
样例
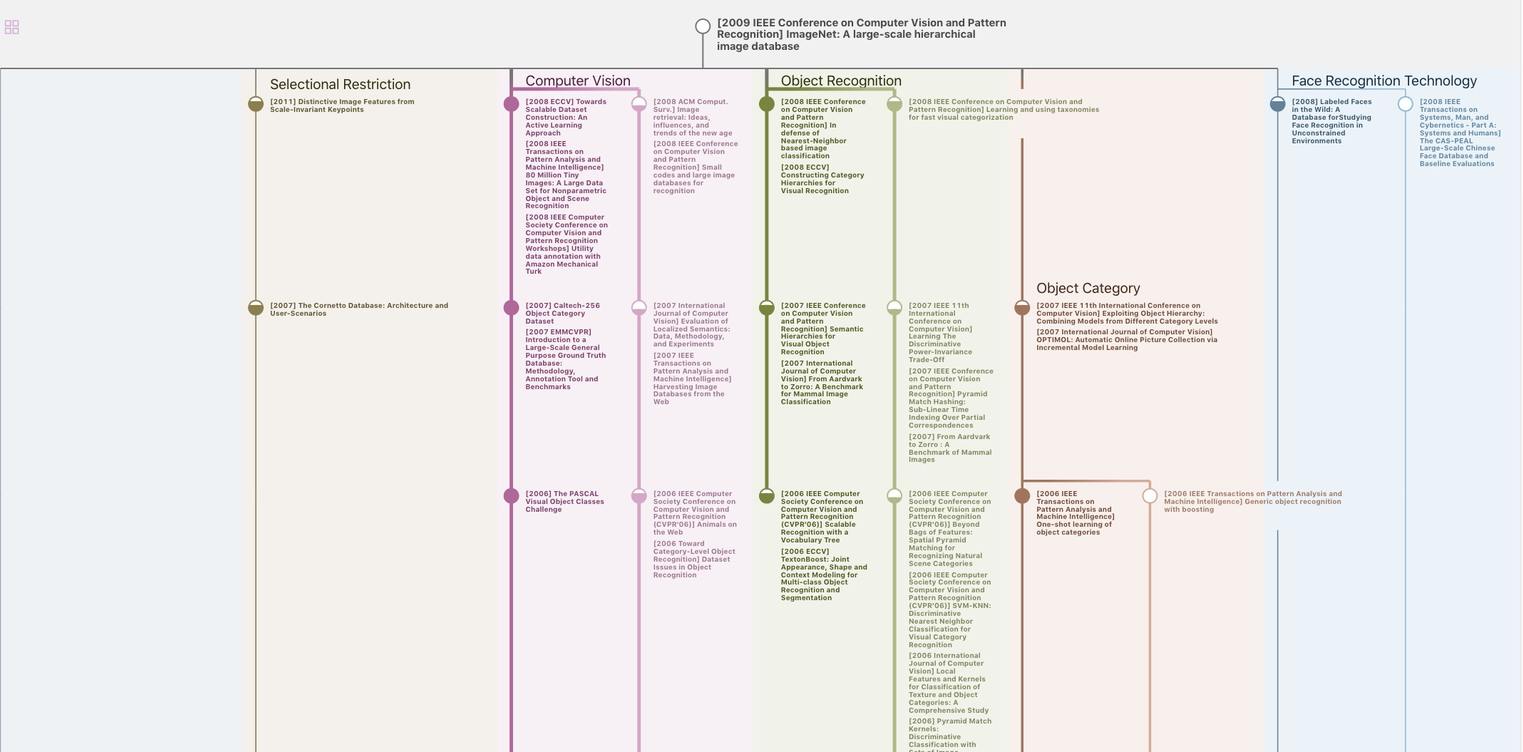
生成溯源树,研究论文发展脉络
Chat Paper
正在生成论文摘要