Deep Learning in Random Neural Fields: Numerical Experiments via Neural Tangent Kernel.
CoRR(2022)
摘要
A biological neural network in the cortex forms a neural field. Neurons in the field have their own receptive fields, and connection weights between two neurons are random but highly correlated when they are in close proximity in receptive fields. In this paper, we investigate such neural fields in a multilayer architecture to investigate the supervised learning of the fields. We empirically compare the performances of our field model with those of randomly connected deep networks. The behavior of a randomly connected network is investigated on the basis of the key idea of the neural tangent kernel regime, a recent development in the machine learning theory of over-parameterized networks; for most randomly connected neural networks, it is shown that global minima always exist in their small neighborhoods. We numerically show that this claim also holds for our neural fields. In more detail, our model has two structures: (i) each neuron in a field has a continuously distributed receptive field, and (ii) the initial connection weights are random but not independent, having correlations when the positions of neurons are close in each layer. We show that such a multilayer neural field is more robust than conventional models when input patterns are deformed by noise disturbances. Moreover, its generalization ability can be slightly superior to that of conventional models.
更多查看译文
关键词
Neural tangent kernel,Random neural field,Reproducing kernel Hilbert space,Supervised learning
AI 理解论文
溯源树
样例
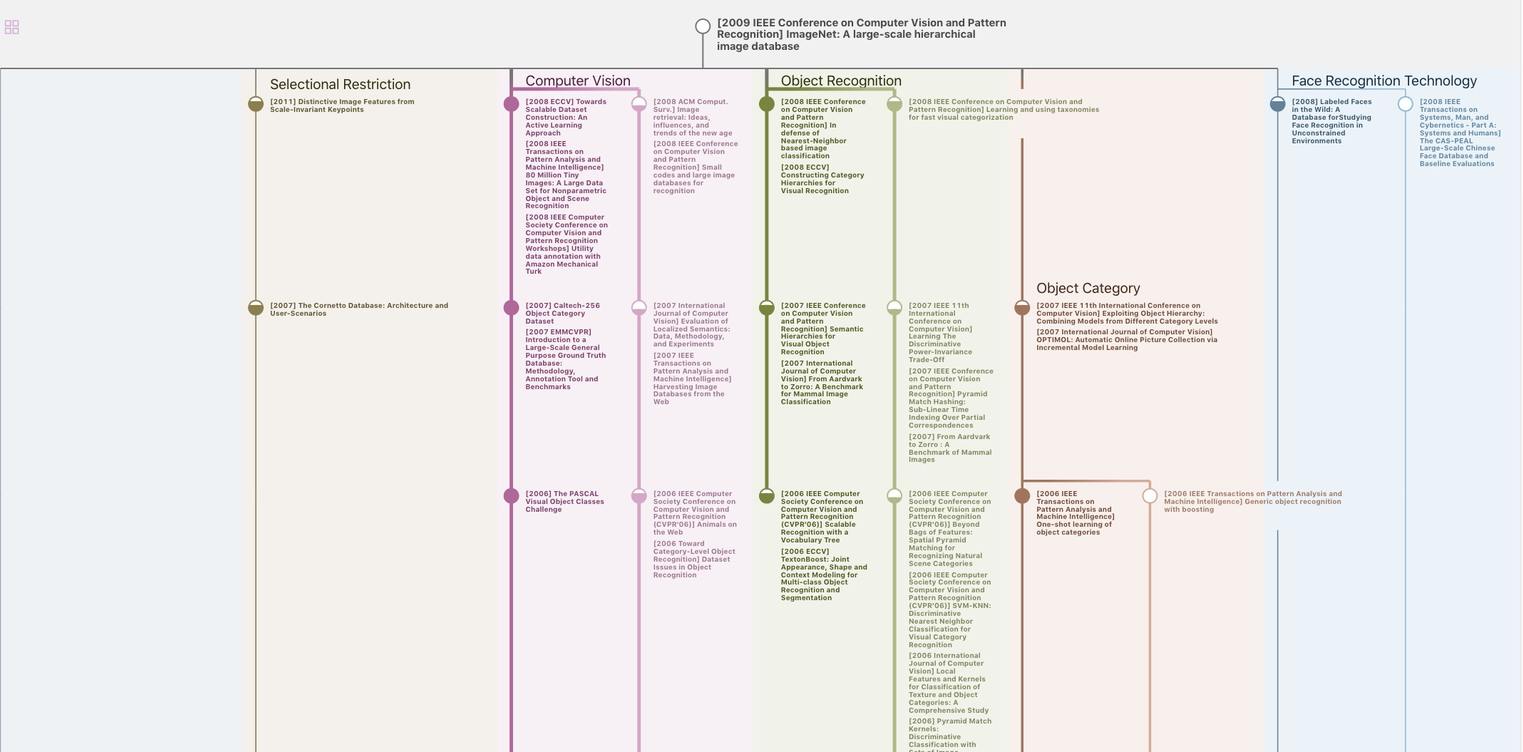
生成溯源树,研究论文发展脉络
Chat Paper
正在生成论文摘要