Anc2vec: embedding gene ontology terms by preserving ancestors relationships
BRIEFINGS IN BIOINFORMATICS(2022)
摘要
The gene ontology (GO) provides a hierarchical structure with a controlled vocabulary composed of terms describing functions and localization of gene products. Recent works propose vector representations, also known as embeddings, of GO terms that capture meaningful information about them. Significant performance improvements have been observed when these representations are used on diverse downstream tasks, such as the measurement of semantic similarity between GO terms and functional similarity between proteins. Despite the success shown by these approaches, existing embeddings of GO terms still fail to capture crucial structural features of the GO. Here, we present anc2vec, a novel protocol based on neural networks for constructing vector representations of GO terms by preserving three important ontological features: its ontological uniqueness, ancestors hierarchy and sub-ontology membership. The advantages of using anc2vec are demonstrated by systematic experiments on diverse tasks: visualization, sub-ontology prediction, inference of structurally related terms, retrieval of terms from aggregated embeddings, and prediction of protein-protein interactions. In these tasks, experimental results show that the performance of anc2vec representations is better than those of recent approaches. This demonstrates that higher performances on diverse tasks can be achieved by embeddings when the structure of the GO is better represented. Full source code and data are available at https://github.com/sinclab/anc2vec.
更多查看译文
关键词
gene ontology, neural networks, semantic similarity, protein-protein interactions
AI 理解论文
溯源树
样例
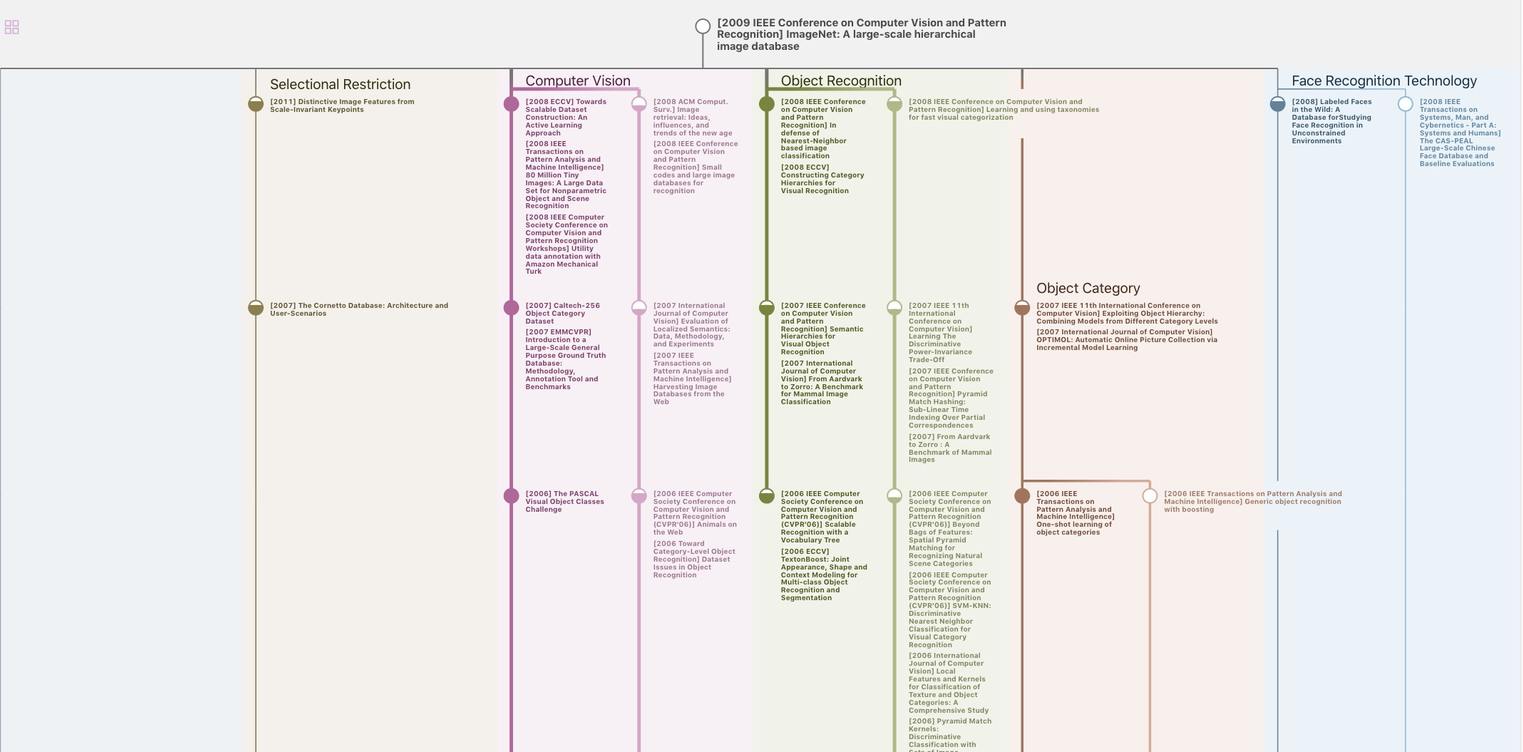
生成溯源树,研究论文发展脉络
Chat Paper
正在生成论文摘要