CausPref: Causal Preference Learning for Out-of-Distribution Recommendation
International World Wide Web Conference(2022)
摘要
ABSTRACT In spite of the tremendous development of recommender system owing to the progressive capability of machine learning recently, the current recommender system is still vulnerable to the distribution shift of users and items in realistic scenarios, leading to the sharp decline of performance in testing environments. It is even more severe in many common applications where only the implicit feedback from sparse data is available. Hence, it is crucial to promote the performance stability of recommendation method in different environments. In this work, we first make a thorough analysis of implicit recommendation problem from the viewpoint of out-of-distribution (OOD) generalization. Then under the guidance of our theoretical analysis, we propose to incorporate the recommendation-specific DAG learner into a novel causal preference-based recommendation framework named CausPref, mainly consisting of causal learning of invariant user preference and anti-preference negative sampling to deal with implicit feedback. Extensive experimental results from real-world datasets clearly demonstrate that our approach surpasses the benchmark models significantly under types of out-of-distribution settings, and show its impressive interpretability.
更多查看译文
关键词
out-of-distribution recommendation, stable recommender system, causal structure learning, user preference
AI 理解论文
溯源树
样例
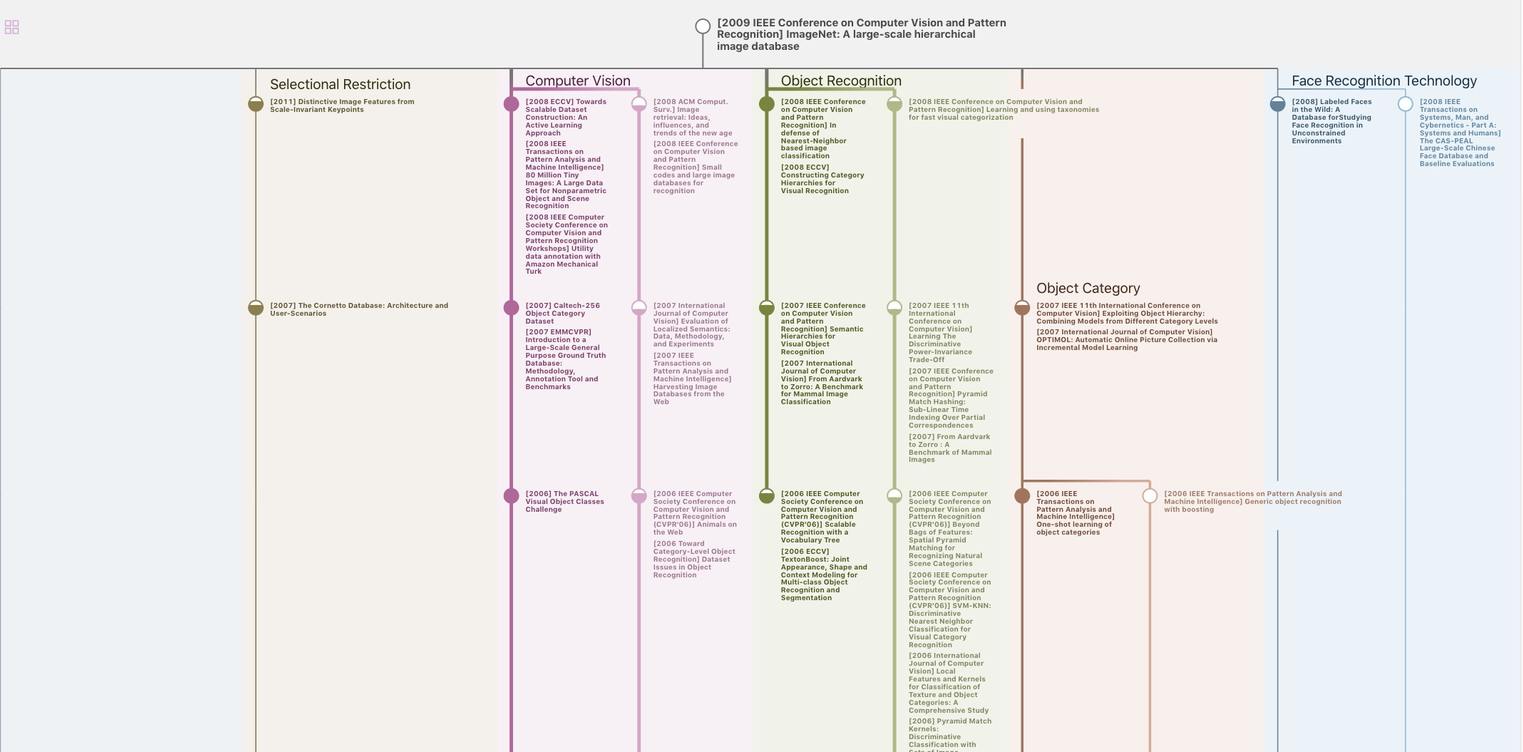
生成溯源树,研究论文发展脉络
Chat Paper
正在生成论文摘要