On The Multi-View Information Bottleneck Representation
2022 IEEE Information Theory Workshop (ITW)(2022)
摘要
In this work, we generalize the information bottleneck (IB) approach to the multi-view learning context. The exponentially growing complexity of the optimal representation motivates the development of two novel formulations with more favorable performance-complexity tradeoffs. The first approach is based on forming a stochastic consensus and is suited for scenarios with significant representation overlap between the different views. The second method, relying on incremental updates, is tailored for the other extreme scenario with minimal representation overlap. In both cases, we extend our earlier work on the alternating directional methods of multiplier (ADMM) solver and establish its convergence and scalability. Empirically, we find that the proposed methods outperform state-of-the-art approaches in multi-view classification problems under a broad range of modelling parameters.
更多查看译文
关键词
Information bottleneck,consensus ADMM,non-convex optimization,classification,multi-view learning
AI 理解论文
溯源树
样例
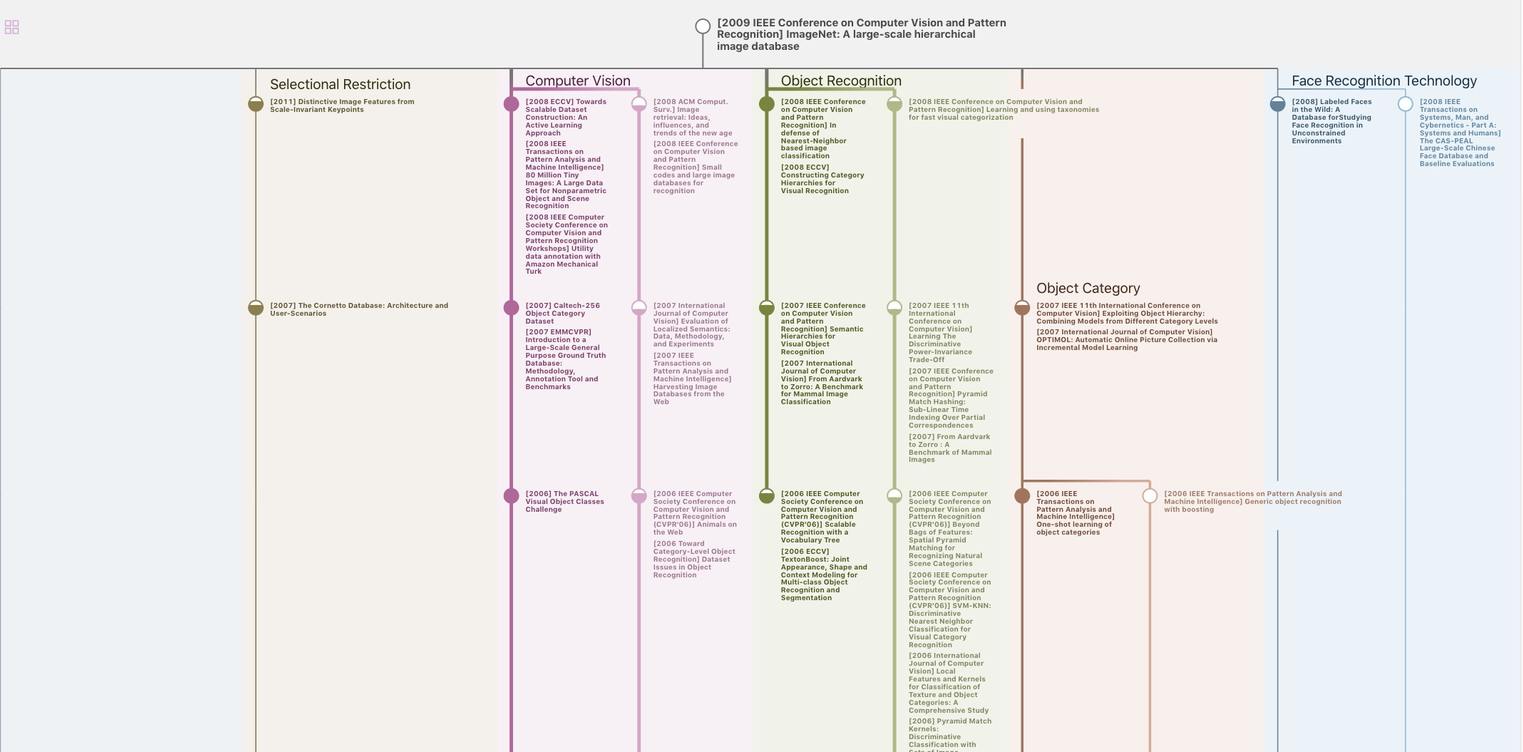
生成溯源树,研究论文发展脉络
Chat Paper
正在生成论文摘要