Training Differentially Private Models with Secure Multiparty Computation.
IACR Cryptology ePrint Archive(2022)
摘要
We address the problem of learning a machine learning model from training data that originates at multiple data owners while providing formal privacy guarantees regarding the protection of each owner's data. Existing solutions based on Differential Privacy (DP) achieve this at the cost of a drop in accuracy. Solutions based on Secure Multiparty Computation (MPC) do not incur such accuracy loss but leak information when the trained model is made publicly available. We propose an MPC solution for training DP models. Our solution relies on an MPC protocol for model training, and an MPC protocol for perturbing the trained model coefficients with Laplace noise in a privacy-preserving manner. The resulting MPC+DP approach achieves higher accuracy than a pure DP approach while providing the same formal privacy guarantees. Our work obtained first place in the iDASH2021 Track III competition on confidential computing for secure genome analysis.
更多查看译文
关键词
Privacy-preserving machine learning,Secure multi party computation,Differential privacy
AI 理解论文
溯源树
样例
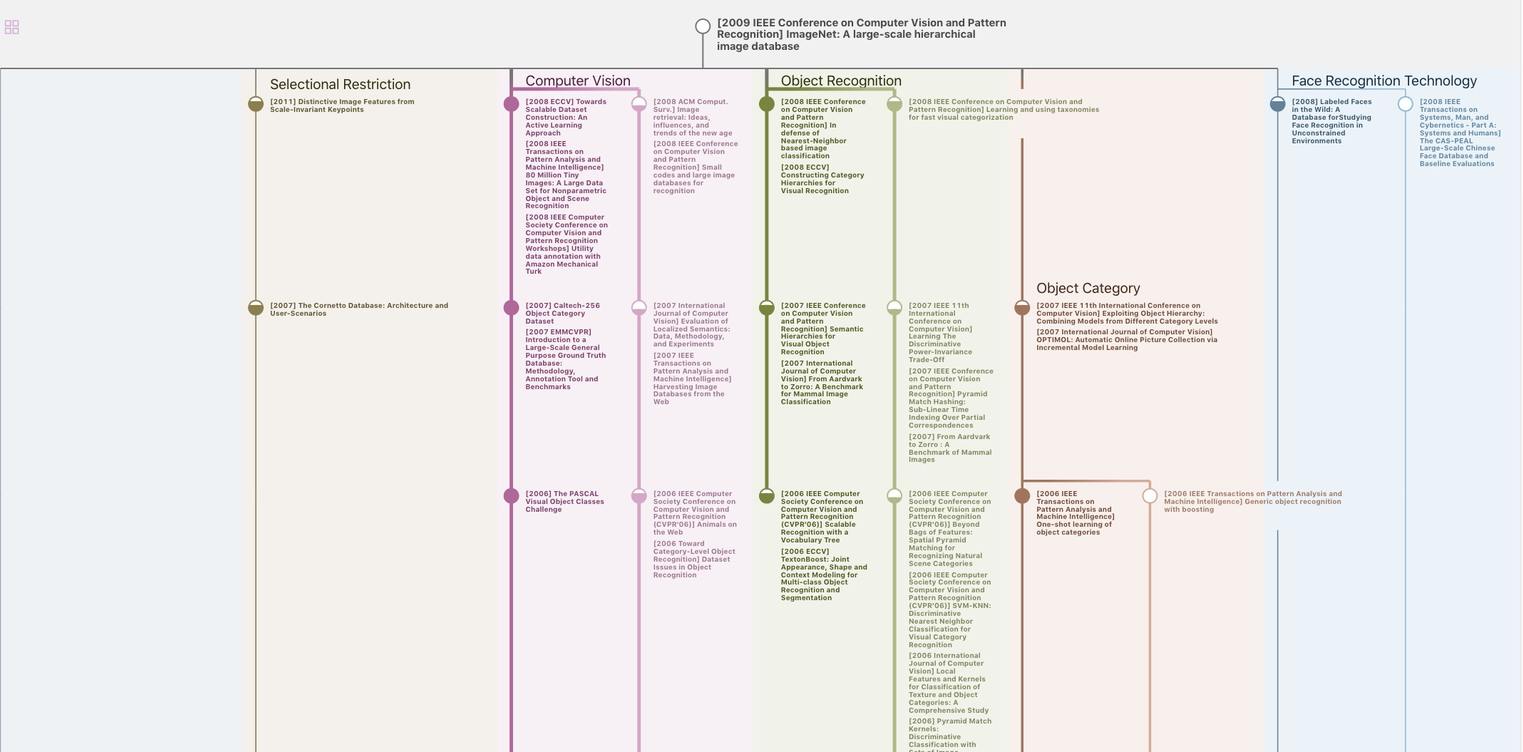
生成溯源树,研究论文发展脉络
Chat Paper
正在生成论文摘要