The challenges facing deep learning-based catheter localization for ultrasound guided high-dose-rate prostate brachytherapy.
MEDICAL PHYSICS(2022)
摘要
BACKGROUND:Automated catheter localization for ultrasound guided high-dose-rate prostate brachytherapy faces challenges relating to imaging noise and artifacts. To date, catheter reconstruction during the clinical procedure is performed manually. Deep learning has been successfully applied to a wide variety of complex tasks and has the potential to tackle the unique challenges associated with multiple catheter localization on ultrasound. Such a task is well suited for automation, with the potential to improve productivity and reliability.
PURPOSE:We developed a deep learning model for automated catheter reconstruction and investigated potential factors influencing model performance. The model was designed to integrate into a clinical workflow, with a proposed reconstruction confidence metric to aid in planner verification.
METHODS:Datasets from 242 patients treated from 2016 to 2020 were collected retrospectively. The anonymized dataset comprises 31,000 transverse images reconstructed from 3D sagittal ultrasound acquisitions and 3500 implanted catheters manually localized by the planner. Each catheter was retrospectively ranked based on the severity of imaging artifacts affecting reconstruction difficulty. The U-NET deep learning architecture was trained to localize implanted catheters on transverse images. A fivefold cross-validation method was used, allowing for evaluation over the entire dataset. The postprocessing software combined the predictions with patient-specific implant information to reconstructed catheters in 3D space, uniquely matched to the implanted grid positions. A reconstruction confidence metric was calculated based on the number and probability of localized predictions per catheter. For each patient, deep learning prediction and postprocessing reconstruction were completed in under 2 min on a nonperformance PC.
RESULTS:Overall, 80% of catheter reconstructions were accurate, within 2 mm along 90% of the length. The catheter tip was often not detected and required extrapolation during reconstruction. The reconstruction accuracy was 89% for the easiest catheter ranking and decreased to 13% for the highest difficulty ranking, when the aid of live ultrasound would have been recommended. Even when limited to the easiest ranked catheters, the reconstruction accuracy decreased at distal grid positions, down to 50%. Individual implantation style was found to influence the frequency of severe artifacts, slightly impacting the model accuracy. A reconstruction confidence metric identified the difficult catheters, removed the observed individual variation, and increased the overall accuracy to 91% while excluding 27% of the reconstructions.
CONCLUSIONS:The deep learning model localized implanted catheters over a large clinical dataset, with overall promising results. The model faced challenges due to ultrasound artifacts and image degradation distal to the probe, underlining the continued importance of maintaining image quality and minimizing artifacts. A potential workflow for integration into the clinical procedure was demonstrated, including the use of a confidence metric to predict low accuracy reconstructions. Comparison between models evaluated on different datasets should also consider underlying differences, such as the frequency and severity of imaging artifacts.
更多查看译文
关键词
deep learning, high-dose-rate prostate brachytherapy, image segmentation
AI 理解论文
溯源树
样例
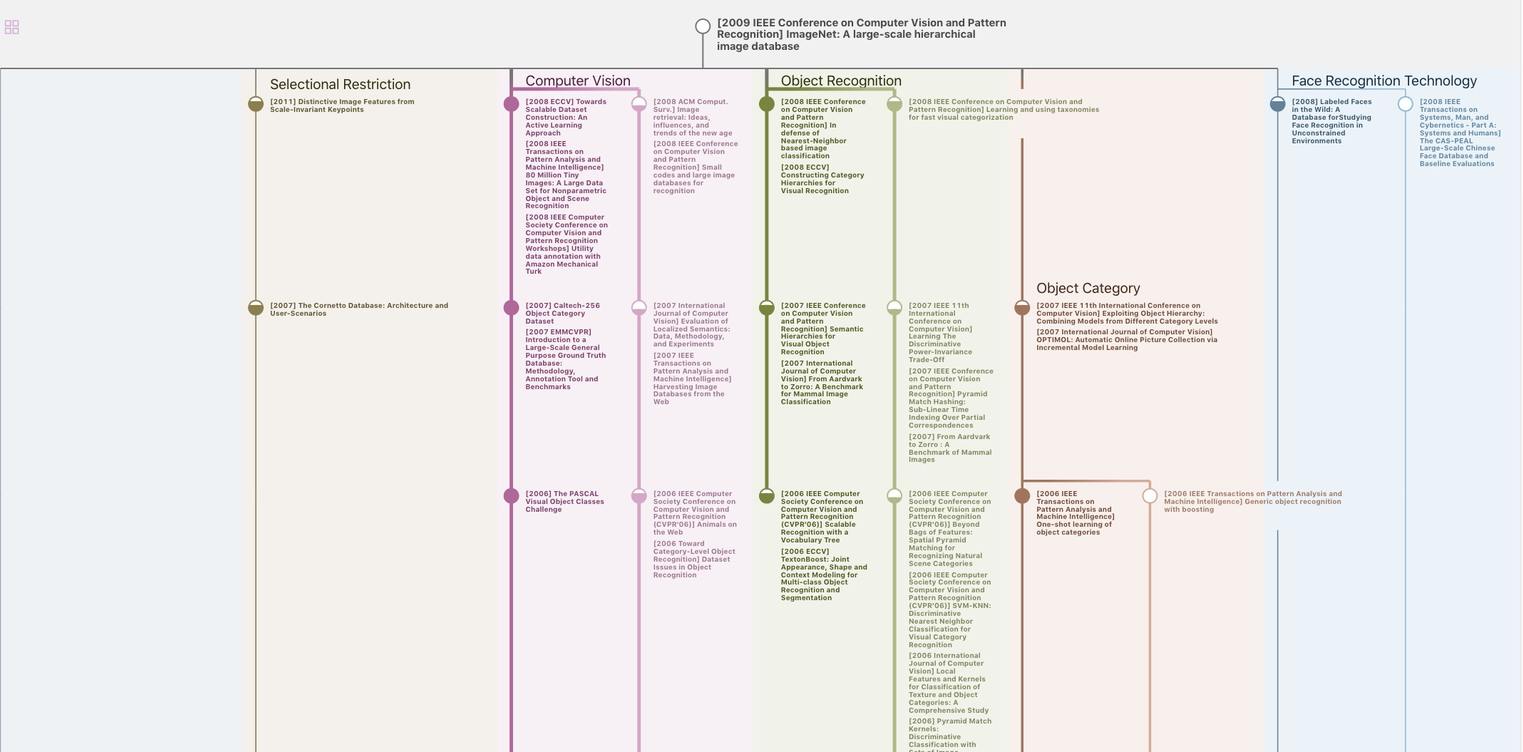
生成溯源树,研究论文发展脉络
Chat Paper
正在生成论文摘要