CoST: Contrastive Learning of Disentangled Seasonal-Trend Representations for Time Series Forecasting
International Conference on Learning Representations (ICLR)(2022)
摘要
Deep learning has been actively studied for time series forecasting, and the mainstream paradigm is based on the end-to-end training of neural network architectures, ranging from classical LSTM/RNNs to more recent TCNs and Transformers. Motivated by the recent success of representation learning in computer vision and natural language processing, we argue that a more promising paradigm for time series forecasting, is to first learn disentangled feature representations, followed by a simple regression fine-tuning step -- we justify such a paradigm from a causal perspective. Following this principle, we propose a new time series representation learning framework for time series forecasting named CoST, which applies contrastive learning methods to learn disentangled seasonal-trend representations. CoST comprises both time domain and frequency domain contrastive losses to learn discriminative trend and seasonal representations, respectively. Extensive experiments on real-world datasets show that CoST consistently outperforms the state-of-the-art methods by a considerable margin, achieving a 21.3\% improvement in MSE on multivariate benchmarks. It is also robust to various choices of backbone encoders, as well as downstream regressors.
更多查看译文
关键词
Time Series,Representation Learning,Forecasting,Self-Supervised Learning
AI 理解论文
溯源树
样例
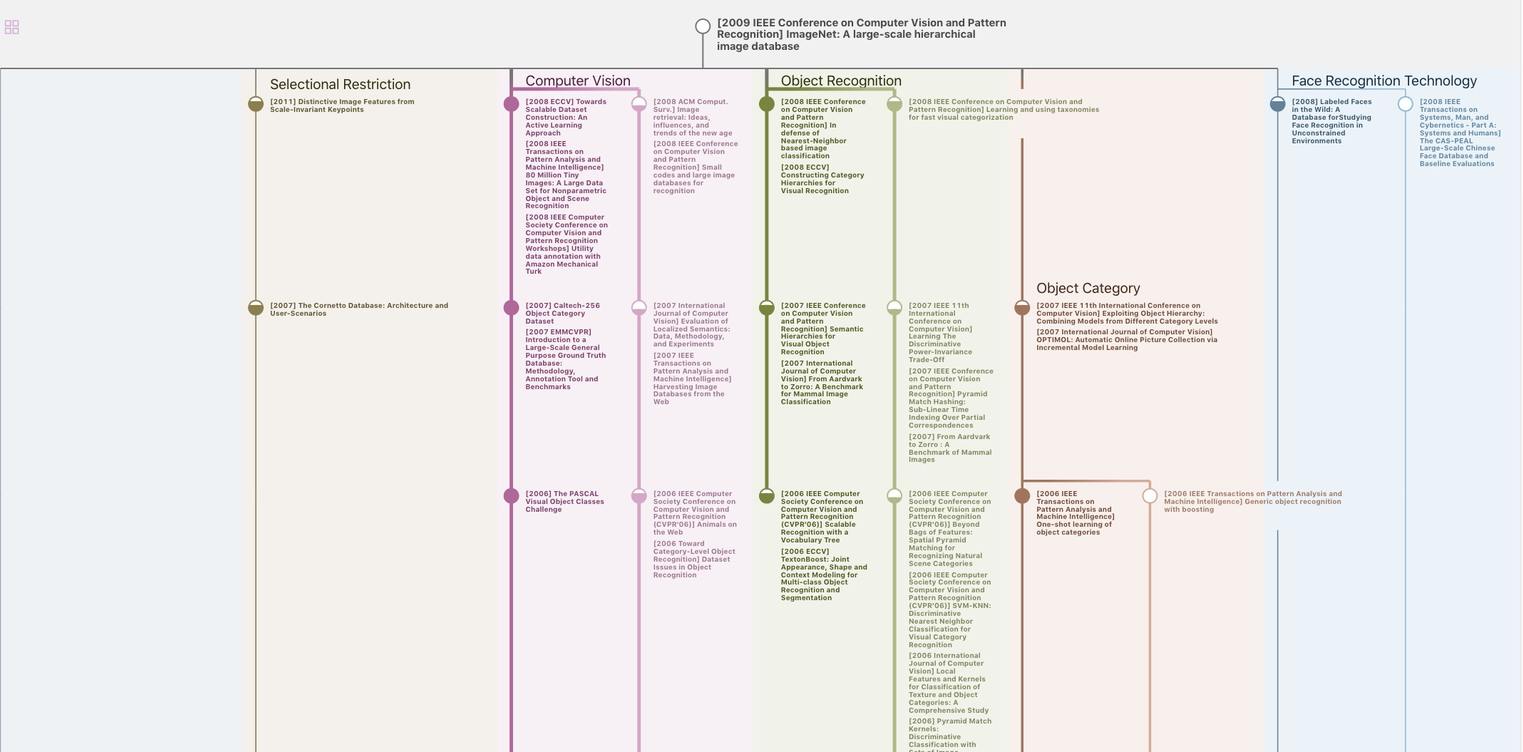
生成溯源树,研究论文发展脉络
Chat Paper
正在生成论文摘要