Interpretability for Multimodal Emotion Recognition using Concept Activation Vectors
2022 International Joint Conference on Neural Networks (IJCNN)(2022)
摘要
Multimodal Emotion Recognition refers to the classification of input video sequences into emotion labels based on multiple input modalities (usually video, audio and text). In recent years, Deep Neural networks have shown remarkable performance in recognizing human emotions, and are on par with human-level performance on this task. Despite of recent advancements in this field, emotion recognition systems are yet to be accepted for real world setups due to the obscure nature of their reasoning and decision-making process. Most of the research in this field deals with novel architectures to improve the performance for this task, with a few attempts at providing explanations for these models' decisions. In this paper, we address the issue of interpretability for neural networks in the context of emotion recognition using Concept Activation Vectors (CAVs). To analyse the model's latent space, we define human-understandable concepts specific to Emotion AI and map them to the widely-used IEMOCAP multimodal database. We then evaluate the influence of our proposed concepts at multiple layers of the Bi-directional Contextual LSTM (BC-LSTM) network to show that the reasoning process of neural networks for emotion recognition can be represented using human-understandable concepts. Finally, we perform hypothesis testing on our proposed concepts to show that they are significant for interpretability of this task.
更多查看译文
关键词
Multimodal Emotion Recognition,Interpretability,Concept Activation Vectors,Emotion AI
AI 理解论文
溯源树
样例
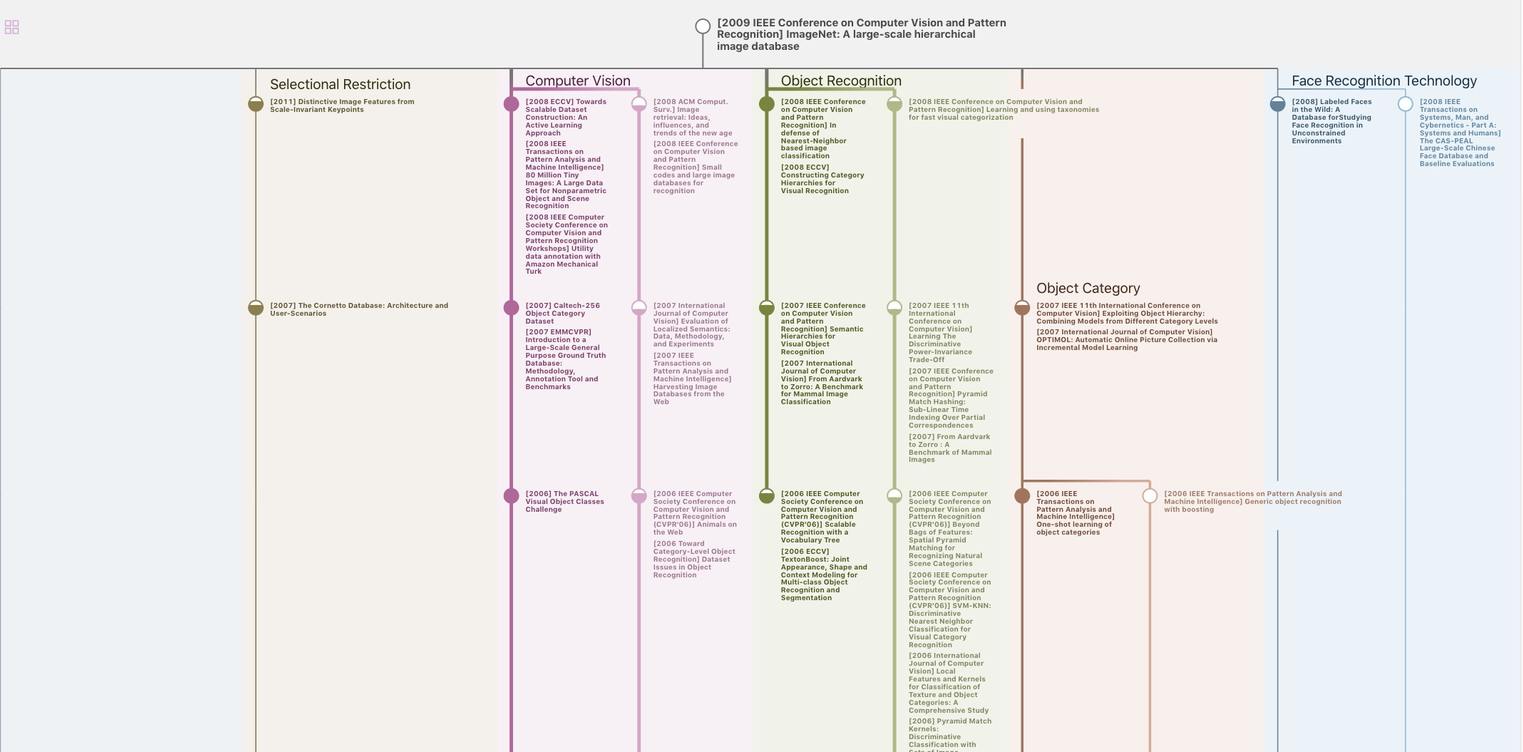
生成溯源树,研究论文发展脉络
Chat Paper
正在生成论文摘要