An unbiased algorithm for objective separation of Alzheimer’s, Alzheimer’s mixed with cerebrovascular symptomology, and healthy controls from one another using electrovestibulography (EVestG)
MEDICAL & BIOLOGICAL ENGINEERING & COMPUTING(2022)
摘要
Diagnosis of Alzheimer’s disease (AD) from AD with cerebrovascular disease pathology (AD-CVD) is a rising challenge. Using electrovestibulography (EVestG) measured signals, we develop an automated feature extraction and selection algorithm for an unbiased identification of AD and AD-CVD from healthy controls as well as their separation from each other. EVestG signals of 24 healthy controls, 16 individuals with AD, and 13 with AD-CVD were analyzed within two separate groupings: One-versus-One and One-versus-All. A multistage feature selection process was conducted over the training dataset using linear support vector machine (SVM) classification with 10-fold cross-validation, k nearest neighbors/averaging imputation, and exhaustive search. The most frequently selected features that achieved highest classification performance were selected. 10-fold cross-validation was applied via a linear SVM classification on the entire dataset. Multivariate analysis was run to test the between population differences while controlling for the covariates. Classification accuracies of ≥ 80% and 78% were achieved for the One-versus-All classification approach and AD versus AD-CVD separation, respectively. The results also held true after controlling for the effect of covariates. AD/AD-CVD participants showed smaller/larger EVestG averaged field potential signals compared to healthy controls and AD-CVD/AD participants. These characteristics are in line with our previous study results. Graphical abstract
更多查看译文
关键词
Feature selection, Classification, Electrovestibulography, Alzheimer's Disease, Cerebrovascular pathology
AI 理解论文
溯源树
样例
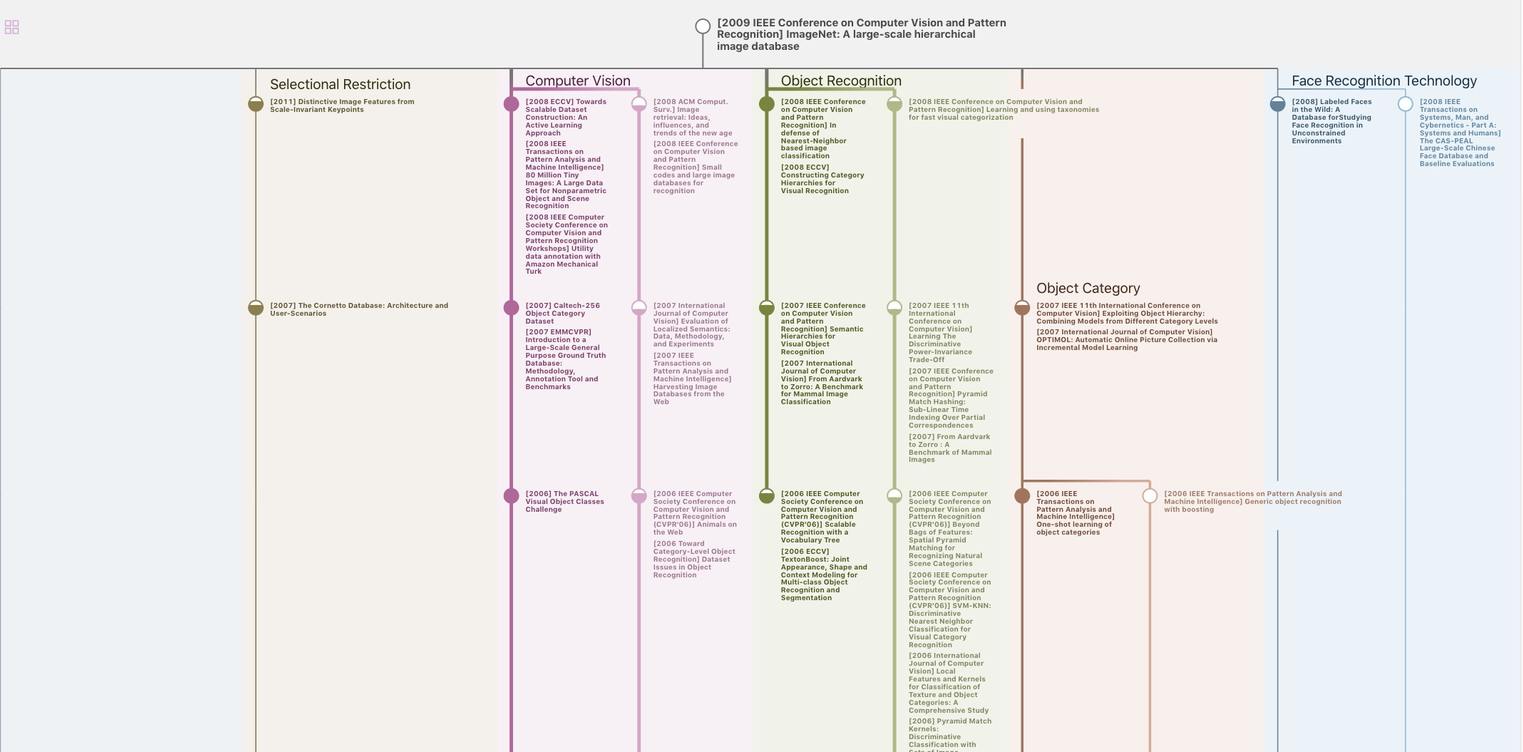
生成溯源树,研究论文发展脉络
Chat Paper
正在生成论文摘要