When Do Flat Minima Optimizers Work?
arxiv(2023)
摘要
Recently, flat-minima optimizers, which seek to find parameters in low-loss neighborhoods, have been shown to improve a neural network's generalization performance over stochastic and adaptive gradient-based optimizers. Two methods have received significant attention due to their scalability: 1. Stochastic Weight Averaging (SWA), and 2. Sharpness-Aware Minimization (SAM). However, there has been limited investigation into their properties and no systematic benchmarking of them across different domains. We fill this gap here by comparing the loss surfaces of the models trained with each method and through broad benchmarking across computer vision, natural language processing, and graph representation learning tasks. We discover several surprising findings from these results, which we hope will help researchers further improve deep learning optimizers, and practitioners identify the right optimizer for their problem.
更多查看译文
关键词
deep learning,optimization,flatness
AI 理解论文
溯源树
样例
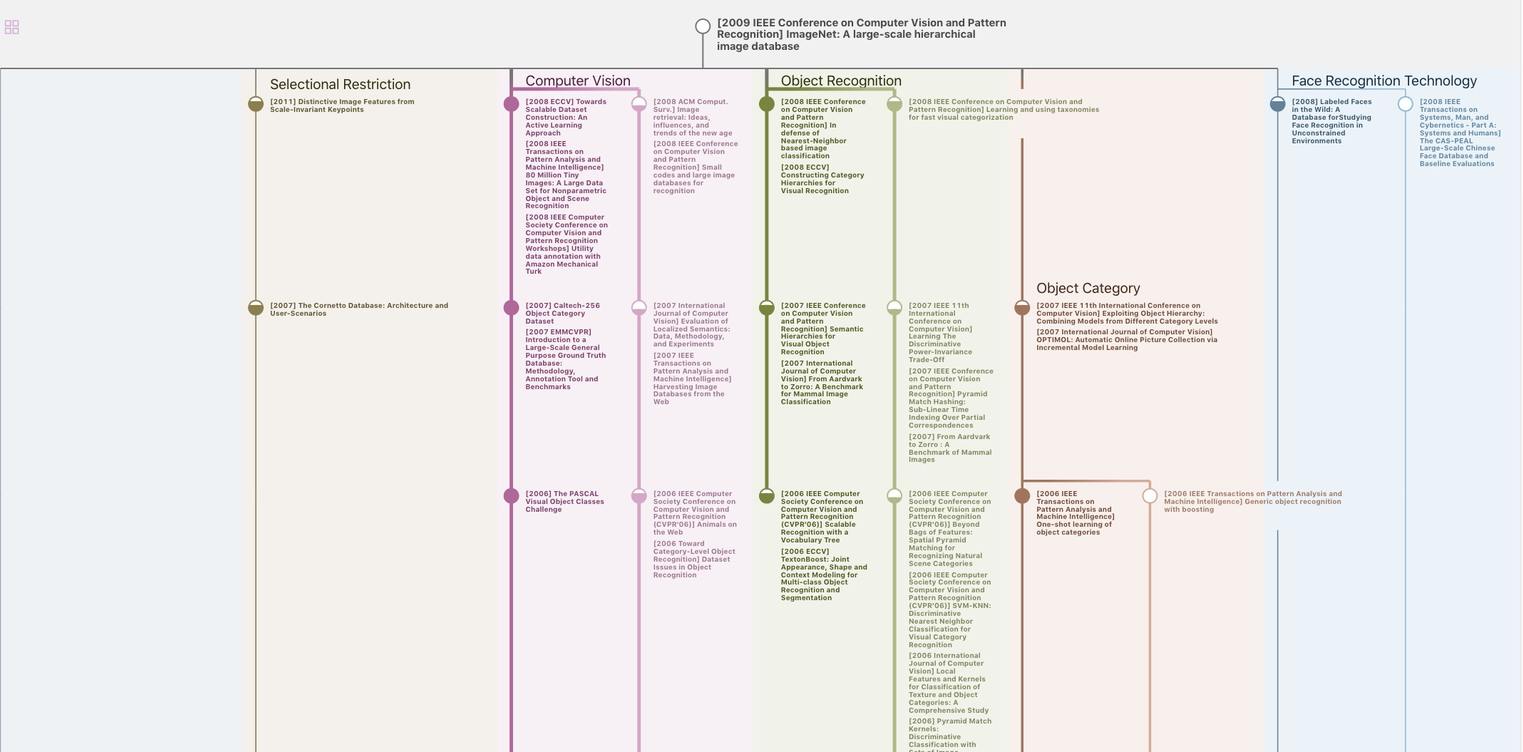
生成溯源树,研究论文发展脉络
Chat Paper
正在生成论文摘要