Bootstrap Confidence Regions for Learned Feature Embeddings
arxiv(2023)
摘要
Algorithmic feature learners provide high-dimensional vector representations for non-matrix structured data, like image or text collections. Low-dimensional projections derived from these representations, called embeddings, are often used to explore variation in these data. However, it is not clear how to assess the embedding uncertainty. We adapt methods developed for bootstrapping principal components analysis to the setting where features are algorithmically derived from nonmatrix data. We empirically compare the derived confidence areas in simulations, varying factors influencing feature learning and the bootstrap, like feature learning algorithm complexity and bootstrap sample size. We illustrate the proposed approaches on a spatial proteomics dataset, where we observe that embedding precision is not uniform across all tissue types. Code, data, and pretrained models are available as an R compendium in the . Supplementary files for this article are available online.
更多查看译文
关键词
Bootstrap,resampling,Data visualization,Dimension reduction,Machine learning
AI 理解论文
溯源树
样例
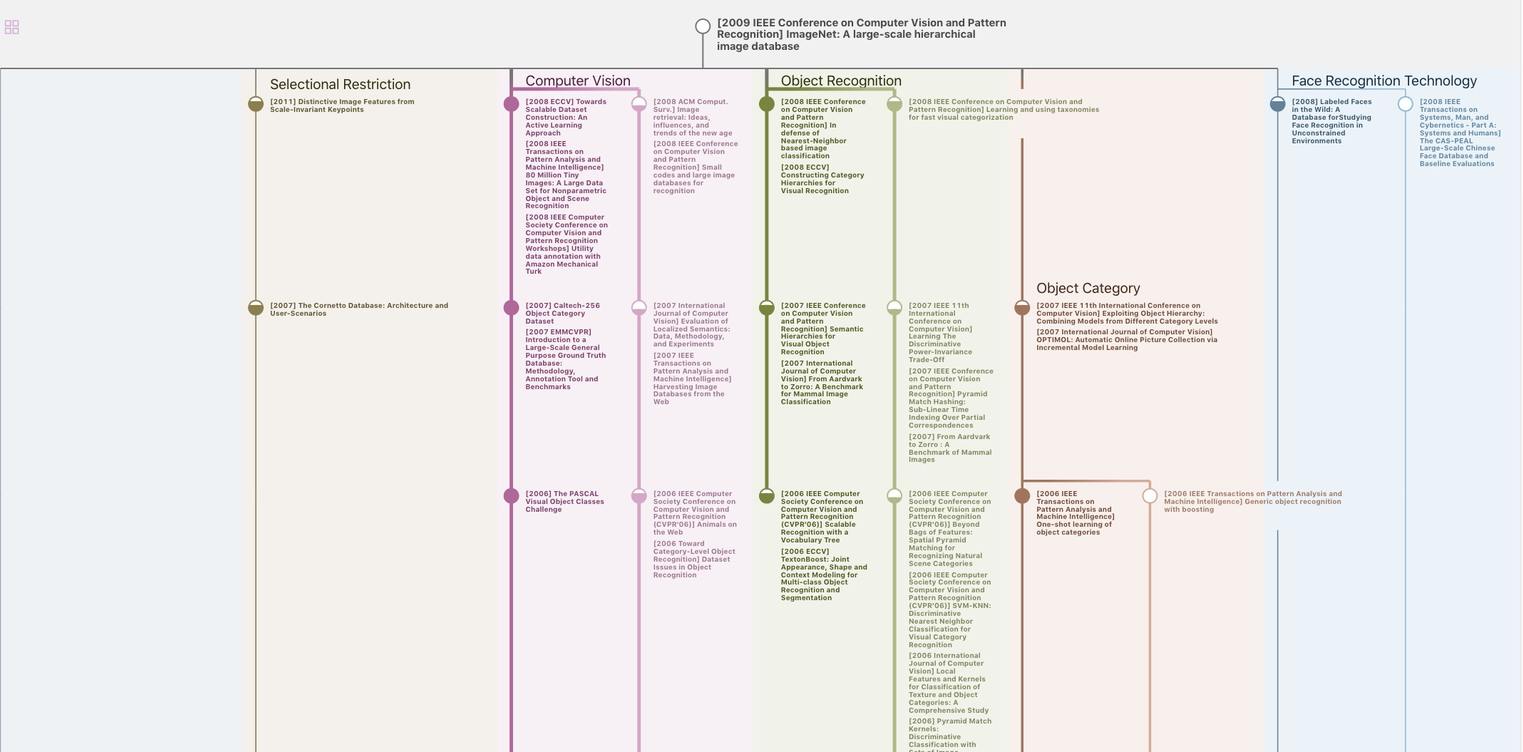
生成溯源树,研究论文发展脉络
Chat Paper
正在生成论文摘要