CLA-NeRF: Category-Level Articulated Neural Radiance Field
IEEE International Conference on Robotics and Automation(2022)
摘要
We propose CLA-NeRF - a Category-Level Articulated Neural Radiance Field that can perform view synthesis, part segmentation, and articulated pose estimation. CLA-NeRF is trained at the object category level using no CAD models and no depth, but a set of RGB images with ground truth camera poses and part segments. During inference, it only takes a few RGB views (i.e., few-shot) of an unseen 3D object instance within the known category to infer the object part segmentation and the neural radiance field. Given an articulated pose as input, CLA-NeRF can perform articulation-aware volume rendering to generate the corresponding RGB image at any camera pose. Moreover, the articulated pose of an object can be estimated via inverse rendering. In our experiments, we evaluate the framework across five categories on both synthetic and real-world data. In all cases, our method shows realistic deformation results and accurate articulated pose estimation. We believe that both few-shot articulated object rendering and articulated pose estimation open doors for robots to perceive and interact with unseen articulated objects.
更多查看译文
关键词
CLA-NeRF,articulation-aware volume rendering,accurate articulated pose estimation,few-shot articulated object rendering,unseen articulated objects,category-level articulated neural radiance field,object category level,ground truth camera poses,part segments,known category,object part segmentation
AI 理解论文
溯源树
样例
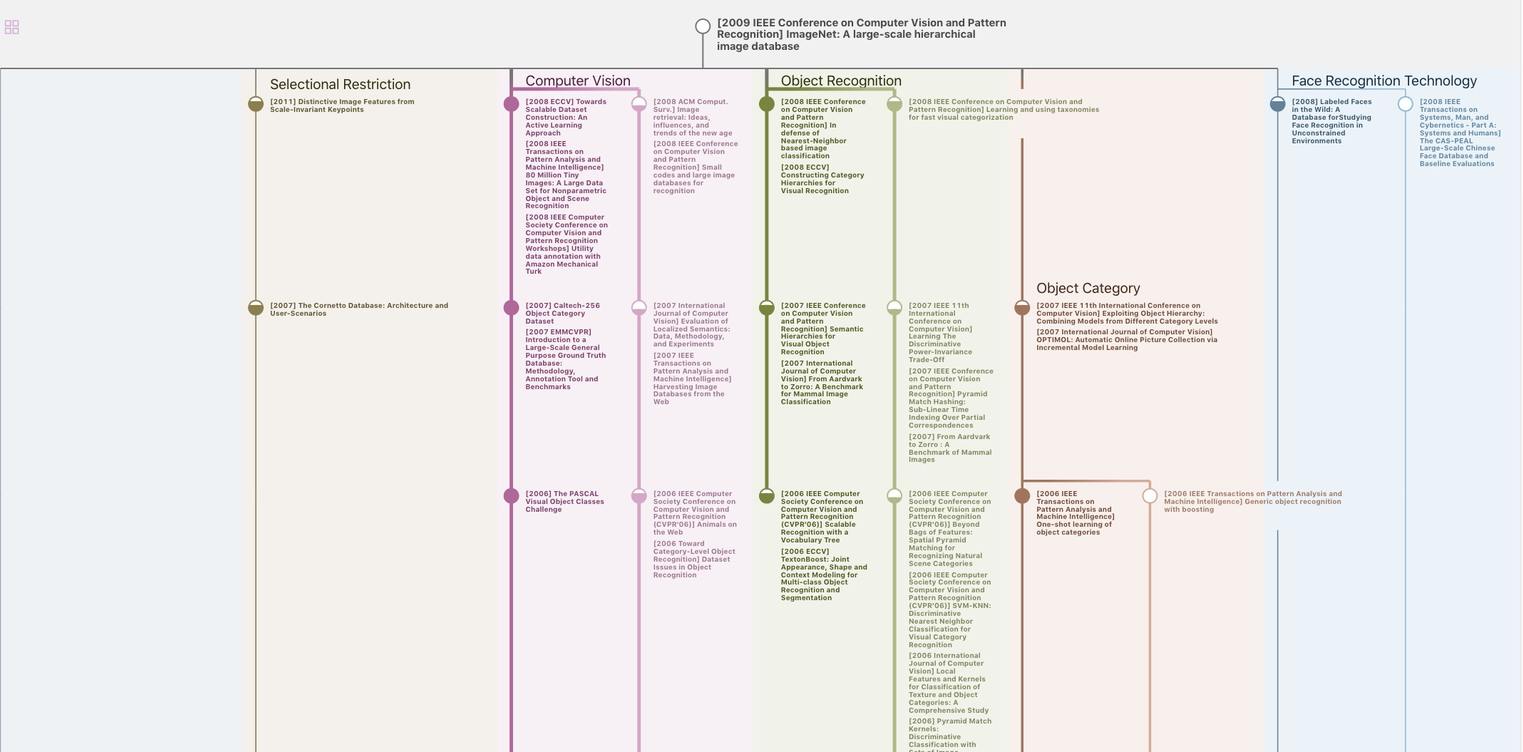
生成溯源树,研究论文发展脉络
Chat Paper
正在生成论文摘要