Robust Generalization of Quadratic Neural Networks via Function Identification
arxiv(2022)
摘要
A key challenge facing deep learning is that neural networks are often not robust to shifts in the underlying data distribution. We study this problem from the perspective of the statistical concept of parameter identification. Generalization bounds from learning theory often assume that the test distribution is close to the training distribution. In contrast, if we can identify the "true" parameters, then the model generalizes to arbitrary distribution shifts. However, neural networks typically have internal symmetries that make parameter identification impossible. We show that we can identify the function represented by a quadratic network even though we cannot identify its parameters; we extend this result to neural networks with ReLU activations. Thus, we can obtain robust generalization bounds for neural networks. We leverage this result to obtain new bounds for contextual bandits and transfer learning with quadratic neural networks. Overall, our results suggest that we can improve robustness of neural networks by designing models that can represent the true data generating process.
更多查看译文
关键词
quadratic neural networks,robust generalization,function identification,neural networks
AI 理解论文
溯源树
样例
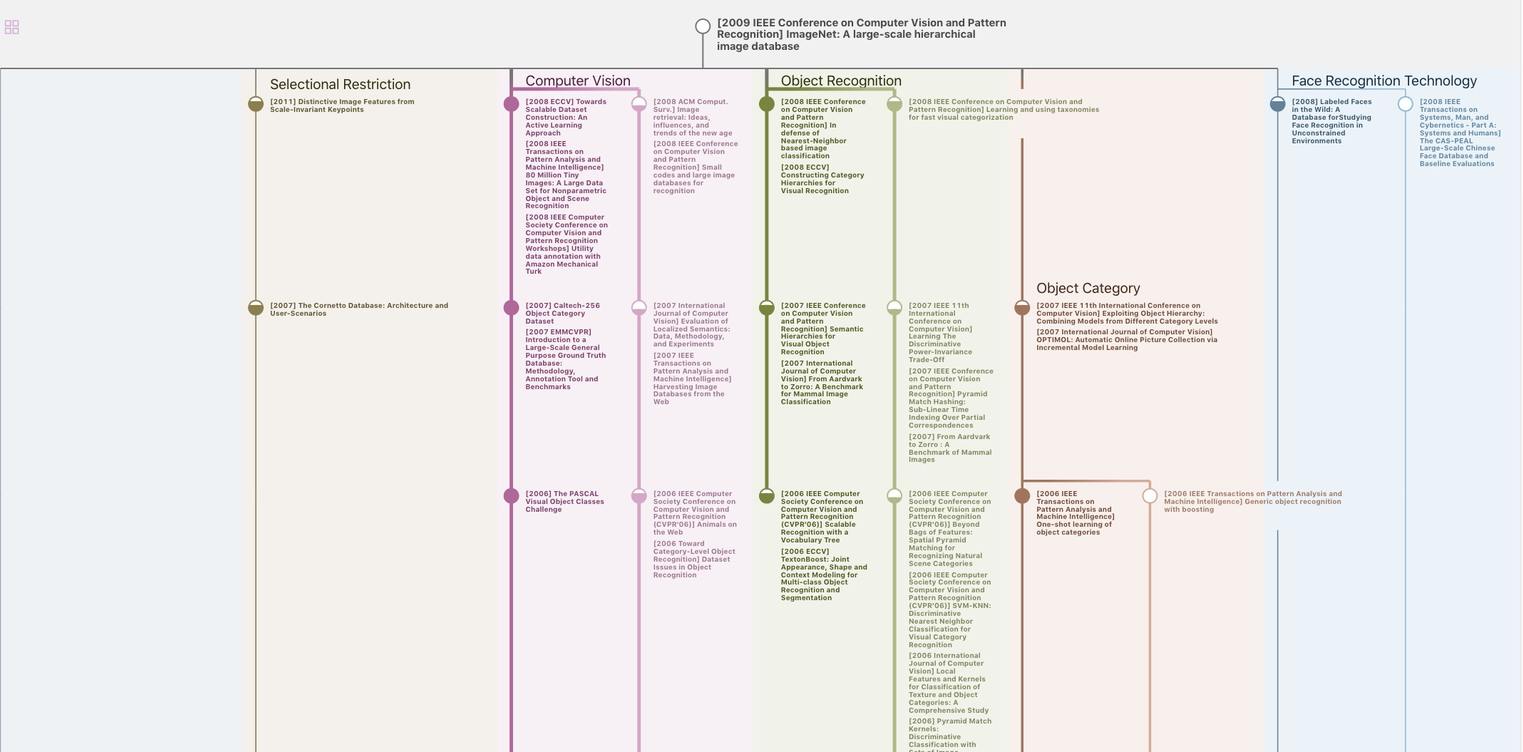
生成溯源树,研究论文发展脉络
Chat Paper
正在生成论文摘要