Sparse Representation-Based Discriminative Metric Learning for Brain MRI Image Retrieval
FRONTIERS IN NEUROSCIENCE(2022)
摘要
Magnetic resonance imaging (MRI) can have a good diagnostic function for important organs and parts of the body. MRI technology has become a common and important disease detection technology. At the same time, medical imaging data is increasing at an explosive rate. Retrieving similar medical images from a huge database is of great significance to doctors' auxiliary diagnosis and treatment. In this paper, combining the advantages of sparse representation and metric learning, a sparse representation-based discriminative metric learning (SRDML) approach is proposed for medical image retrieval of brain MRI. The SRDML approach uses a sparse representation framework to learn robust feature representation of brain MRI, and uses metric learning to project new features into the metric space with matching discrimination. In such a metric space, the optimal similarity measure is obtained by using the local constraints of atoms and the pairwise constraints of coding coefficients, so that the distance between similar images is less than the given threshold, and the distance between dissimilar images is greater than another given threshold. The experiments are designed and tested on the brain MRI dataset created by Chang. Experimental results show that the SRDML approach can obtain satisfactory retrieval performance and achieve accurate brain MRI image retrieval.
更多查看译文
关键词
medical image retrieval, magnetic resonance imaging, brain images, sparse representation, metric learning
AI 理解论文
溯源树
样例
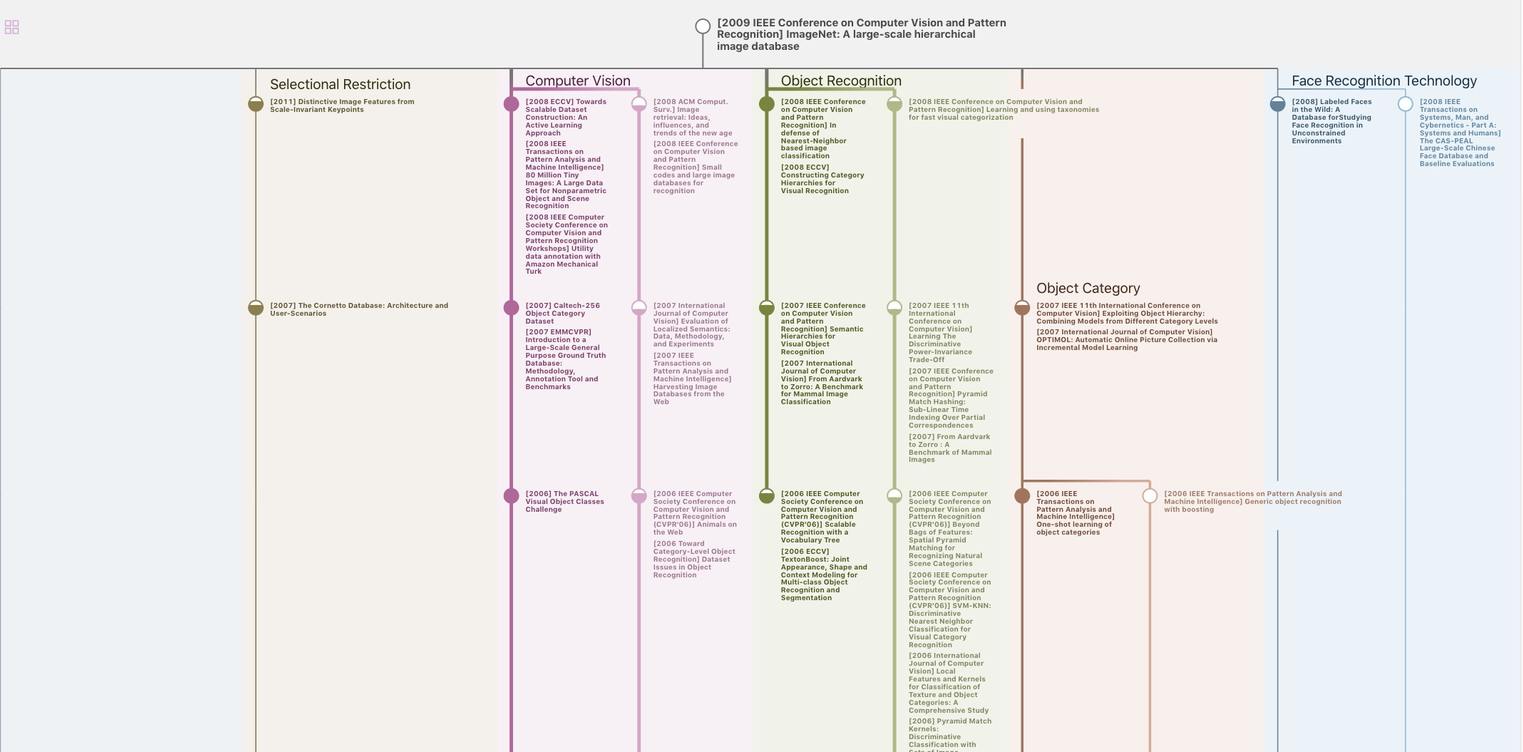
生成溯源树,研究论文发展脉络
Chat Paper
正在生成论文摘要