Integrated pretraining with evolutionary information to improve RNA secondary structure prediction
biorxiv(2022)
摘要
RNA secondary structure prediction is a fundamental task in computational and molecular biology. While machine learning approaches in this area have been shown to improve upon traditional RNA folding algorithms, performance remains limited for several reasons such as the small number of experimentally determined RNA structures and suboptimal use of evolutionary information. To address these challenges, we introduce a practical and effective pretraining strategy that enables learning from a larger set of RNA sequences with computationally predicted structures and in the meantime, tapping into the rich evolutionary information available in databases such as Rfam. Coupled with a flexible and scalable neural architecture that can navigate different learning scenarios while providing ease of integrating evolutionary information, our approach significantly improves upon state-of-the-art across a range of benchmarks, including both single sequence and alignment based structure prediction tasks, with particularly notable benefits on new, less well-studied RNA families.
### Competing Interest Statement
The authors have declared no competing interest.
更多查看译文
关键词
rna,evolutionary information,prediction
AI 理解论文
溯源树
样例
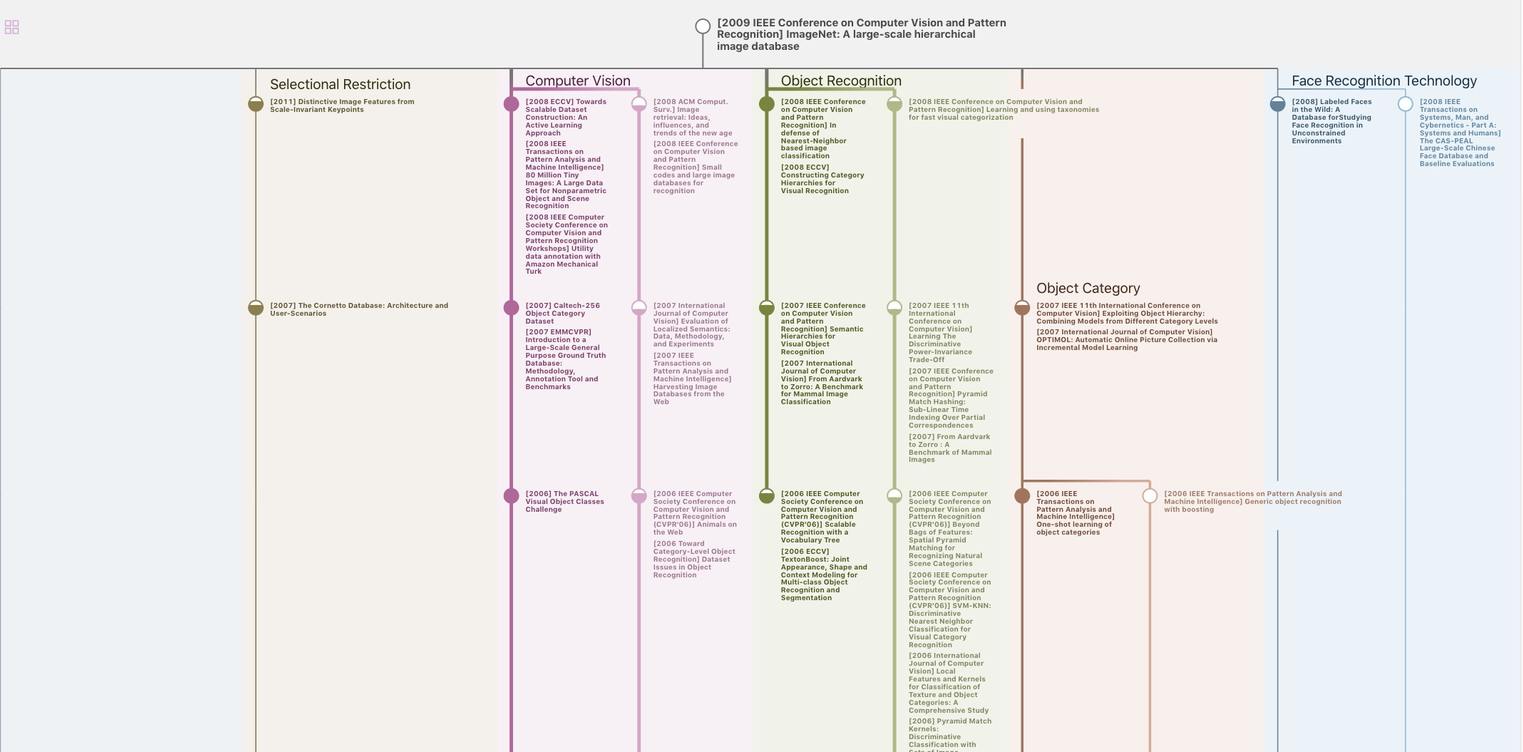
生成溯源树,研究论文发展脉络
Chat Paper
正在生成论文摘要