Excavation Reinforcement Learning Using Geometric Representation
IEEE ROBOTICS AND AUTOMATION LETTERS(2022)
摘要
Excavation of irregular rigid objects in clutter, such as fragmented rocks and wood blocks, is very challenging due to their complex interaction dynamics and highly variable geometries. In this paper, we adopt reinforcement learning (RL) to tackle this challenge and learn policies to plan for a sequence of excavation trajectories for irregular rigid objects, given point clouds of excavation scenes.Moreover, we separately learn a compact representation of the point cloud on geometric tasks that do not require human labeling. We show that using the representation reduces training time for RL, while achieving similar asymptotic performance compare to an end-to-end RL algorithm. When using a policy trained in simulation directly on a real scene, we show that the policy trained with the representation outperforms end-to-end RL. To our best knowledge, this letter presents the first application of RL to plan a sequence of excavation trajectories of irregular rigid objects in clutter.
更多查看译文
关键词
Excavation, Point cloud compression, Task analysis, Trajectory, Planning, Feature extraction, Reinforcement learning, Deep learning in grasping and manipulation, manipulation planning, reinforcement learning
AI 理解论文
溯源树
样例
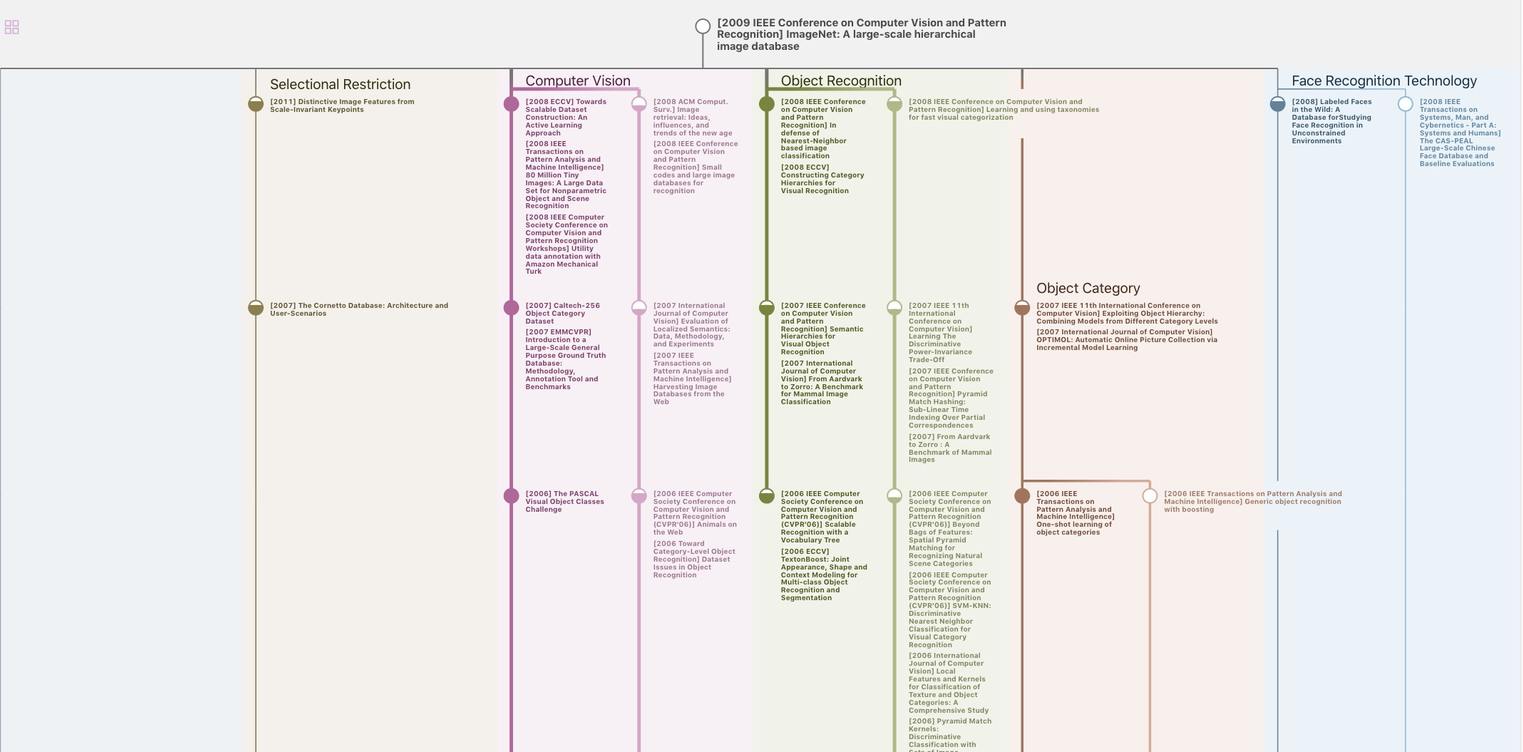
生成溯源树,研究论文发展脉络
Chat Paper
正在生成论文摘要