Machine learning for predicting readmission risk among the frail: Explainable AI for healthcare
Patterns(2022)
摘要
Healthcare costs due to unplanned readmissions are high and negatively affect health and wellness of patients. Hospital readmission is an undesirable outcome for elderly patients. Here, we present readmission risk prediction using five machine learning approaches for predicting 30-day unplanned readmission for elderly patients (age ≥ 50 years). We use a comprehensive and curated set of variables that include frailty, comorbidities, high-risk medications, demographics, hospital, and insurance utilization to build these models. We conduct a large-scale study with electronic health record (her) data with over 145,000 observations from 76,000 patients. Findings indicate that the category boost (CatBoost) model outperforms other models with a mean area under the curve (AUC) of 0.79. We find that prior readmissions, discharge to a rehabilitation facility, length of stay, comorbidities, and frailty indicators were all strong predictors of 30-day readmission. We present in-depth insights using Shapley additive explanations (SHAP), the state of the art in machine learning explainability.
更多查看译文
关键词
readmission risk,machine learning,explainable AI,frailty,comorbidity
AI 理解论文
溯源树
样例
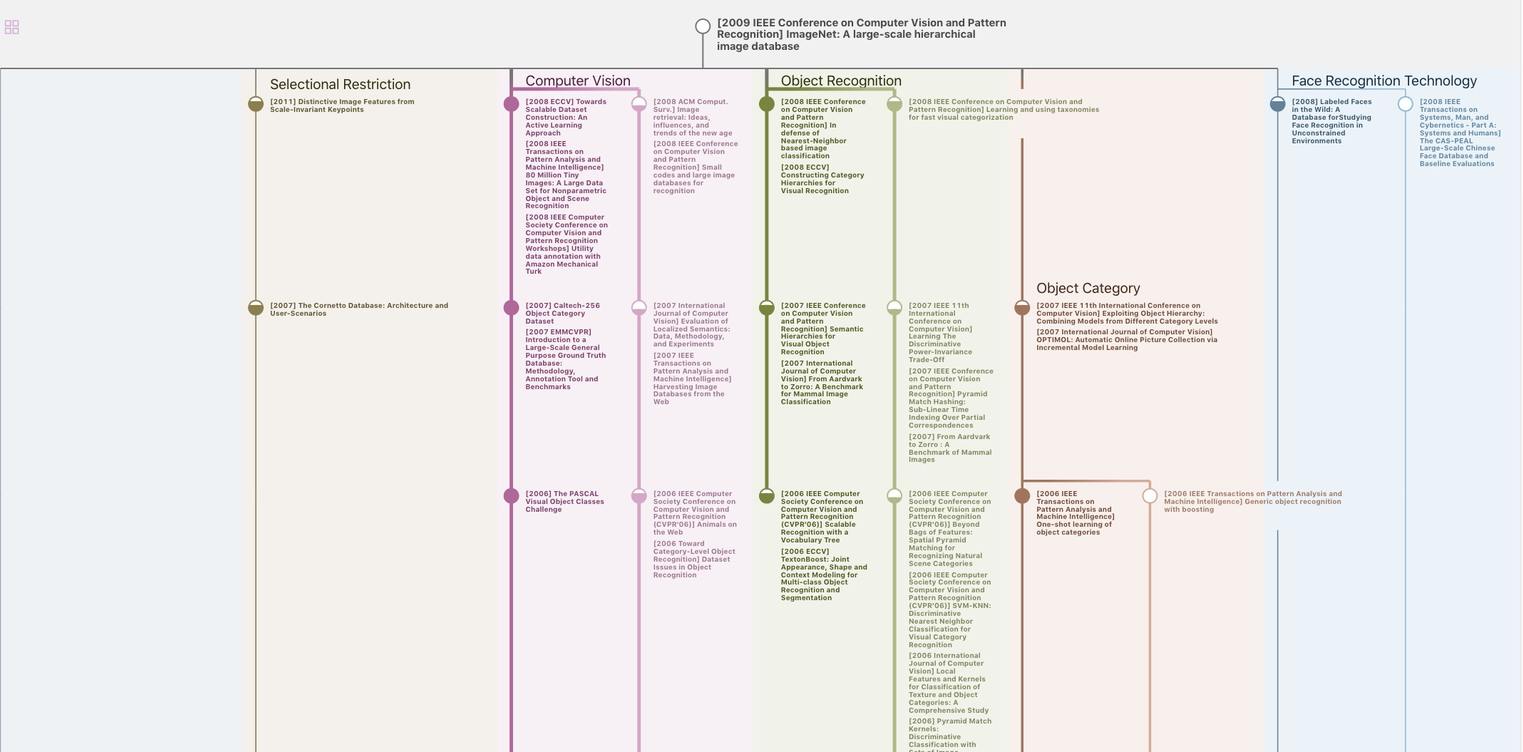
生成溯源树,研究论文发展脉络
Chat Paper
正在生成论文摘要