Weighted Feature Fusion of Convolutional Neural Network and Graph Attention Network for Hyperspectral Image Classification
IEEE TRANSACTIONS ON IMAGE PROCESSING(2022)
摘要
Convolutional Neural Networks (CNN) and Graph Neural Networks (GNN), such as Graph Attention Networks (GAT), are two classic neural network models, which are applied to the processing of grid data and graph data respectively. They have achieved outstanding performance in hyperspectral images (HSIs) classification field, which have attracted great interest. However, CNN has been facing the problem of small samples and GNN has to pay a huge computational cost, which restrict the performance of the two models. In this paper, we propose Weighted Feature Fusion of Convolutional Neural Network and Graph Attention Network (WFCG) for HSI classification, by using the characteristics of superpixel-based GAT and pixel-based CNN, which proved to be complementary. We first establish GAT with the help of superpixel-based encoder and decoder modules. Then we combined the attention mechanism to construct CNN. Finally, the features are weighted fusion with the characteristics of two neural network models. Rigorous experiments on three real-world HSI data sets show WFCG can fully explore the high-dimensional feature of HSI, and obtain competitive results compared to other state-of-the art methods.
更多查看译文
关键词
Feature extraction, Convolutional neural networks, Training, Hyperspectral imaging, Data mining, Decoding, Data models, Hyperspectral image classification, convolutional neural network, graph attention network, weighted feature fusion, attention mechanism
AI 理解论文
溯源树
样例
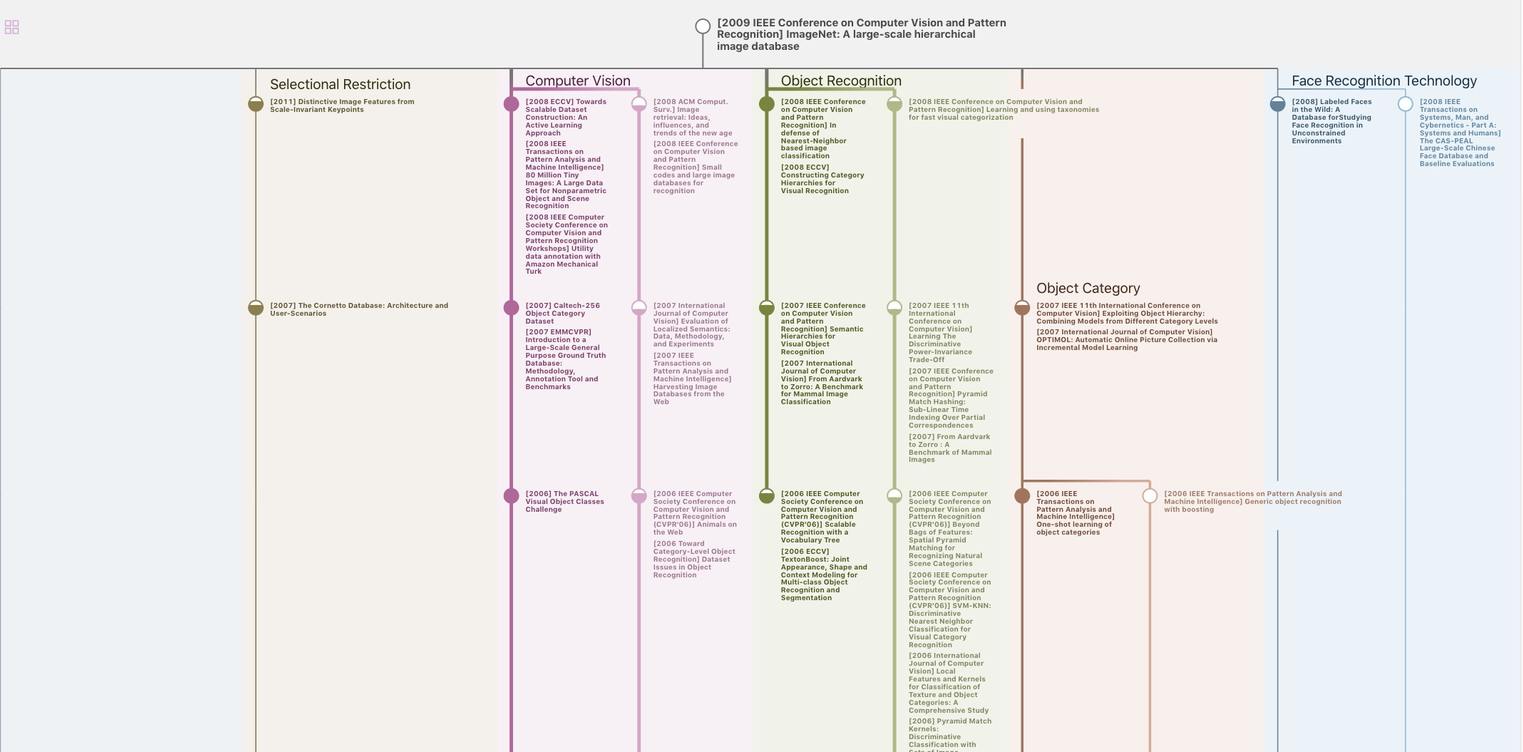
生成溯源树,研究论文发展脉络
Chat Paper
正在生成论文摘要