Causality and Correlation Graph Modeling for Effective and Explainable Session-Based Recommendation
ACM TRANSACTIONS ON THE WEB(2024)
摘要
Session-based recommendation, which has witnessed a booming interest recently, focuses on predicting a user's next interested item(s) based on an anonymous session. Most existing studies adopt complex deep learning techniques (e.g., graph neural networks) for effective session-based recommendation. However, they merely address co-occurrence between items, but fail to distinguish a causality and correlation relationship. Considering the varied interpretations and characteristics of causality and correlation relationships between items, in this study, we propose a novel method denoted as CGSR by jointly modeling causality and correlation relationships between items. In particular, we construct cause, effect, and correlation graphs from sessions by simultaneously considering the false causality problem. We further design a graph neural network-based method for session-based recommendation. To conclude, we strive to explore the relationship between items from specific "causality" (directed) and "correlation" (undirected) perspectives. Extensive experiments on three datasets show that our model outperforms other state-of-the-art methods in terms of recommendation accuracy. Moreover, we further propose an explainable framework on CGSR and demonstrate the explainability of our model via case studies on an Amazon dataset.
更多查看译文
关键词
Session-based recommendation,graph neural network,product relationship
AI 理解论文
溯源树
样例
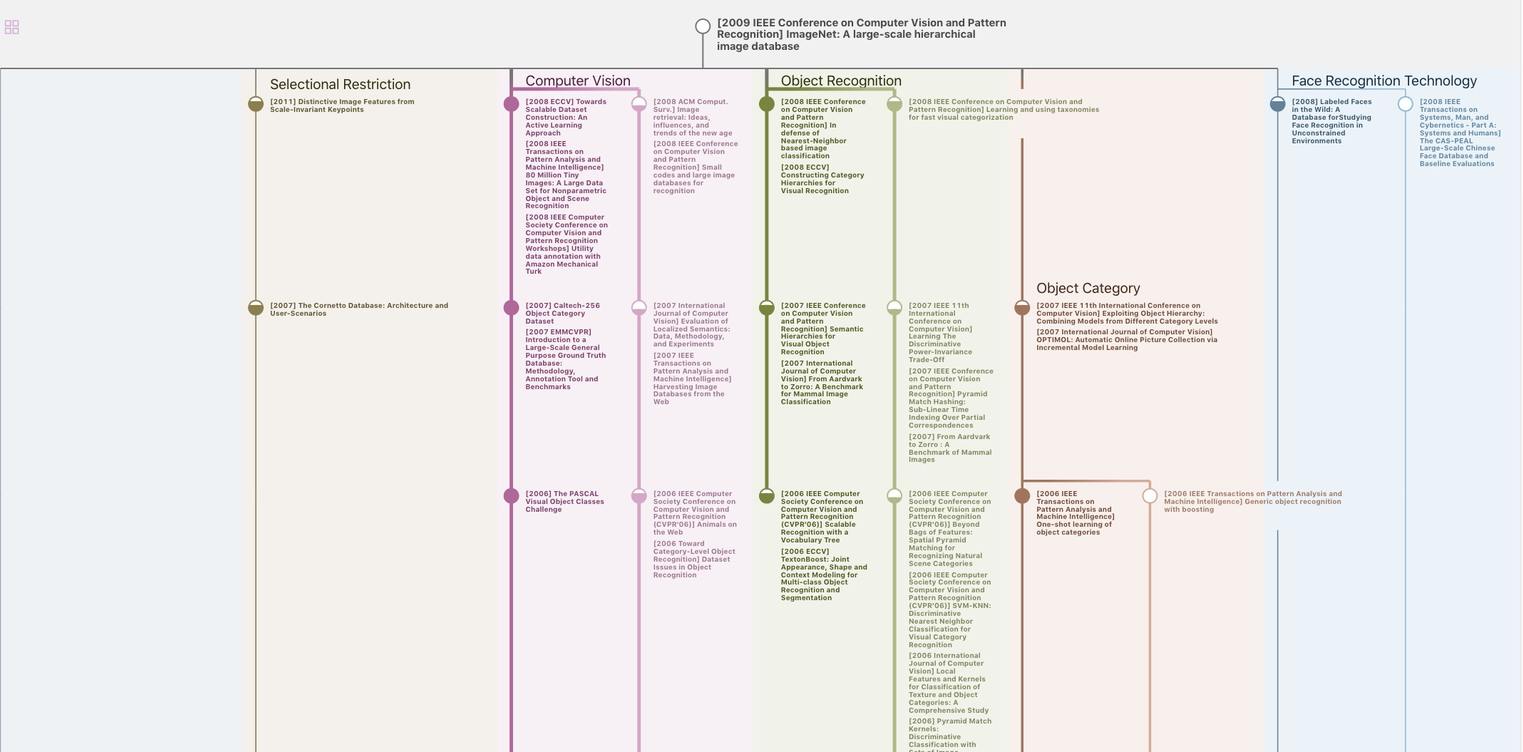
生成溯源树,研究论文发展脉络
Chat Paper
正在生成论文摘要