Neural Grapheme-To-Phoneme Conversion with Pre-Trained Grapheme Models
IEEE International Conference on Acoustics, Speech, and Signal Processing (ICASSP)(2022)
摘要
Neural network models have achieved state-of-the-art performance on grapheme-to-phoneme (G2P) conversion. However, their performance relies on large-scale pronunciation dictionaries, which may not be available for a lot of languages. Inspired by the success of the pre-trained language model BERT, this paper proposes a pre-trained grapheme model called grapheme BERT (GBERT), which is built by self-supervised training on a large, language-specific word list with only grapheme information. Furthermore, two approaches are developed to incorporate GBERT into the state-of-the-art Transformer-based G2P model, i.e., fine-tuning GBERT or fusing GBERT into the Transformer model by attention. Experimental results on the Dutch, Serbo-Croatian, Bulgarian and Korean datasets of the SIGMORPHON 2021 G2P task confirm the effectiveness of our GBERT-based G2P models under both medium-resource and low-resource data conditions.
更多查看译文
关键词
grapheme-to-phoneme conversion,pre-trained grapheme model,self-supervised training,Transformer
AI 理解论文
溯源树
样例
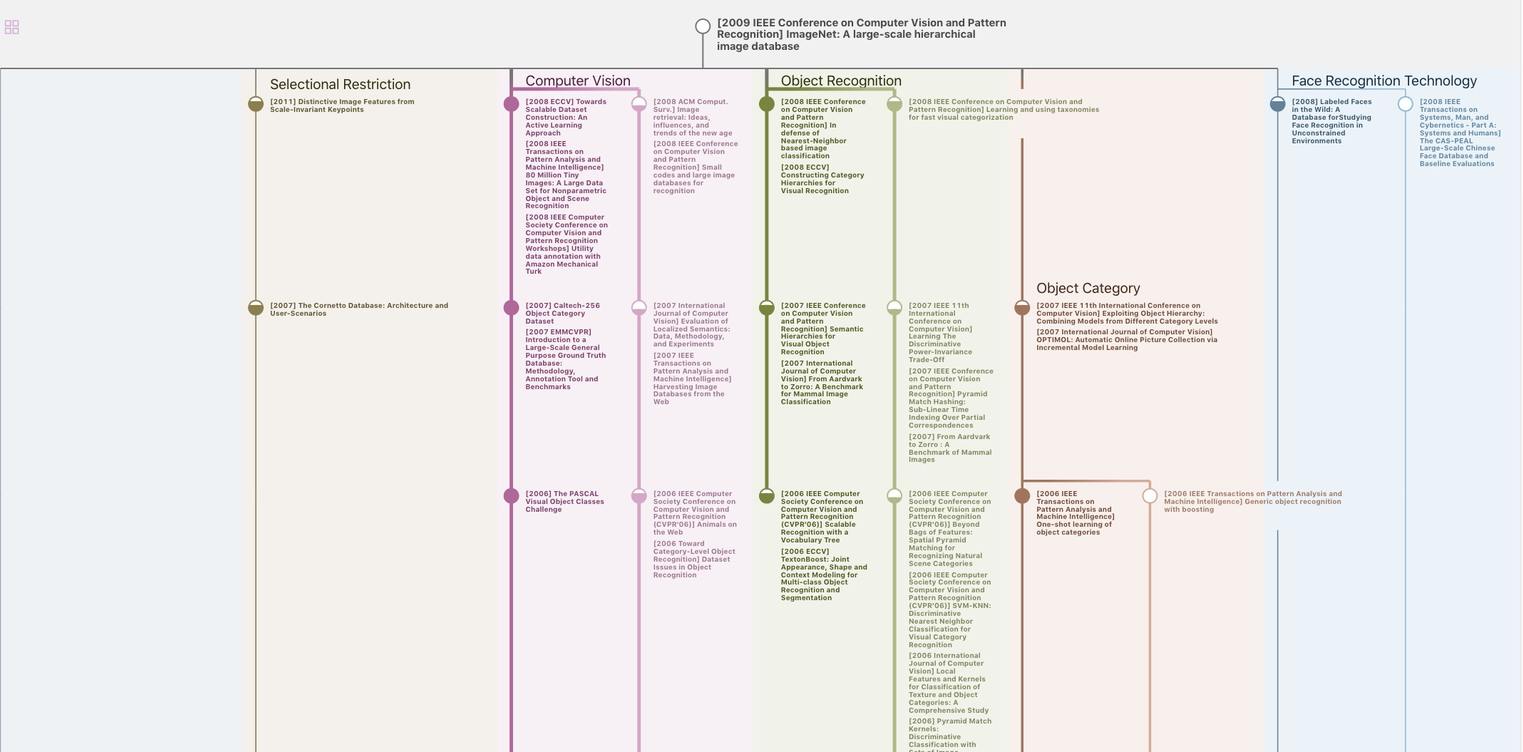
生成溯源树,研究论文发展脉络
Chat Paper
正在生成论文摘要