Computed tomography-based deep-learning prediction of induction chemotherapy treatment response in locally advanced nasopharyngeal carcinoma
STRAHLENTHERAPIE UND ONKOLOGIE(2021)
摘要
Background Deep learning methods have great potential to predict treatment response. The objective of this study was to evaluate and validate the predictive performance of the computed tomography (CT)-based model using deep learning features for identification of responders and nonresponders to induction chemotherapy (IC) in nasopharyngeal carcinoma (NPC). Materials and methods All eligible patients were included retrospectively between January 2012 and December 2018, and assigned to the training ( n = 208) or the testing cohort ( n = 89). We extracted deep learning features of six pretrained convolutional neural networks (CNNs) via transfer learning method, and handcrafted radiomics features manually. Support vector machine (SVM) was adopted as the classifier. All predictive models were evaluated using the area under the receiver operating characteristics curve (AUC), by which an optimal model was selected. We also built clinical and clinical–radiological models for comparison. Results The model with features extracted from ResNet50 (RN-SVM) had optimal performance among all models with features extracted from pretrained CNNs with an AUC of 0.811, accuracy of 68.54%, sensitivity of 61.54%, specificity of 87.50%, positive predictive value (PPV) of 93.02%, and negative predictive value (NPV) of 45.65% in the testing cohort. The handcrafted radiomics model was slightly inferior to the RN-SVM model with an AUC of 0.663 and accuracy of 60.67% in the testing cohort. All the imaging-derived models had better predictive performance than the clinical model. Conclusion The noninvasive deep learning method could provide efficient prediction of treatment response to IC in locally advanced NPC and might be a practicable approach in therapeutic strategy decision-making.
更多查看译文
关键词
Nasopharyngeal carcinoma,Convolutional neural network,Transfer learning,Predictor,CT
AI 理解论文
溯源树
样例
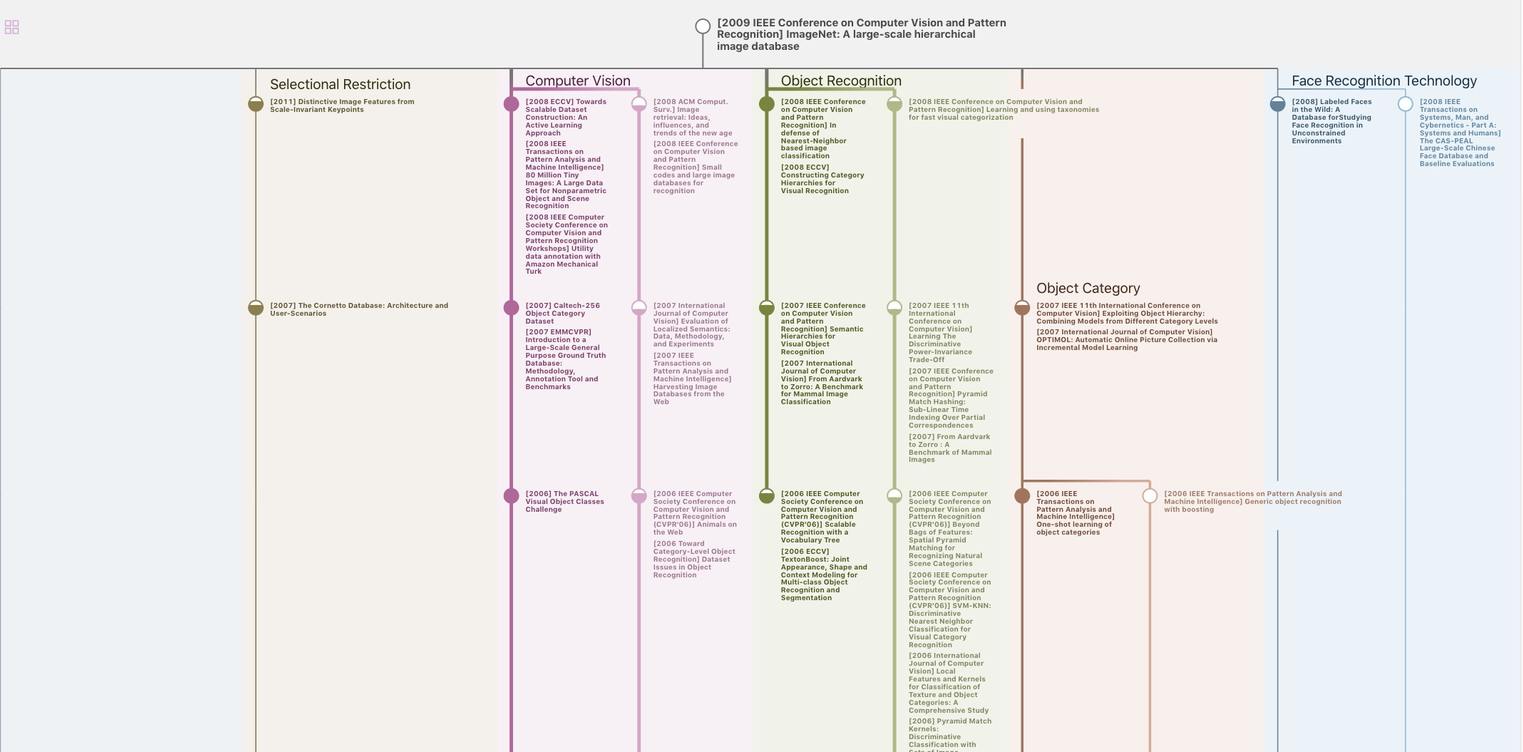
生成溯源树,研究论文发展脉络
Chat Paper
正在生成论文摘要