Sleep Stage Classification Using Non-Invasive Bed Sensing and Deep Learning.
BIBM(2021)
摘要
Sleep stage classification can be used to monitor sleep quality and diagnose sleep disorders. Sleep disorders can be correlated to health conditions such as Alzheimer’s and Parkinson’s disease. This project uses a hydraulic bed sensor positioned under the mattress, as well as a deep learning approach, for sleep stage classification. Our motivation is to provide an automatic, non-invasive and more accessible method of classifying sleep stages by using deep learning to analyze data gathered from the hydraulic bed sensor. The test subjects for this project were elderly patients with sleep disorders. Polysomnography (PSG) data, the current gold standard, was also collected in a Sleep Lab to serve as the ground truth for the bed sensor data. In this study, sleep stages are categorized into 3 categories: Wake, Rapid Eye Movement (REM), and Non-Rapid Eye Movement (NREM). This paper uses a Convolutional Neural Network (CNN)-Long-Short Term Memory (LSTM) hybrid model with 2 CNNs of different filter sizes for feature extraction. These features are then fed into the LSTM for classification. Our results show an average accuracy of about 76% using the leave-one-subject-out (LOSO) validation. These results are promising and show that the hydraulic bed sensor combined with a deep learning approach is capable of providing an automatic and non-invasive method of classifying sleep stages.
更多查看译文
关键词
classification,sleep stages,in-home monitoring,deep learning
AI 理解论文
溯源树
样例
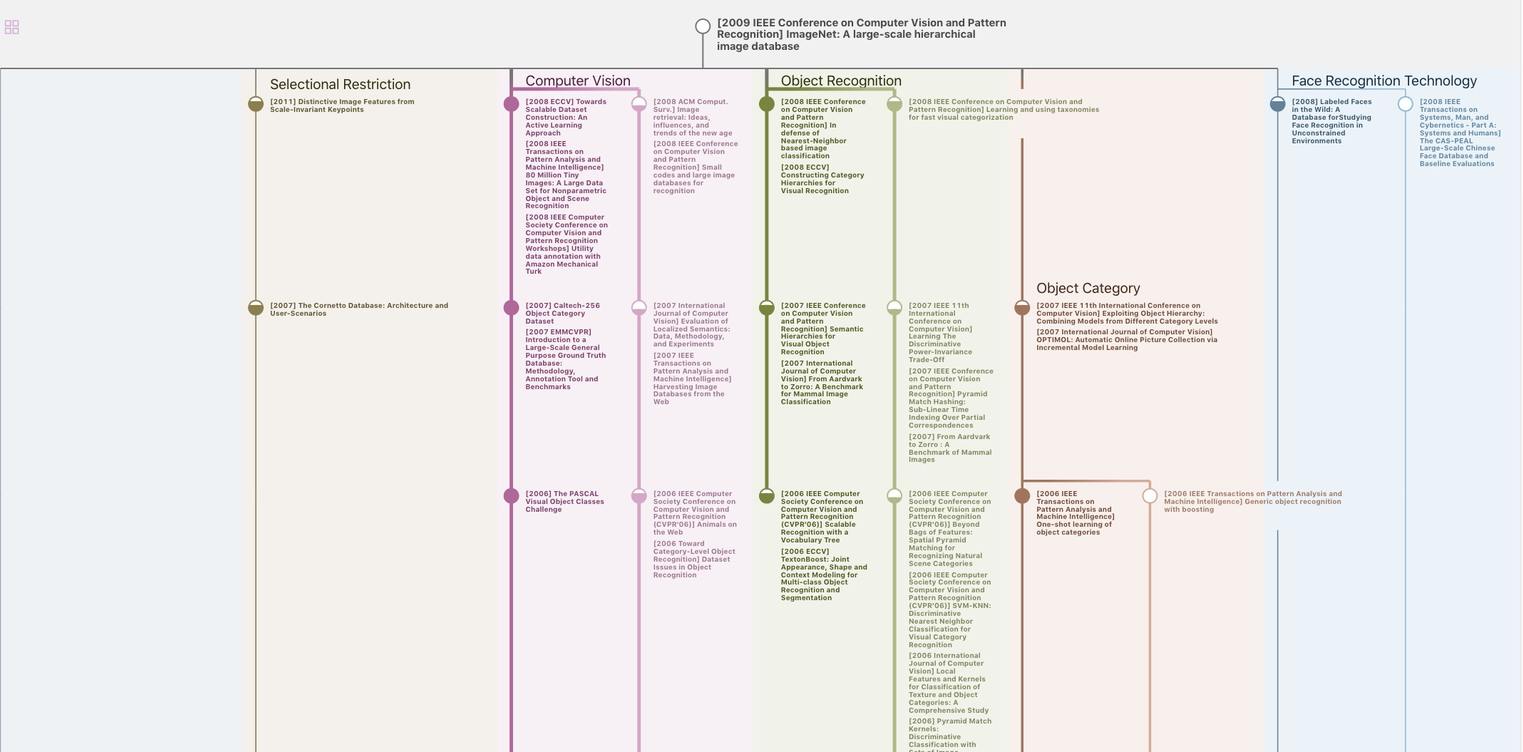
生成溯源树,研究论文发展脉络
Chat Paper
正在生成论文摘要