A machine learning approach for medical device classification.
ICEGOV(2021)
摘要
The growth of medical device innovation over the last decades has necessitated the need for strong regulatory control in order to ensure the safety and performance of such devices. Medical devices are categorised according to the risk posed to the public. However, the legislation describing the classification rules are often dense and difficult to read. In order to facilitate device classification, the medical device regulator in Australia, the Therapeutic Goods Authority (TGA), provides online digital support tool for device classification. In this work, we (i) evaluate the online tool and (ii) make a further a proposal for using machine learning as means to provide more effective results. For the first part of this work, we asses whether the tool increases the readability of the legislative rules by evaluating the Flesch reading ease score of the legislation and the tool. While the online tool provides some degree of simplicity and readability over the legislation, we argue that the TGA can make more use of its data in order to provide more effective services. In the second part, we develop a proof-of-concept machine learning model to classify a device based on its stated purpose. The results of the experiment show a 82% weighted accuracy across four class labels, indicating that a more data-driven approach could be adopted by the authority.
更多查看译文
AI 理解论文
溯源树
样例
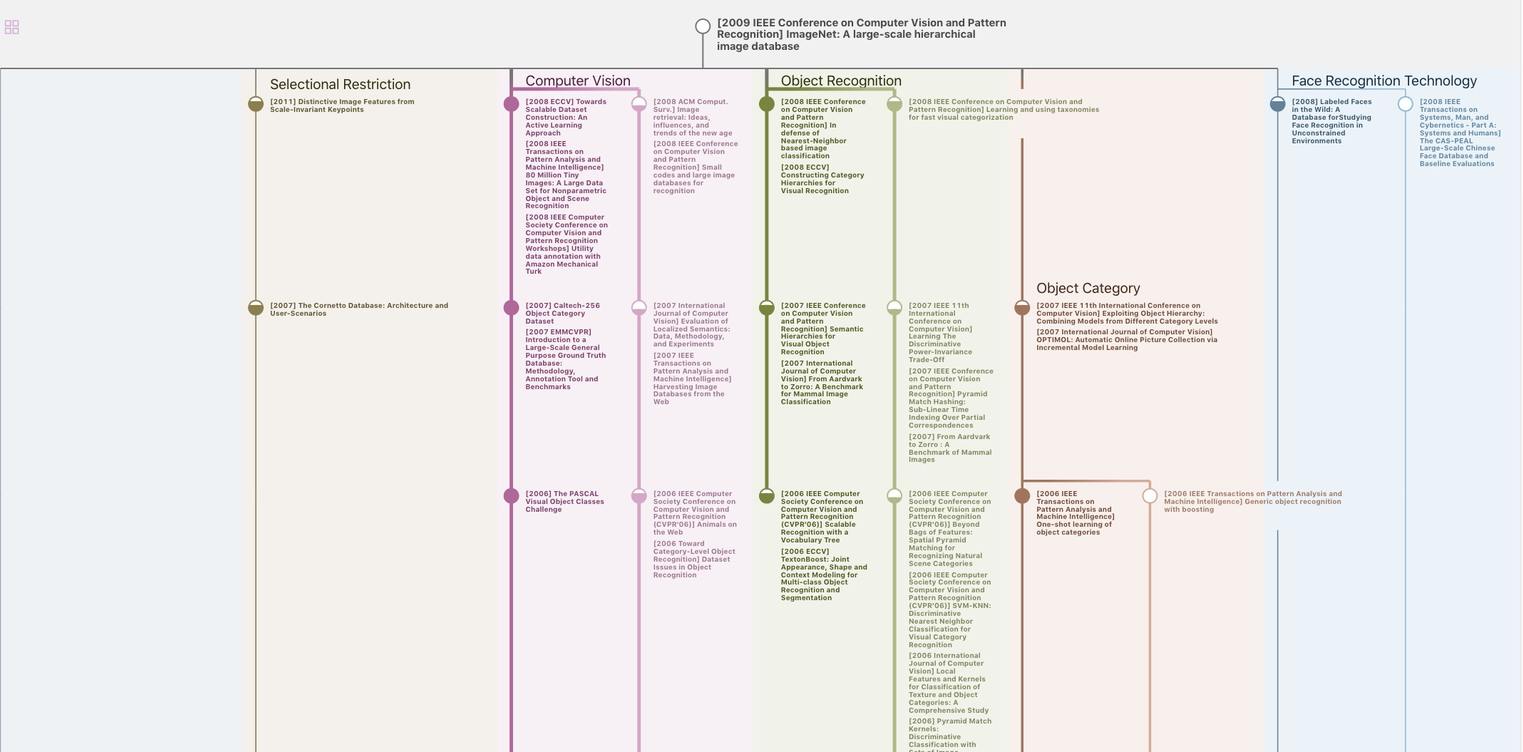
生成溯源树,研究论文发展脉络
Chat Paper
正在生成论文摘要