An Evaluation of Segmentation Techniques for Covid-19 Identification in Chest X-Ray.
CIARP(2021)
摘要
COVID-19 is a highly contagious disease caused by the SARS-CoV-2 virus. Due to its high impact on society, several efforts have been made to design practical ways to support COVID-19 diagnosis. In this context, automated solutions based on chest x-rays (CXR) images and deep learning are among the popular ones. Although these techniques achieved exciting results in the literature, the use of regions that do not support pneumonia diagnosis, i.e., regions outside the lung area, may bias the recognition model. A strategy to avoid this issue is to use segmentation techniques to isolate the lung area before the classification process. In this work, we investigate the impact of three CNN segmentation architectures on COVID-19 identification: U-Net, MultiResUnet, and BCDU-NET. We also investigate which portions of the CXR most influence each model’s predictions, using Explainable Artificial Intelligence. The BCDU-NET architecture achieved a Jaccard Index of 0.91 and a Dice Coefficient of 0.95. In the best scenario, lung segmentation improved the COVID-19 identification F1-Score by about 6.6%.
更多查看译文
关键词
segmentation techniques,chest,x-ray
AI 理解论文
溯源树
样例
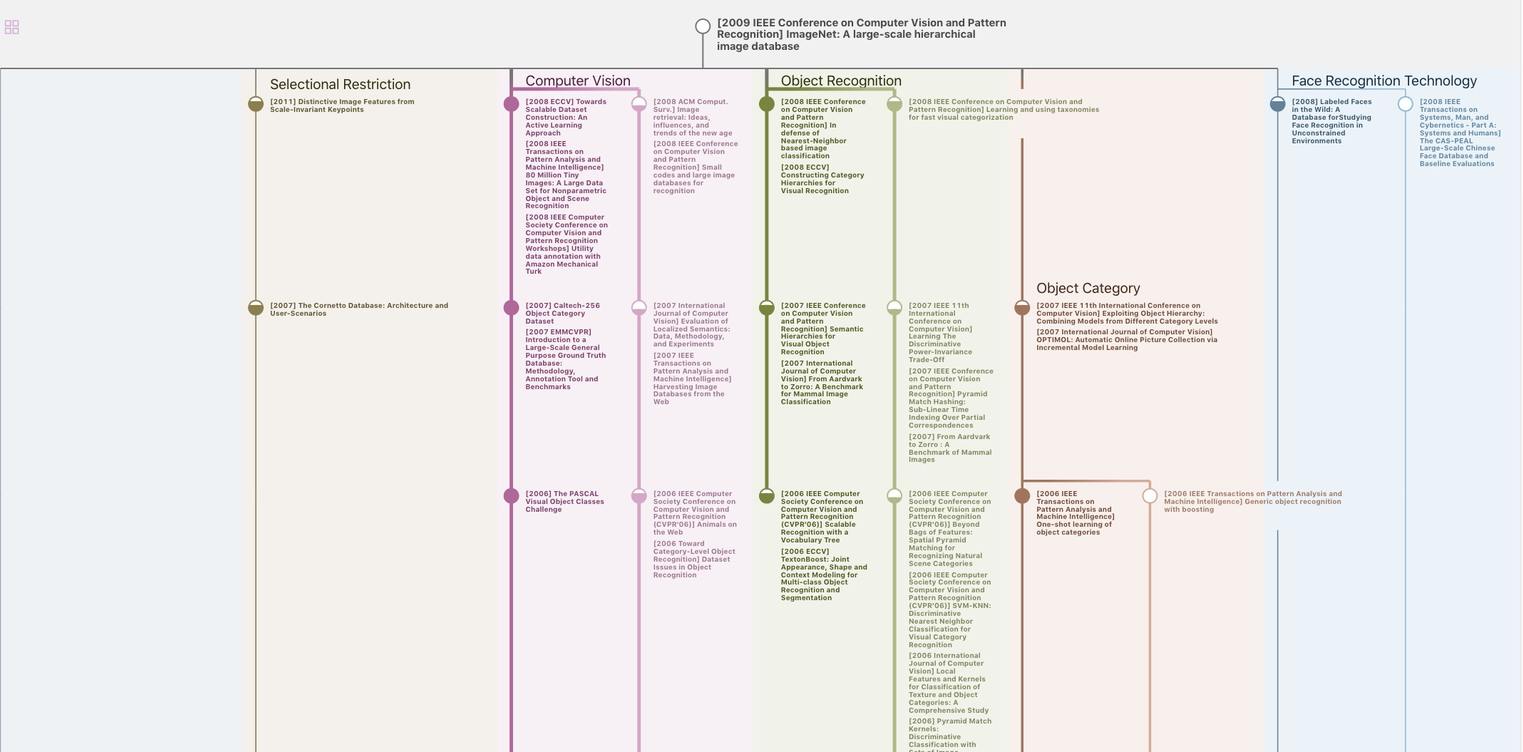
生成溯源树,研究论文发展脉络
Chat Paper
正在生成论文摘要