Language-Agnostic and Language-Aware Multilingual Natural Language Understanding for Large-Scale Intelligent Voice Assistant Application.
IEEE BigData(2021)
摘要
Natural language understanding (NLU) is one of the most critical components in goal-oriented dialog systems and enables innovative Big Data applications such as intelligent voice assistants (IVA) and chatbots. While recent advances in deep learning-based NLU models have achieved significant improvements in terms of accuracy, most existing works are monolingual or bilingual. In this work, we propose and experiment with techniques to develop multilingual NLU models. In particular, we first p ropose a p urely l anguage-agnostic m ultilingual NLU framework using a multilingual BERT (mBERT) encoder, a joint decoder design for intent classification a nd s lot filling tasks, and a novel co-appearance regularization technique. Then three distinct language-aware multilingual NLU approaches are proposed including using language code as explicit input; using language-specific parameters during decoding; and using implicit language identification a s an a uxiliary t ask. We show results for a large-scale, commercial IVA system trained on a various set of intents with huge vocabulary sizes, as well as on a public multilingual NLU dataset. We performed experiments in explicit consideration of code-mixing and language dissimilarities which are practical concerns in large-scale real-world IVA systems. We have found that language-aware designs can improve NLU performance when language dissimilarity and code-mixing exist. The empirical results together with our proposed architectures provide important insights towards designing multilingual NLU systems.
更多查看译文
关键词
natural language understanding,multilingual representation,intelligent voice assistant
AI 理解论文
溯源树
样例
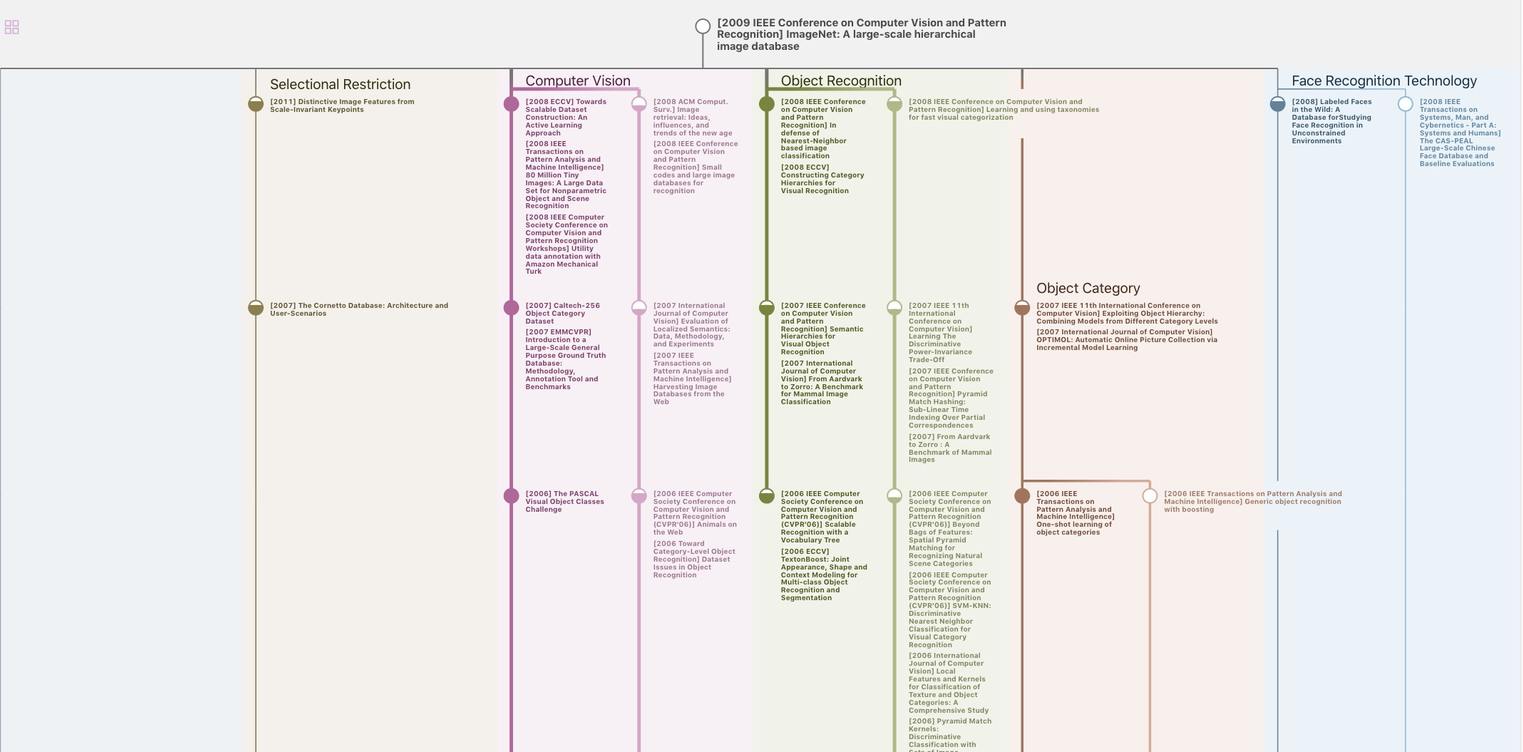
生成溯源树,研究论文发展脉络
Chat Paper
正在生成论文摘要