Time Series Representation Learning with Contrastive Triplet Selection.
COMAD/CODS(2022)
摘要
Representation learning, with its proven appeal and advantage in visual and textual modalities, has seen extensions to numerical time series. Recent work proposed a triplet loss formulation based on random triplet sampling to derive a fixed length embedding for time series. Unlike images and text however, statistical and distance measures can be readily computed from numerical time series data to quantitatively contrast differences or cluster based on similarity. This paper investigates the triplet mining problem through contrastive identification methods to select anchors, positive and negative samples in the raw signal space as well as in the embedded vector space. Selected triplets are then learned by a causal temporal neural network to minimize anchor’s distance to positives and maximize its distance to negatives. Experimental results along with an ablation study to compare these methods measured in classification accuracy and variance demonstrated notable improvement over random triplet selection. We also investigate and report performance improvement when sampling avoids label contamination, demonstrating advantage of algorithms proposed.
更多查看译文
AI 理解论文
溯源树
样例
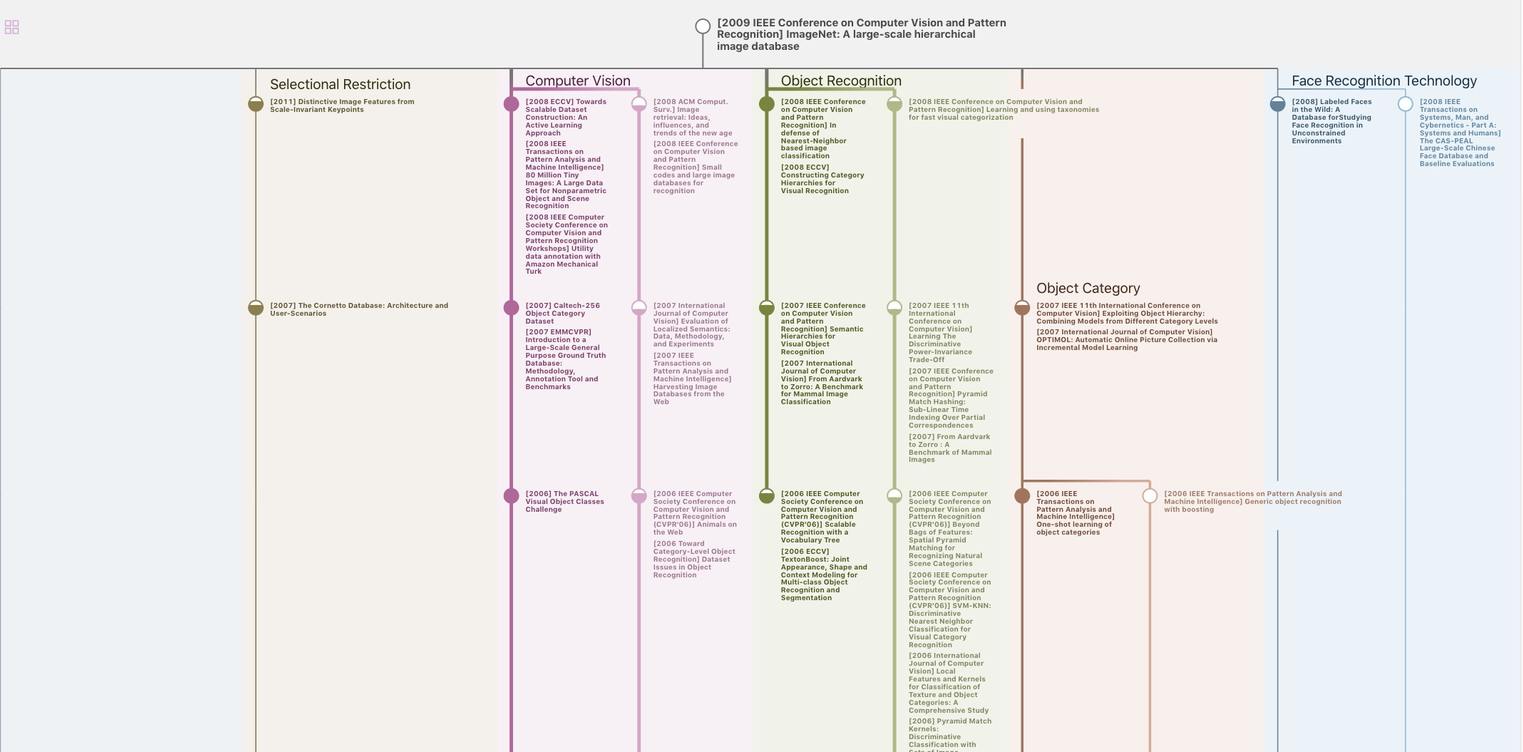
生成溯源树,研究论文发展脉络
Chat Paper
正在生成论文摘要