Rumor Detection on Social Media with Out-In-Degree Graph Convolutional Networks.
SMC(2021)
摘要
With the tremendous development in hardware computing and the widespread use of mobile terminal devices, there are increasingly more people who prefer to share their lives and opinions on social media. Though social media platforms allow everyone to express their opinions freely, they create convenience for rumor propagation in the meantime, which brings huge negative influence on the public and makes rumor detection extremely necessary. Currently, the most effective methods regard rumor propagation network as a graph and adopt graph convolutional networks (GCN) to detect rumor automatically. Such methods achieve promising performance in rumor detection, however, we argue that they have two critical defects: 1) they neglect the position contributions of rumor nodes in a graph, reducing the accuracy of rumor detection results; 2) they are inadequate in dealing with imbalanced data, which also indicates the inflexibility and the poor generalization ability of the model. To overcome these issues, we incorporate Katz centrality into spectral-domain graph convolution and propose a novel model named Out-In-Degree Graph Convolutional Networks (OID-GCN). Specifically, besides enhancing accuracy, Katz centrality can efficiently capture the position information of nodes, while the rest structure of OID-GCN shows a superb ability in dealing with imbalanced data. Comprehensive experimental results on two real-world datasets Twitter-15 and Twitter-16 demonstrate our OID-GCN outperforms existing methods.
更多查看译文
关键词
social media,networks,out-in-degree
AI 理解论文
溯源树
样例
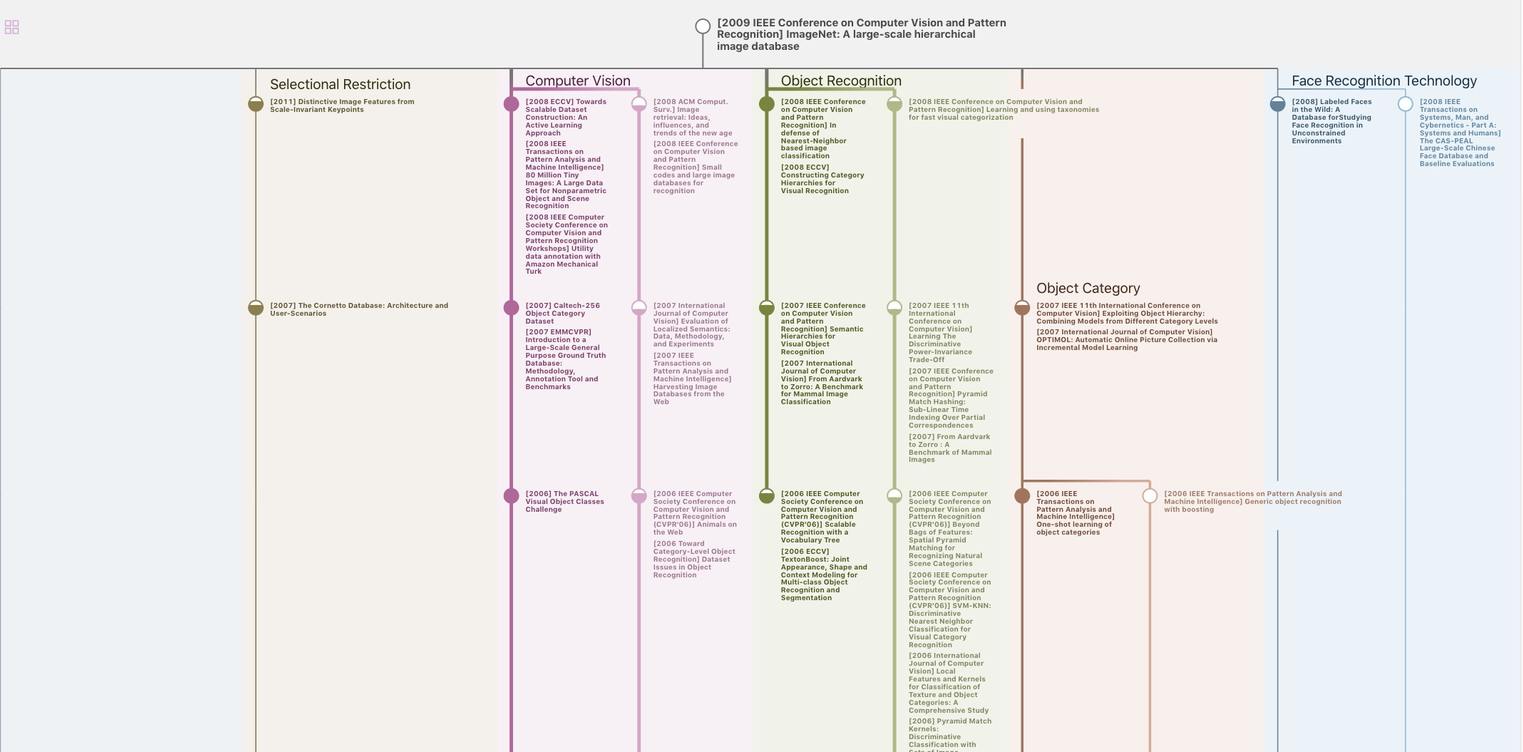
生成溯源树,研究论文发展脉络
Chat Paper
正在生成论文摘要