Evolutionary Multitasking via Artificial Neural Networks.
SMC(2021)
摘要
Evolutionary Multi-Tasking (EMT), which solves multiple optimization tasks simultaneously, is a burgeoning topic in the area of evolutionary computation. As the EMT transfers useful knowledge across tasks to guide the search while the optimization process progresses online, superior search performance has been obtained in many recent attempts. Autoencoding evolutionary multitasking is a recently proposed EMT algorithm, which employs a single-layer denoising autoencoder for knowledge transfer. However, since the autoencoding evolutionary multitasking (AEEMT) algorithm learns the relationships between tasks through a linear auto-encoder, it may lead to negative transfer in cases that the linear variable relationship does not hold across tasks. Taking this cue, we propose an evolutionary multitasking algorithm with artificial neural networks for transferring knowledge across tasks in this paper, which possesses higher modeling capability of variable relationships. To test and verify the efficiency and effectiveness of the proposed model, we conducted comprehensive empirical studies on the well-known single-objectives multi-task optimization benchmarks. The experimental results have shown that the proposed method has a remarkable effect on the evolutionary search in terms of both search speed and solution quality and comparing to the autoencoding evolutionary multitasking algorithms and the single-task evolutionary counterpart.
更多查看译文
关键词
Evolutionary Multi-tasking,Knowledge Transfer,Neural Network,Multi-task Optimization
AI 理解论文
溯源树
样例
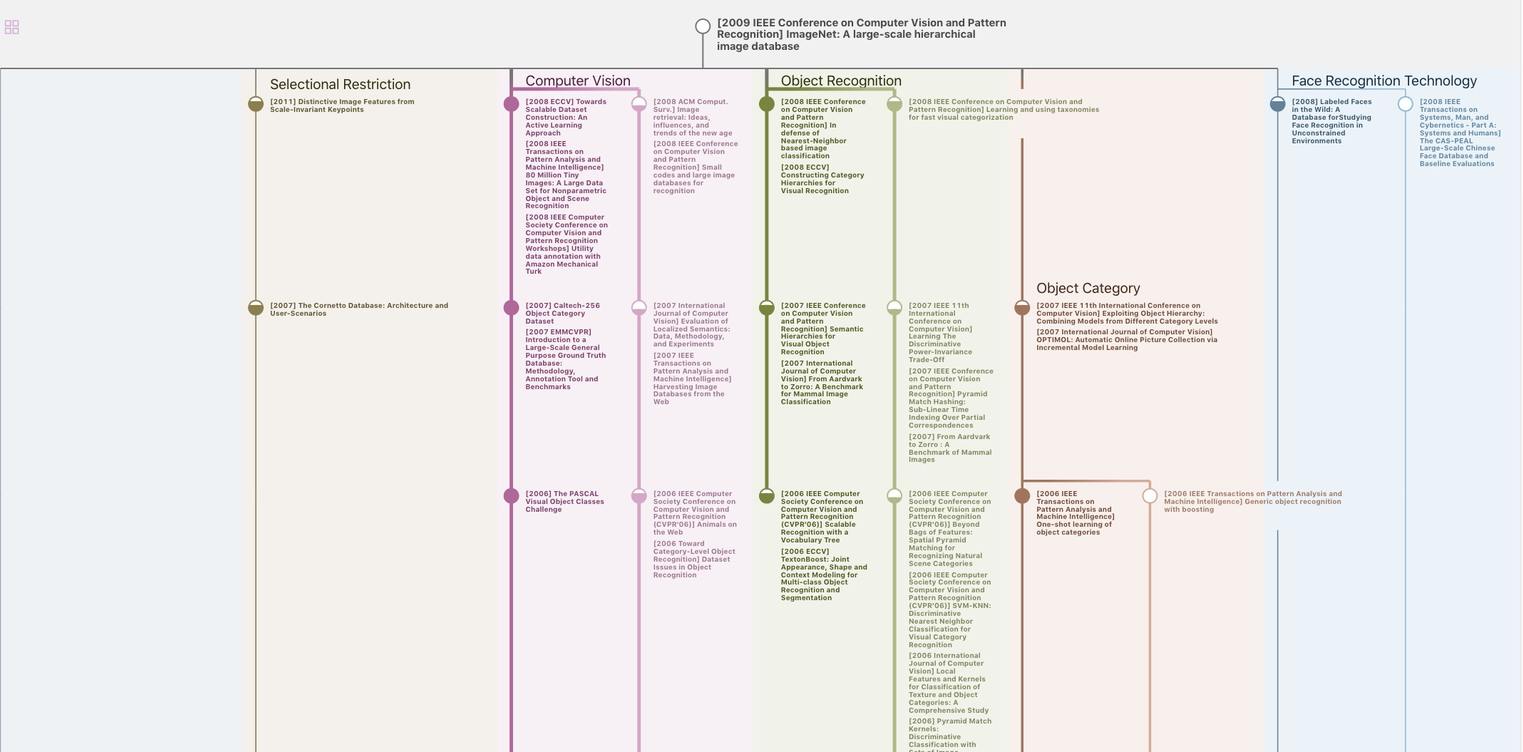
生成溯源树,研究论文发展脉络
Chat Paper
正在生成论文摘要