Federated Learning and Privacy: Building privacy-preserving systems for machine learning and data science on decentralized data
Queue(2021)
摘要
AbstractCentralized data collection can expose individuals to privacy risks and organizations to legal risks if data is not properly managed. Federated learning is a machine learning setting where multiple entities collaborate in solving a machine learning problem, under the coordination of a central server or service provider. Each client's raw data is stored locally and not exchanged or transferred; instead, focused updates intended for immediate aggregation are used to achieve the learning objective. This article provides a brief introduction to key concepts in federated learning and analytics with an emphasis on how privacy technologies may be combined in real-world systems and how their use charts a path toward societal benefit from aggregate statistics in new domains and with minimized risk to individuals and to the organizations who are custodians of the data.
更多查看译文
AI 理解论文
溯源树
样例
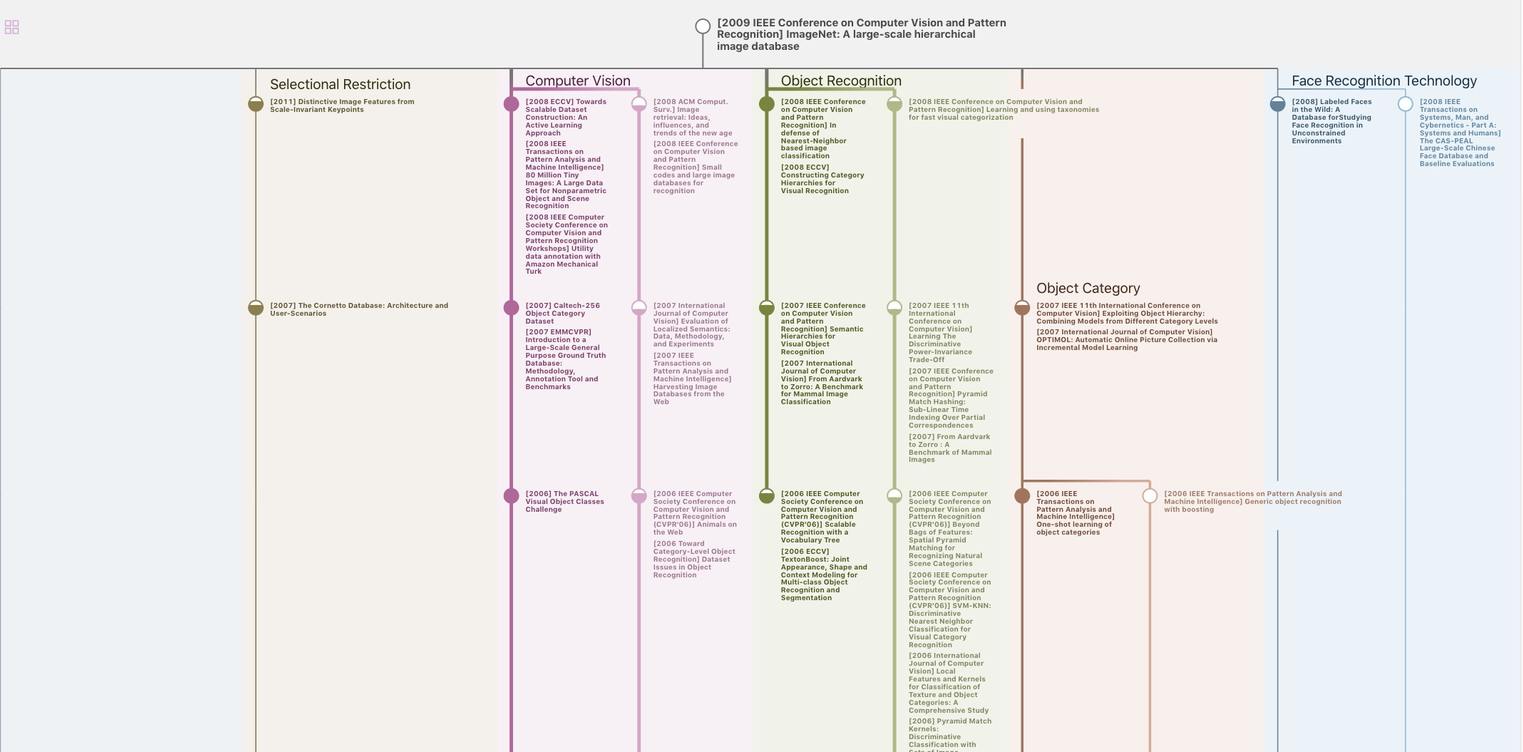
生成溯源树,研究论文发展脉络
Chat Paper
正在生成论文摘要