Mining High Utility Itemsets with Hill Climbing and Simulated Annealing
ACM Transactions on Management Information Systems(2022)
摘要
AbstractHigh utility itemset mining (HUIM) is the task of finding all items set, purchased together, that generate a high profit in a transaction database. In the past, several algorithms have been developed to mine high utility itemsets (HUIs). However, most of them cannot properly handle the exponential search space while finding HUIs when the size of the database and total number of items increases. Recently, evolutionary and heuristic algorithms were designed to mine HUIs, which provided considerable performance improvement. However, they can still have a long runtime and some may miss many HUIs. To address this problem, this article proposes two algorithms for HUIM based on Hill Climbing (HUIM-HC) and Simulated Annealing (HUIM-SA). Both algorithms transform the input database into a bitmap for efficient utility computation and for search space pruning. To improve population diversity, HUIs discovered by evolution are used as target values for the next population instead of keeping the current optimal values in the next population. Through experiments on real-life datasets, it was found that the proposed algorithms are faster than state-of-the-art heuristic and evolutionary HUIM algorithms, that HUIM-SA discovers similar HUIs, and that HUIM-SA evolves linearly with the number of iterations.
更多查看译文
关键词
Hill climbing, simulated annealing, high utility itemsets, bitmap, neighbor
AI 理解论文
溯源树
样例
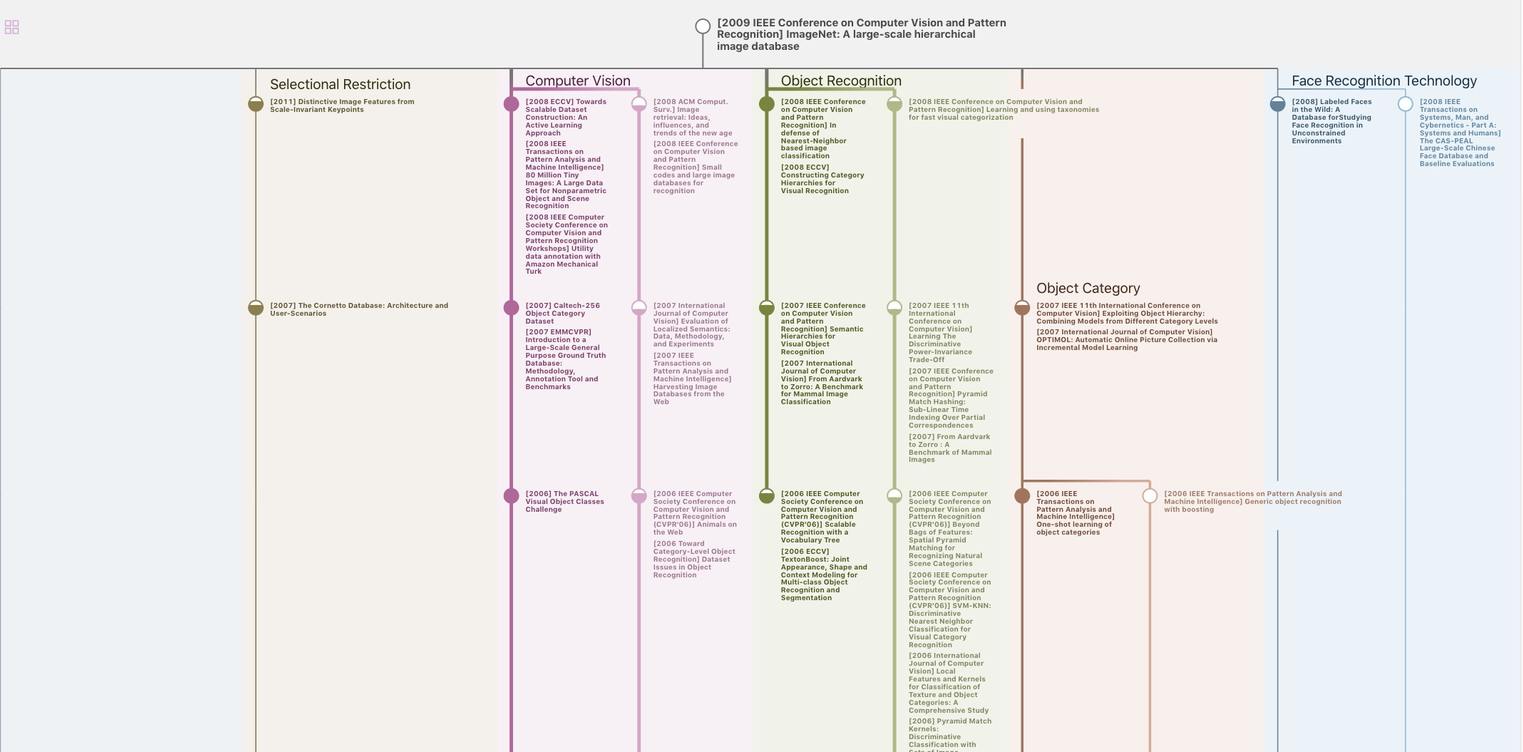
生成溯源树,研究论文发展脉络
Chat Paper
正在生成论文摘要