AFGL-Net: Attentive Fusion of Global and Local Deep Features for Building Facades Parsing
REMOTE SENSING(2021)
摘要
In this paper, we propose a deep learning framework, namely AFGL-Net to achieve building facade parsing, i.e., obtaining the semantics of small components of building facade, such as windows and doors. To this end, we present an autoencoder embedding position and direction encoding for local feature encoding. The autoencoder enhances the local feature aggregation and augments the representation of skeleton features of windows and doors. We also integrate the Transformer into AFGL-Net to infer the geometric shapes and structural arrangements of facade components and capture the global contextual features. These global features can help recognize inapparent windows/doors from the facade points corrupted with noise, outliers, occlusions, and irregularities. The attention-based feature fusion mechanism is finally employed to obtain more informative features by simultaneously considering local geometric details and the global contexts. The proposed AFGL-Net is comprehensively evaluated on Dublin and RueMonge2014 benchmarks, achieving 67.02% and 59.80% mIoU, respectively. We also demonstrate the superiority of the proposed AFGL-Net by comparing with the state-of-the-art methods and various ablation studies.
更多查看译文
关键词
facade parsing, semantic segmentation, MLP, autoencoder, global transformer, attentive feature fusion, Dublin dataset, RueMonge2014 dataset
AI 理解论文
溯源树
样例
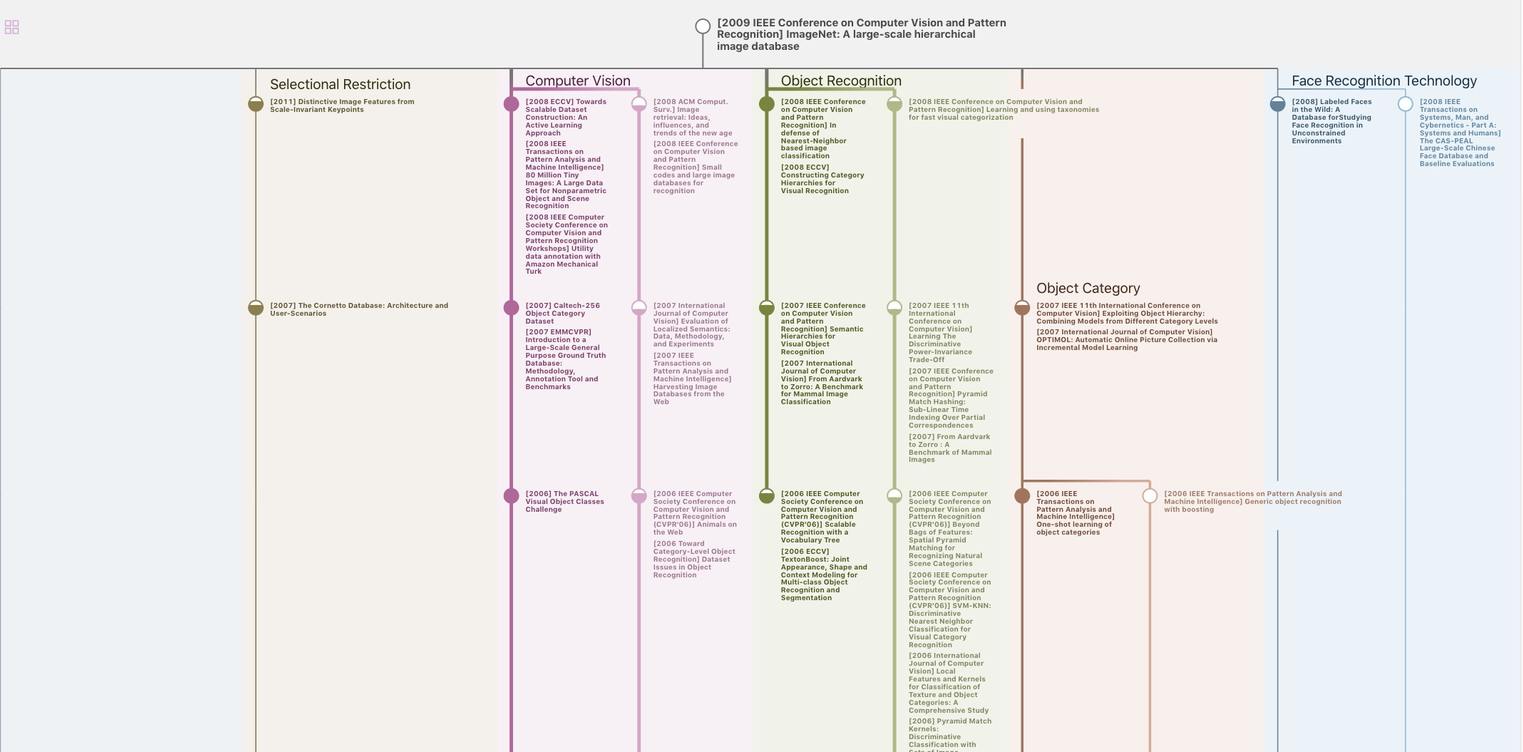
生成溯源树,研究论文发展脉络
Chat Paper
正在生成论文摘要