A Machine Learning Framework for Distributed Functional Compression over Wireless Channels in IoT
arxiv(2022)
摘要
IoT devices generating enormous data and state-of-the-art machine learning techniques together will revolutionize cyber-physical systems. In many diverse fields, from autonomous driving to augmented reality, distributed IoT devices compute specific target functions without simple forms like obstacle detection, object recognition, etc. Traditional cloud-based methods that focus on transferring data to a central location either for training or inference place enormous strain on network resources. To address this, we develop, to the best of our knowledge, the first machine learning framework for distributed functional compression over both the Gaussian Multiple Access Channel (GMAC) and orthogonal AWGN channels. Due to the Kolmogorov-Arnold representation theorem, our machine learning framework can, by design, compute any arbitrary function for the desired functional compression task in IoT. Importantly the raw sensory data are never transferred to a central node for training or inference, thus reducing communication. For these algorithms, we provide theoretical convergence guarantees and upper bounds on communication. Our simulations show that the learned encoders and decoders for functional compression perform significantly better than traditional approaches, are robust to channel condition changes and sensor outages. Compared to the cloud-based scenario, our algorithms reduce channel use by two orders of magnitude.
更多查看译文
关键词
distributed functional compression,iot,wireless channels
AI 理解论文
溯源树
样例
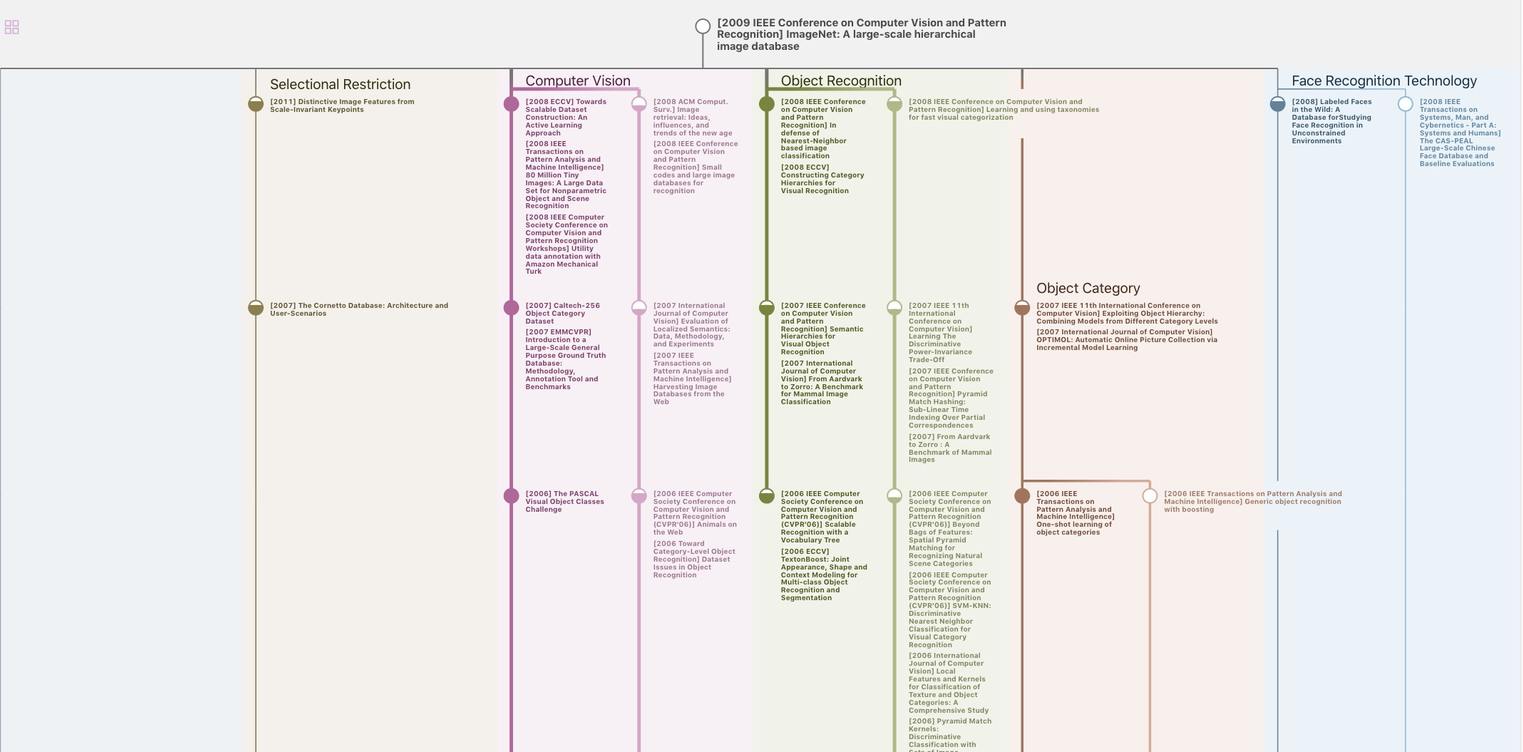
生成溯源树,研究论文发展脉络
Chat Paper
正在生成论文摘要