Adaptive Data Analysis with Correlated Observations.
International Conference on Machine Learning(2022)
摘要
The vast majority of the work on adaptive data analysis focuses on the case where the samples in the dataset are independent. Several approaches and tools have been successfully applied in this context, such as differential privacy, max-information, compression arguments, and more. The situation is far less well-understood without the independence assumption. We embark on a systematic study of the possibilities of adaptive data analysis with correlated observations. First, we show that, in some cases, differential privacy guarantees generalization even when there are dependencies within the sample, which we quantify using a notion we call Gibbs-dependence. We complement this result with a tight negative example. % Second, we show that the connection between transcript-compression and adaptive data analysis can be extended to the non-iid setting.
更多查看译文
关键词
observations,data,analysis
AI 理解论文
溯源树
样例
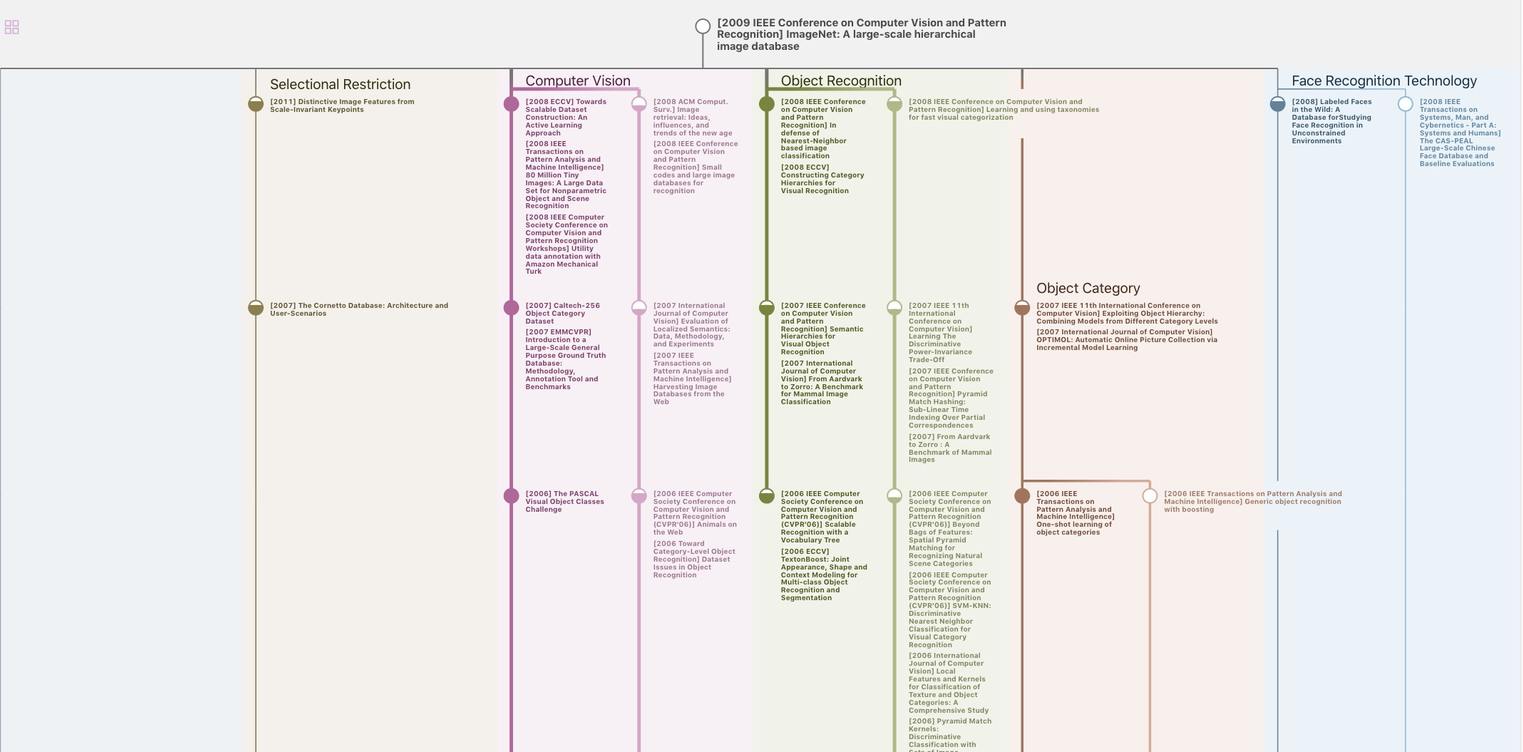
生成溯源树,研究论文发展脉络
Chat Paper
正在生成论文摘要