Bayesian nonparametric mixtures of Exponential Random Graph Models for ensembles of networks
arxiv(2023)
摘要
Ensembles of networks arise in various fields where multiple independent networks are observed, for example, a collection of student networks from different classes. However, there are few models that describe both the variations and characteristics of networks in an ensemble at the same time. In this manuscript, we propose to model ensembles of networks using a Dirichlet Process Mixture of Exponential Random Graph Models (DPM-ERGMs), which divides an ensemble into different clusters and models each cluster of networks using a separate Exponential Random Graph Model (ERGM). By employing a Dirichlet process mixture, the number of clusters can be determined automatically and changed adaptively with the data provided. Moreover, in order to perform full Bayesian inference for DPM-ERGMs, we develop a Metropolis-within-slice sampling algorithm to address the problem of sampling from the intractable ERGMs on an infinite sample space. We also demonstrate the performance of DPM-ERGMs with both simulated and real datasets.
更多查看译文
关键词
Network clustering,Dirichlet process,Markov Chain Monte Carlo,Importance sampling,Adjusted pseudo likelihood
AI 理解论文
溯源树
样例
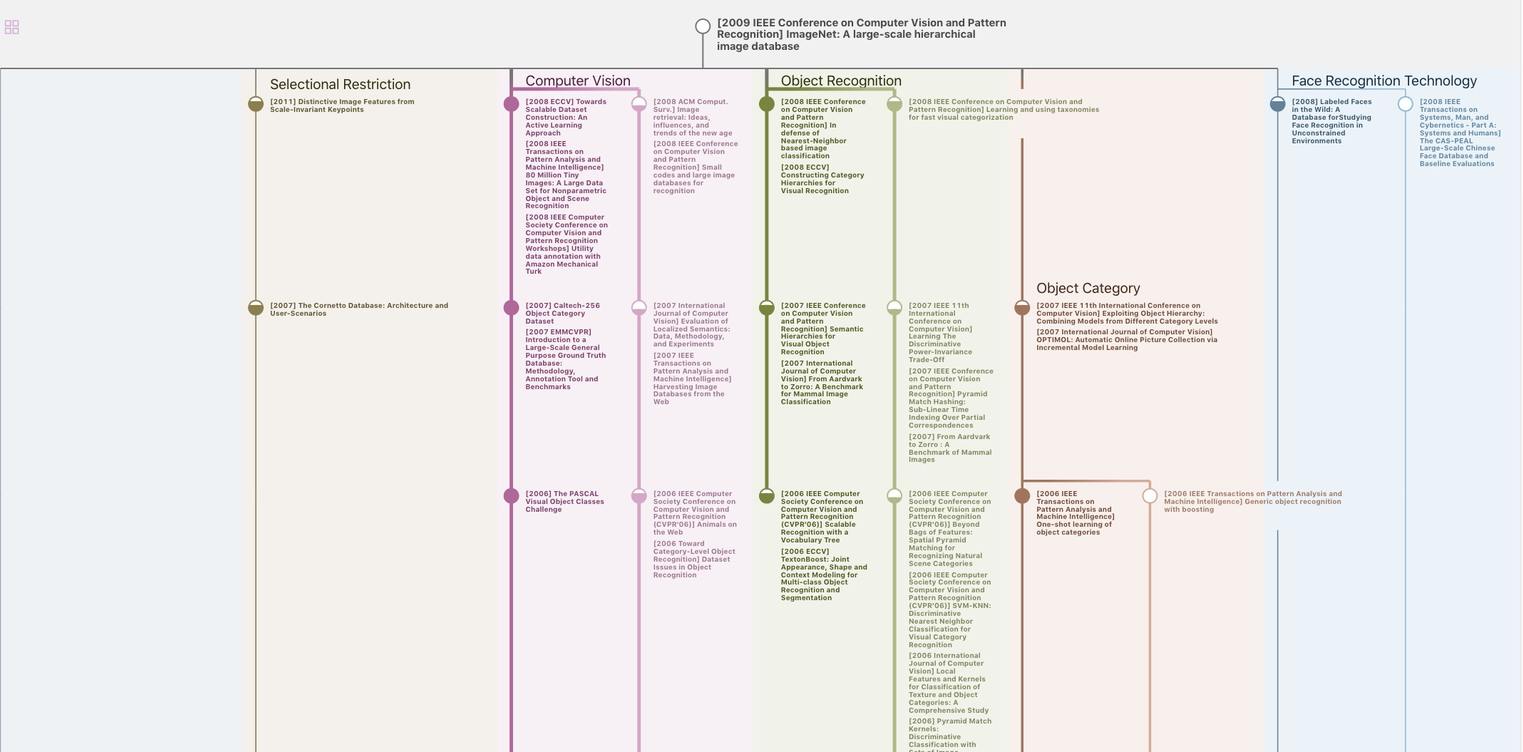
生成溯源树,研究论文发展脉络
Chat Paper
正在生成论文摘要