PDE-Based Optimal Strategy for Unconstrained Online Learning.
International Conference on Machine Learning(2022)
摘要
Unconstrained Online Linear Optimization (OLO) is a practical problem setting to study the training of machine learning models. Existing works proposed a number of potential-based algorithms, but in general the design of these potential functions relies heavily on guessing. To streamline this workflow, we present a framework that generates new potential functions by solving a Partial Differential Equation (PDE). Specifically, when losses are 1-Lipschitz, our framework produces a novel algorithm with anytime regret bound $C\sqrt{T}+||u||\sqrt{2T}[\sqrt{\log(1+||u||/C)}+2]$, where $C$ is a user-specified constant and $u$ is any comparator unknown and unbounded a priori. Such a bound attains an optimal loss-regret trade-off without the impractical doubling trick. Moreover, a matching lower bound shows that the leading order term, including the constant multiplier $\sqrt{2}$, is tight. To our knowledge, the proposed algorithm is the first to achieve such optimalities.
更多查看译文
关键词
online learning,optimal strategy,pde-based
AI 理解论文
溯源树
样例
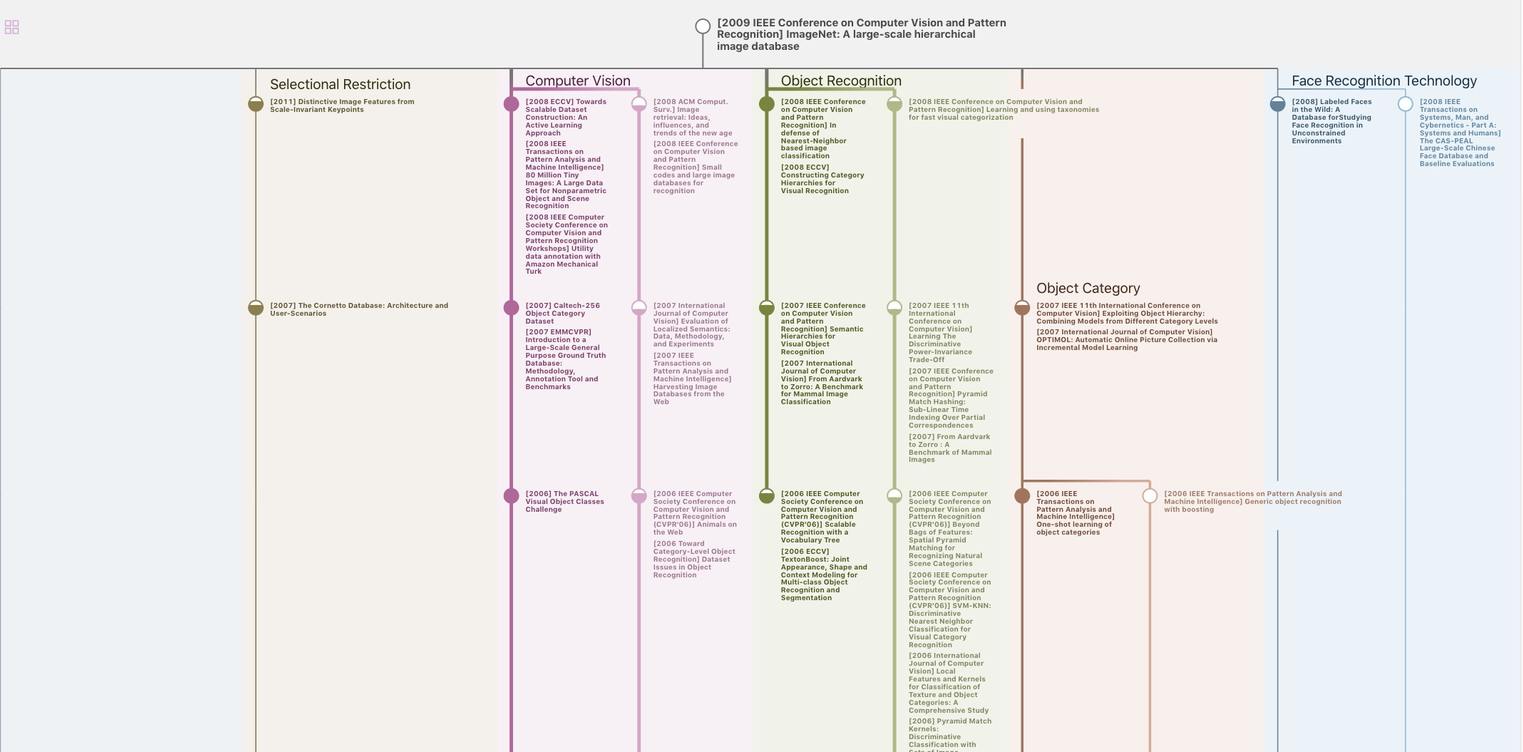
生成溯源树,研究论文发展脉络
Chat Paper
正在生成论文摘要