Explainable Ensemble Machine Learning for Breast Cancer Diagnosis Based on Ultrasound Image Texture Features
FORECASTING(2022)
摘要
Image classification is widely used to build predictive models for breast cancer diagnosis. Most existing approaches overwhelmingly rely on deep convolutional networks to build such diagnosis pipelines. These model architectures, although remarkable in performance, are black-box systems that provide minimal insight into the inner logic behind their predictions. This is a major drawback as the explainability of prediction is vital for applications such as cancer diagnosis. In this paper, we address this issue by proposing an explainable machine learning pipeline for breast cancer diagnosis based on ultrasound images. We extract first- and second-order texture features of the ultrasound images and use them to build a probabilistic ensemble of decision tree classifiers. Each decision tree learns to classify the input ultrasound image by learning a set of robust decision thresholds for texture features of the image. The decision path of the model predictions can then be interpreted by decomposing the learned decision trees. Our results show that our proposed framework achieves high predictive performance while being explainable.
更多查看译文
关键词
ultrasound image texture analysis, breast cancer prediction, explainable machine learning, ensemble classification, decision tree classification
AI 理解论文
溯源树
样例
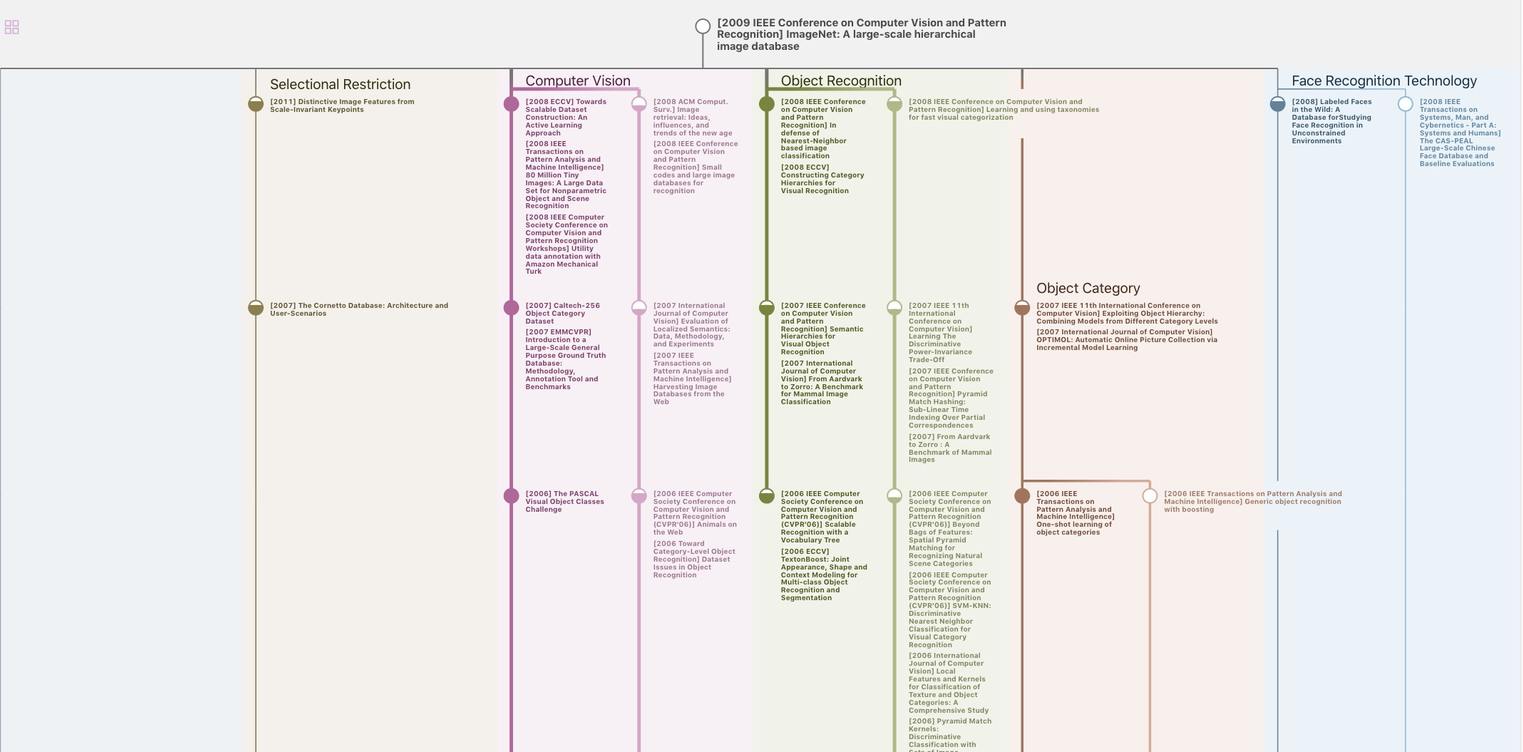
生成溯源树,研究论文发展脉络
Chat Paper
正在生成论文摘要