An Overview of Uncertainty Quantification Methods for Infinite Neural Networks
arxiv(2022)
摘要
To better understand the theoretical behavior of large neural networks, several works have analyzed the case where a network's width tends to infinity. In this regime, the effect of random initialization and the process of training a neural network can be formally expressed with analytical tools like Gaussian processes and neural tangent kernels. In this paper, we review methods for quantifying uncertainty in such infinite-width neural networks and compare their relationship to Gaussian processes in the Bayesian inference framework. We make use of several equivalence results along the way to obtain exact closed-form solutions for predictive uncertainty.
更多查看译文
关键词
uncertainty quantification methods,infinite neural
AI 理解论文
溯源树
样例
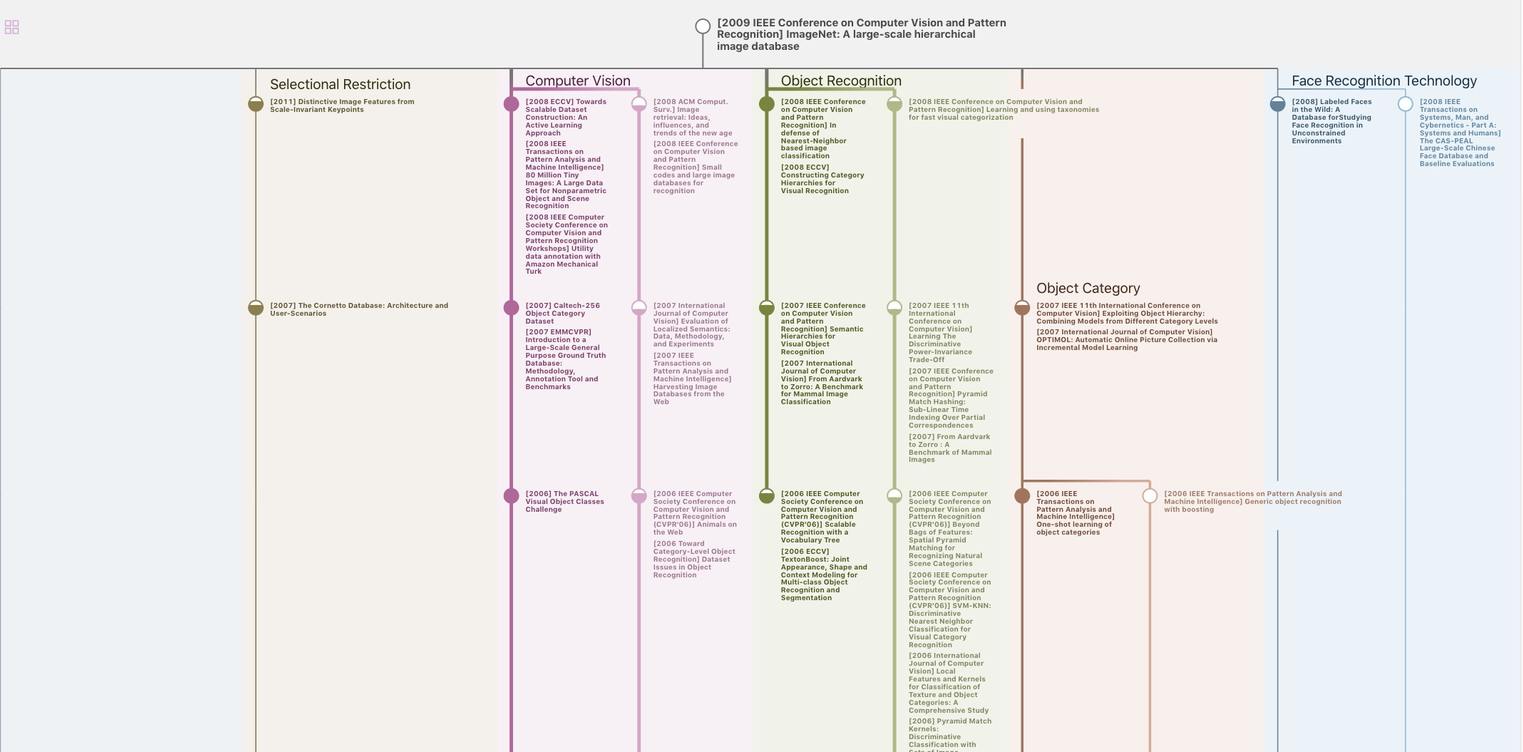
生成溯源树,研究论文发展脉络
Chat Paper
正在生成论文摘要